ImageCLEF 2021 Best of Labs: The Curious Case of Caption Generation for Medical Images
EXPERIMENTAL IR MEETS MULTILINGUALITY, MULTIMODALITY, AND INTERACTION (CLEF 2022)(2022)
摘要
As part of Best of Labs, we have been invited to conduct further investigation on the ImageCLEFmed Caption task of 2021. The task required participants to automatically compose coherent captions for a set of medical images. The most popular means of doing this is with an encoder-to-decoder model. In this work, we investigate a set of choices with regards to aspects of an encoder-to-decoder model. Such choices include what pre-training data should be used, what architecture should be used for the encoder, whether a natural language understanding (e.g., BERT) or generation (e.g., GPT2) checkpoint should be used to initialise the parameters of the decoder, and what formatting should be applied to the ground truth captions during training. For each of these choices, we first made assumptions about what should be used for each choice and why. Our empirical evaluation then either proved or disproved these assumptions with the aim to inform others in the field. Our most important finding was that the formatting applied to the ground truth captions of the training set had the greatest impact on the scores of the task's official metric. In addition, we discuss a number of inconsistencies in the results that others may experience when developing a medical image captioning system.
更多查看译文
关键词
Medical image captioning, Encoder-to-decoder, Multi-modal, Warm-starting
AI 理解论文
溯源树
样例
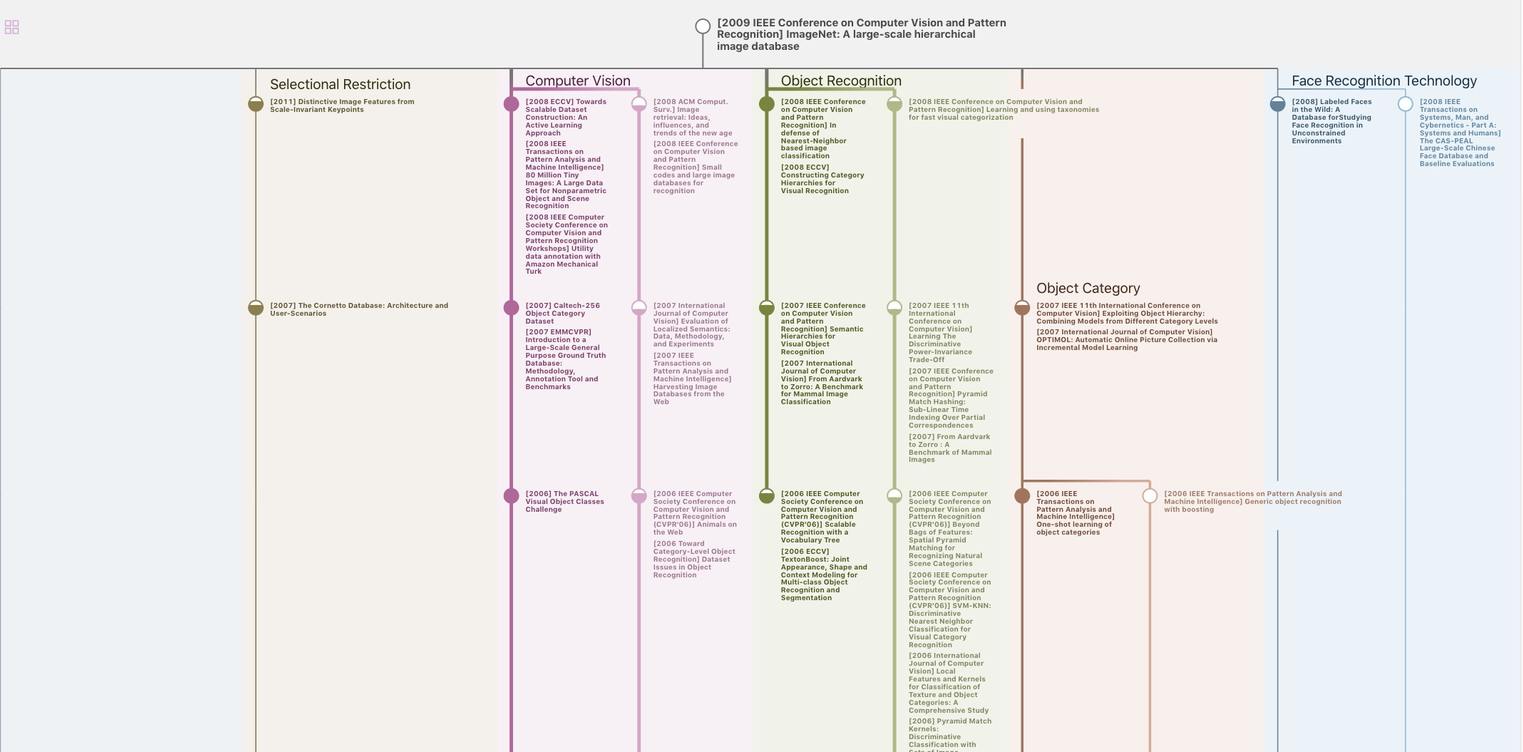
生成溯源树,研究论文发展脉络
Chat Paper
正在生成论文摘要