Enhancing Cluster Analysis With Explainable AI and Multidimensional Cluster Prototypes
IEEE ACCESS(2022)
摘要
Explainable Artificial Intelligence (XAI) aims to introduce transparency and intelligibility into the decision-making process of AI systems. Most often, its application concentrates on supervised machine learning problems such as classification and regression. Nevertheless, in the case of unsupervised algorithms like clustering, XAI can also bring satisfactory results. In most cases, such application is based on the transformation of an unsupervised clustering task into a supervised one and providing generalised global explanations or local explanations based on cluster centroids. However, in many cases, the global explanations are too coarse, while the centroid-based local explanations lose information about cluster shape and distribution. In this paper, we present a novel approach called ClAMP (Cluster Analysis with Multidimensional Prototypes) that aids experts in cluster analysis with human-readable rule-based explanations. The developed state-of-the-art explanation mechanism is based on cluster prototypes represented by multidimensional bounding boxes. This allows representing of arbitrary shaped clusters and combines the strengths of local explanations with the generality of global ones. We demonstrate and evaluate the use of our approach in a real-life industrial case study from the domain of steel manufacturing as well as on the benchmark datasets. The explanations generated with ClAMP were more precise than either centroid-based or global ones.
更多查看译文
关键词
Prototypes, Clustering algorithms, Artificial intelligence, Task analysis, Data mining, Machine learning algorithms, Feature extraction, Knowledge engineering, Data mining, clustering, explainable AI, expert's knowledge
AI 理解论文
溯源树
样例
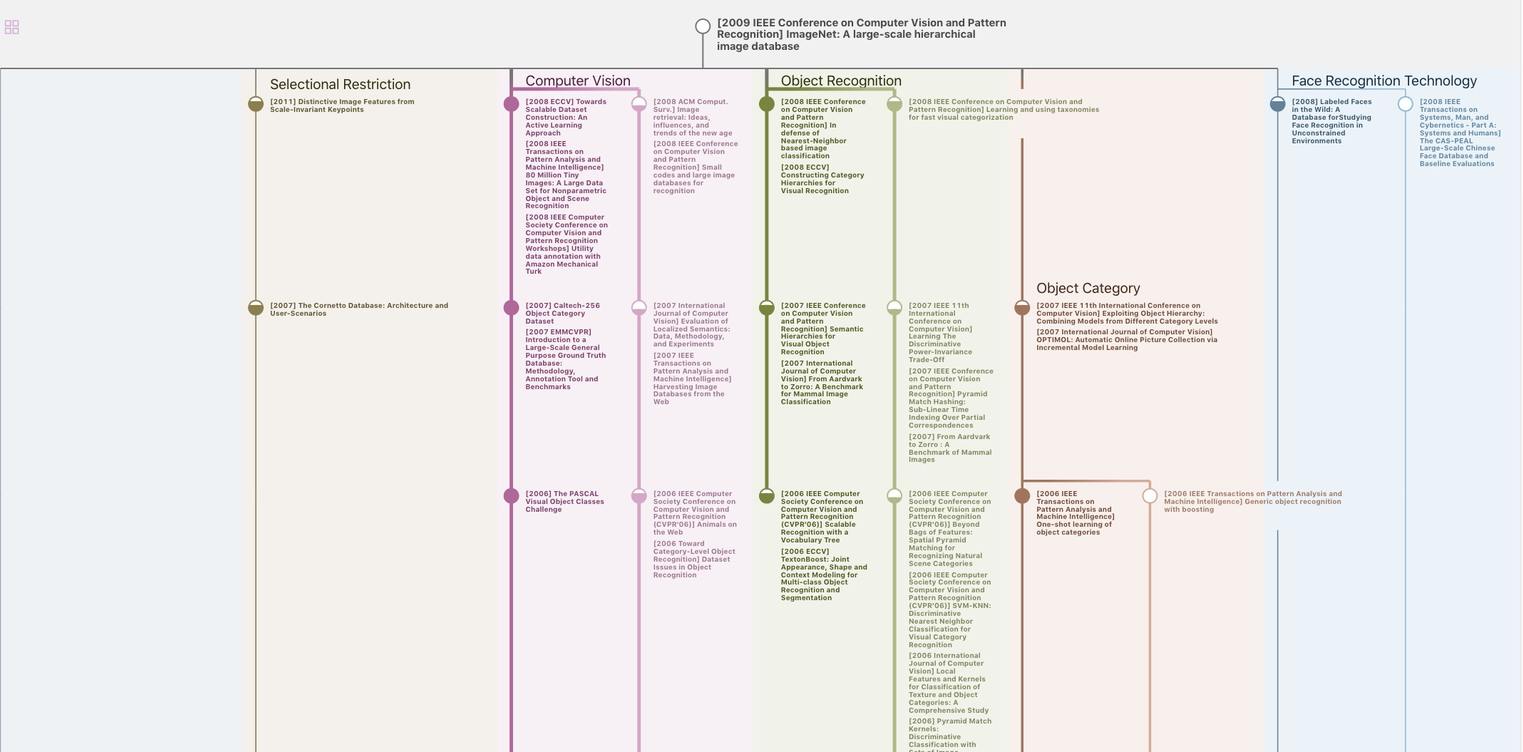
生成溯源树,研究论文发展脉络
Chat Paper
正在生成论文摘要