Task Offloading and Resource Allocation for Industrial Internet of Things: A Double-Dueling Deep Q-Network Approach
IEEE ACCESS(2022)
摘要
With the development of 5G technology, Mobile Edge Computing (MEC) has become a promising technology that is widely used in the Industrial Internet of Things (IIoT) and other fields. However, the increase in terminal devices and massive data growth has brought new challenges to MEC systems. How to meet the latency requirements of mobile devices while reducing system costs as much as possible is an urgent problem to be solved. To address this problem, we construct a smart factory model and formulate a mixed-integer nonlinear programming problem with the goal of minimizing the weighted sum of task delay and energy consumption. Considering that this problem is non-deterministic polynomial hard (NP-hard), we choose the Deep Q-network (DQN) approach to solve the objective function. In order to avoid the inaccurate Q-value estimation problem of the Double DQN algorithm and the overestimation problem of the Dueling DQN algorithm, we combine them to propose a Double-Dueling DQN (D3QN) algorithm. The simulation results show that the D3QN algorithm significantly outperforms the DQN, the Double DQN, and the Dueling DQN algorithm in reducing the total system cost.
更多查看译文
关键词
Deep learning, Servers, Industrial Internet of Things, Resource management, Computational modeling, Energy consumption, Delays, Edge computing, Mobile computing, Mobile edge computing (MEC), task offloading, deep reinforcement learning, Industrial Internet of Things (IIoT)
AI 理解论文
溯源树
样例
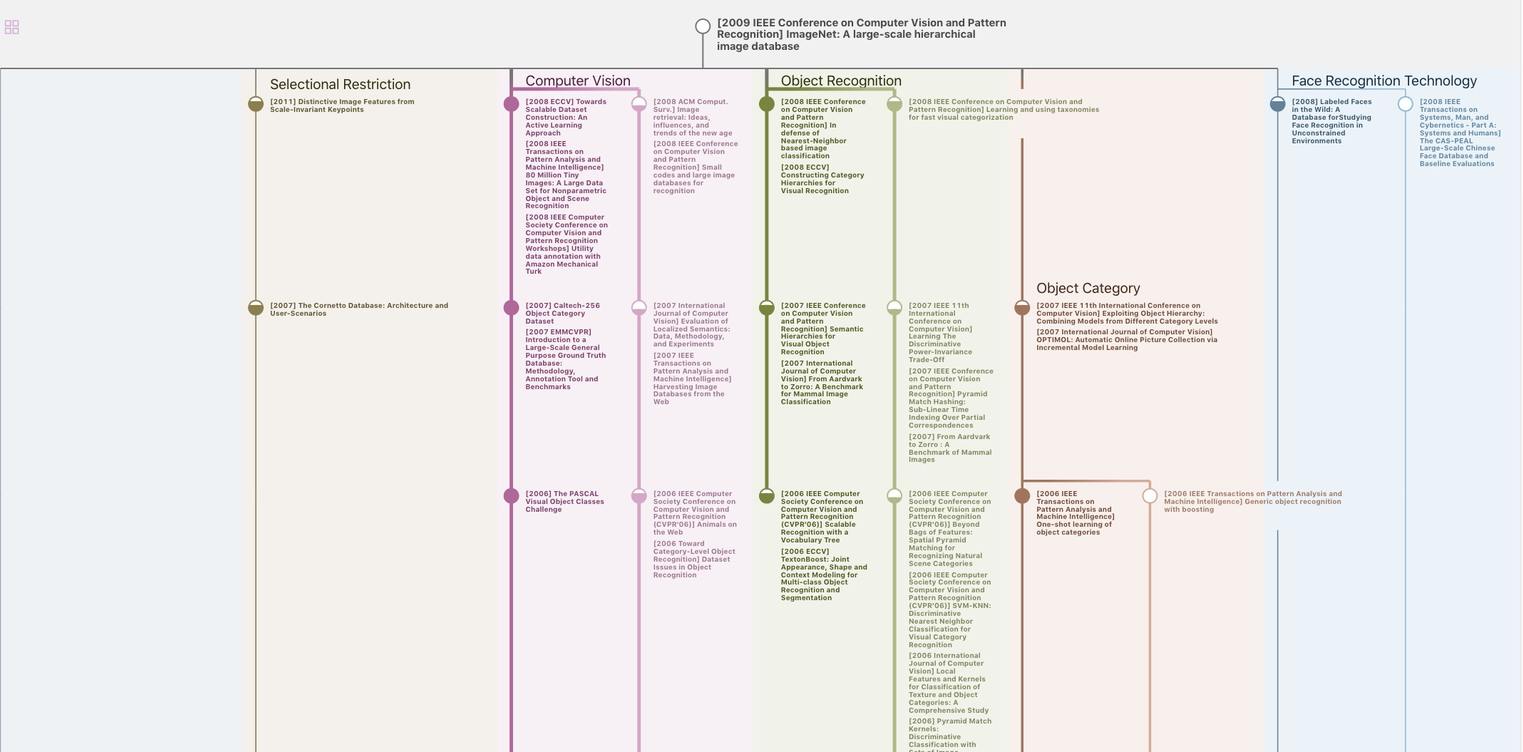
生成溯源树,研究论文发展脉络
Chat Paper
正在生成论文摘要