A Full Tensor Network for Hyperspectral Image Classification
IEEE GEOSCIENCE AND REMOTE SENSING LETTERS(2022)
Abstract
Hyperspectral image (HSI) is widely used in real-world classification tasks since it contains rich spatial and spectral features consisting of hundreds of continuous bands. However, the rich spectral features always result in "dimension disaster" for HSI classification. Some traditional methods adopt the strategy of dimensionality reduction in advance to alleviate the influences of high spectral feature dimension, which usually results in degraded classification accuracy due to the hard coupling of the dimensionality reduction model and the classifier. In this letter, we propose a full tensor network (FTN) for HSI classification based on Tucker decomposition-based hidden (TDH) layer and Candecomp/Parafac decomposition-based classification (CPDC) layer. The proposed network can efficiently extract the high-level structural features directly from the original data and classify HSI in a single network without dimension reduction procedure in advance. Moreover, the proposed model has a simple structure and holds fewer parameters than the state-of-the-art classification methods. The experimental results on Pavia University (PU) and Salinas (SA) datasets show that for a training ratio of 5%, the overall accuracy (OA) of our model is averaged 3.41% higher than convolutional neural network (CNN) models while the network parameters are averaged 80.44% less, which validate the merits of the proposed method.
MoreTranslated text
Key words
Tensors, Dimensionality reduction, Neurons, Neural networks, Training, Periodic structures, Matrix decomposition, Full tensor network (FTN), hyperspectral image (HSI) classification, tensor decomposition
AI Read Science
Must-Reading Tree
Example
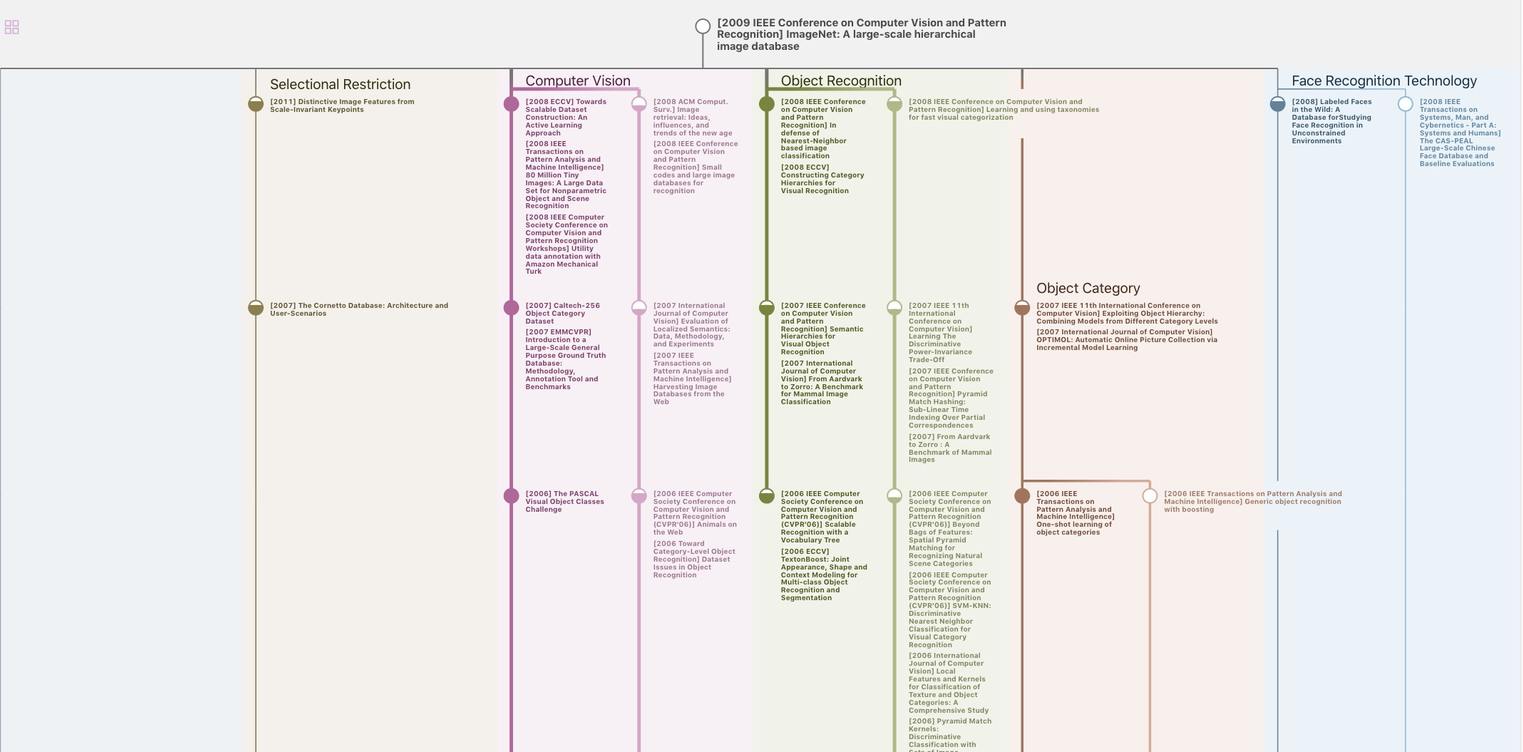
Generate MRT to find the research sequence of this paper
Chat Paper
Summary is being generated by the instructions you defined