Tier-Based Optimization for Synthesized Network Intrusion Detection System
IEEE ACCESS(2022)
摘要
The innovation and evolution of hacking methodologies have led to a sharp rise in cyber attacks, highlighting the need for enhanced network security approaches. Network intrusion detection systems based on machine learning are playing a significant role in the domain of network security. However, designing an optimal framework for a network intrusion detection system is an ongoing concern. In this study, an optimal framework for a network intrusion detection system based on image processing is proposed. The framework is a fusion of augmented feature selection flow with an image transformation and enhancement methodology. Initially, the proposed framework reduces the number of features to achieve overall efficiency. Later, the non-image data is transformed into images. The transformed images are then enhanced for achieving effective anomaly detection based on a deep-learning classifier. The proposed method is implemented on three diverse benchmark datasets of intrusion detection. To illustrate the efficiency of the proposed framework it is compared with some of the most recent publications on image-processing-based network intrusion detection systems.
更多查看译文
关键词
Feature extraction, Telecommunication traffic, Image processing, Network intrusion detection, Matrix converters, Convolutional neural networks, Intrusion detection, Computer hacking, Cyber attack, CNN, CSE-CIC-IDS 2018, CIC-IDS 2017, ISCX-IDS 2012, intrusion detection, network intrusion detection system
AI 理解论文
溯源树
样例
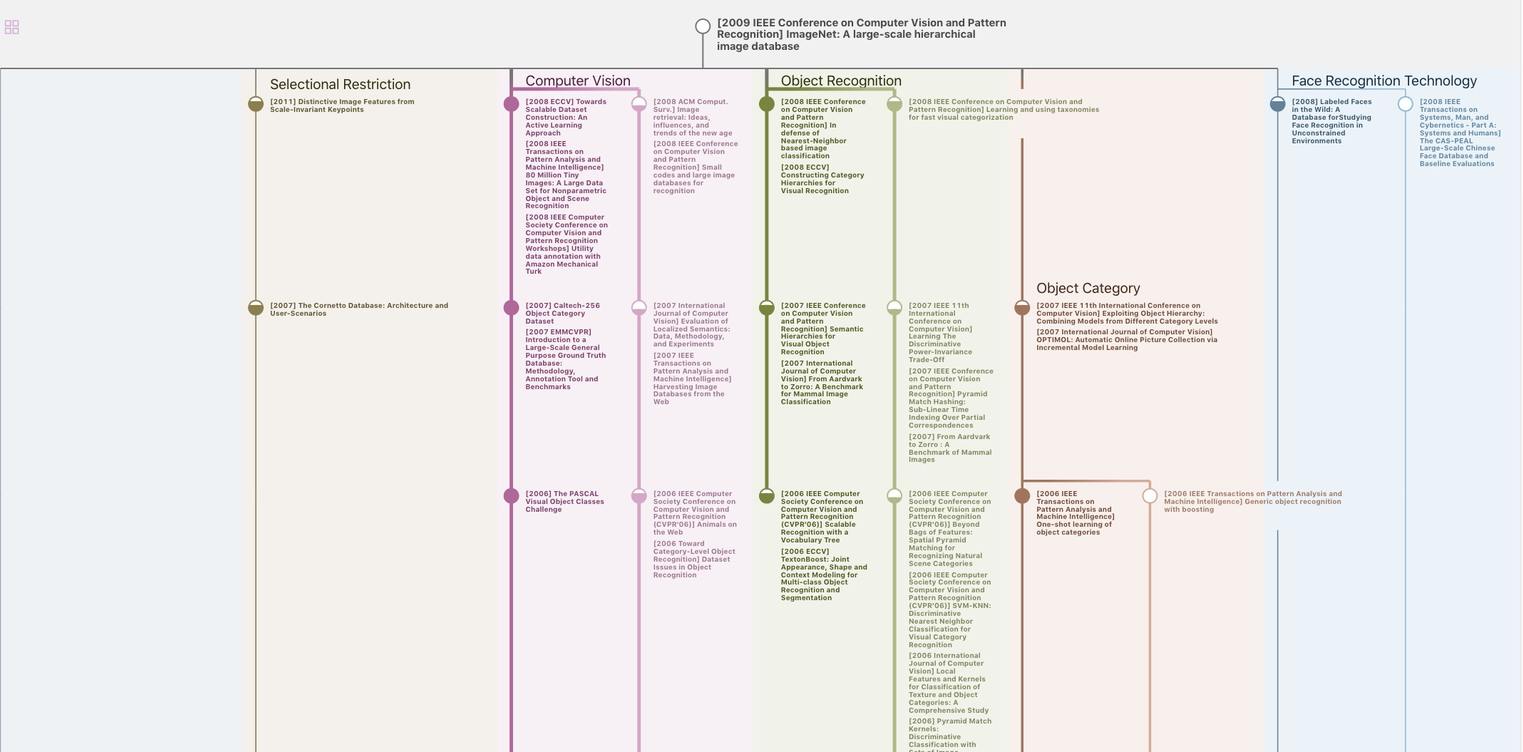
生成溯源树,研究论文发展脉络
Chat Paper
正在生成论文摘要