Unsupervised Domain Adaptation for Crime Risk Prediction Across Cities
IEEE TRANSACTIONS ON COMPUTATIONAL SOCIAL SYSTEMS(2023)
摘要
Crime risk prediction is crucial for city safety and residents’ life quality. However, without labeled data, it is challenging to predict crime risk in cities. Due to municipal regulations and maintenance costs, it is not trivial for many cities to collect high-quality labeled crime data. In particular, some cities have lots of labeled data while others may have few. It has been possible to develop a crime prediction model for a city without labeled crime data by learning knowledge from a city with abundant data. Nevertheless, the inconsistency of relevant context data between cities exacerbates the difficulty of this prediction task. To this end, this article proposes an effective unsupervised domain adaptation model (UDAC) for crime risk prediction across cities while addressing the contexts’ inconsistency issue. More specifically, we first identify several similar source city grids for each target city grid. Based on these source city grids, we then construct auxiliary contexts for the target city, to make contexts consistent between the two cities. A dense convolutional network with unsupervised domain adaptation is designed to learn high-level representations for accurate crime risk prediction and simultaneously learn domain-invariant features for domain adaptation. The effectiveness of our model is verified through extensive experiments using three real-world datasets.
更多查看译文
关键词
Urban areas, Predictive models, Data models, Adaptation models, Task analysis, Public transportation, Transfer learning, Crime prediction, crime risk, unsupervised domain adaptation
AI 理解论文
溯源树
样例
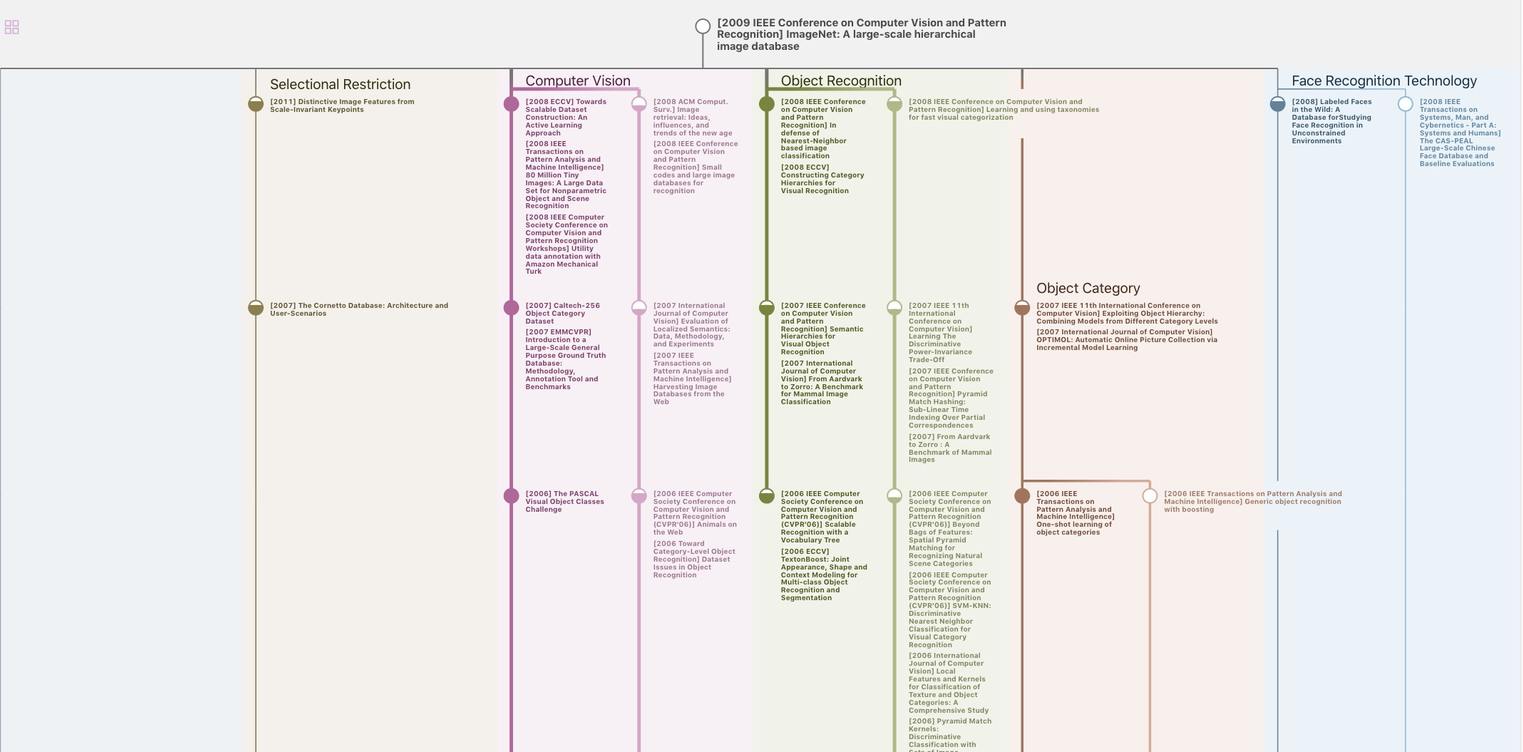
生成溯源树,研究论文发展脉络
Chat Paper
正在生成论文摘要