Deep Learning Model Development for Detecting Coffee Tree Changes Based on Sentinel-2 Imagery in Vietnam
IEEE ACCESS(2022)
摘要
Scientists and land managers have spent considerable time and resources monitoring coffee forests in the great basalt plateau. Deep learning models for coffee classification using remote sensing data have developed into a tool that may eventually replace manual image interpretation. This study proposes a U-Net model for classifying coffee planting regions using Sentinel-2 data, which aid in the annual monitoring of coffee plantation area changes. Numerous optimizer methods were evaluated and compared to support-vector-machine and random-forest methods. Twelve U-Net models were trained and compared in total. The trained deep learning models outperformed the two benchmark methods. As a result, the U-Net model with the Adadelta optimizer and 128 x 128 x 4 input data size was chosen due to its near-95 percent accuracy and 0.12 loss function value. The model was used to successfully detect location of the Vietnamese coffee ecosystem. The Net-Adadelta-128 model's output is consistent with data from statistical reports, which estimated the area of the coffee land cover to be 684, 681, and 676 thousand hectares in 2019, 2020, and 2021, respectively. The best U-Net model, which takes approximately 30 minutes to create a new classification for 55,000 square kilometres, may one day be used for coffee research and management.
更多查看译文
关键词
Deep learning,coffee,U-Net,loss function,optimization
AI 理解论文
溯源树
样例
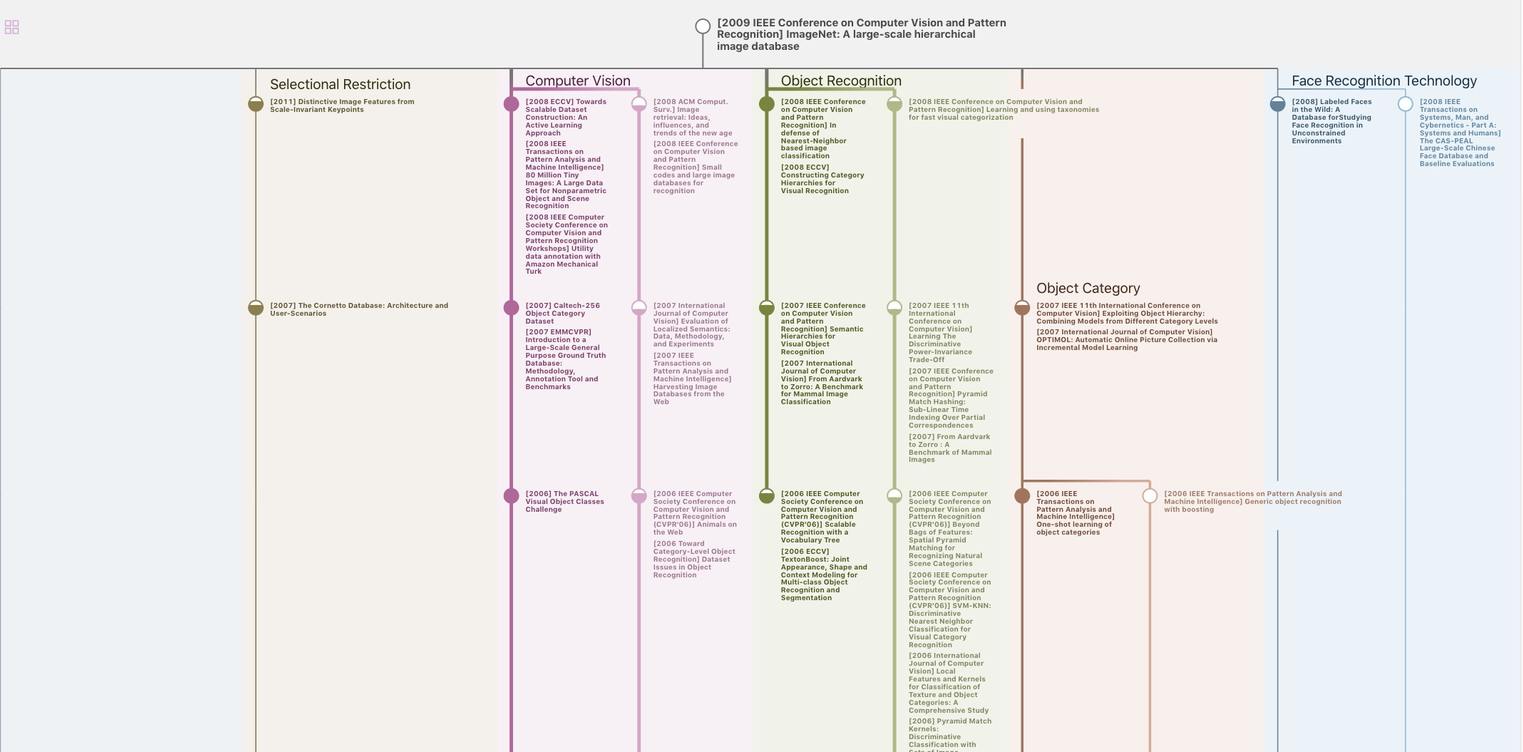
生成溯源树,研究论文发展脉络
Chat Paper
正在生成论文摘要