k-best feature selection and ranking via stochastic approximation
Expert Systems with Applications(2023)
摘要
This study presents SPFSR, a novel stochastic approximation approach for performing simultaneous k-best feature ranking (FR) and feature selection (FS) based on Simultaneous Perturbation Stochastic Approximation (SPSA) with Barzilai and Borwein (BB) non-monotone gains. SPFSR is a wrapper-based method which may be used in conjunction with any given classifier or regressor with respect to any suitable corresponding performance metric. Numerical experiments are performed on 47 public datasets which contain both classification and regression problems, with the mean accuracy and R2 reported from four different classifiers and four different regressors respectively. In over 80% of classification experiments and over 85% of regression experiments SPFSR provided a statistically significant improvement or equivalent performance compared to existing, well-known FR techniques. Furthermore, SPFSR obtained a better classification accuracy and R-squared on average compared to utilising the entire feature set.
更多查看译文
关键词
Explainable artificial intelligence,Feature selection,Feature ranking,Stochastic approximation,Barzilai and Borwein method
AI 理解论文
溯源树
样例
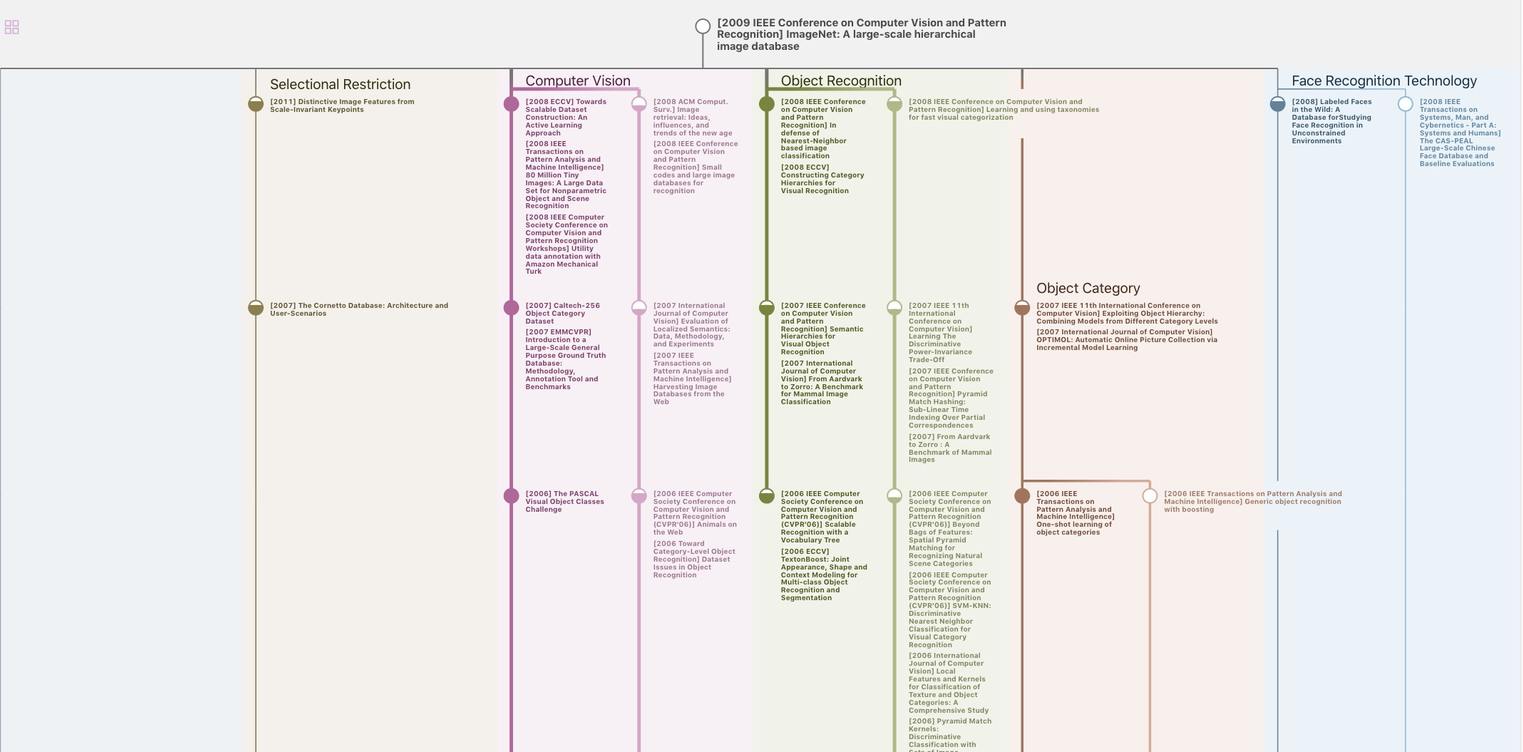
生成溯源树,研究论文发展脉络
Chat Paper
正在生成论文摘要