Data-Driven Precoder Codebook Design for SU-MIMO Systems
2022 IEEE 95TH VEHICULAR TECHNOLOGY CONFERENCE (VTC2022-SPRING)(2022)
摘要
Conventional MIMO precoding relying on a predefined codebook exhibits significantly poor performance in terms of bit error ratio (BER). This is because traditionally codebooks are designed relying on the simplifying assumption of having fixed channel probability distribution. However, owing to both hardware imperfections arising due to non-linear components and channel estimation imperfections, as well as channel aging, the fixed channel probability assumption is unrealizable in practical MIMO systems. Therefore, in this paper, we first propose a data-driven minimum BER (MBER) based precoder using deep reinforcement learning (DRL) without relying on channel model distribution. Then to facilitate the finite-rate MIMO feedback systems, we propose a data-driven user-specific codebook design for downlink users (UE), where UE populates MBER precoders and constructs the codebook by invoking an unsupervised clustering based quantizer. We demonstrate by simulations that our proposed precoder selection relying on data-driven codebook performs similarly to that of genie-aided channel state information (CSI), whilst our data-driven precoder design outperforms the conventional MIMO precoding by more than 5 dB for BER of 10(-3) with CSI error variance of 0.02.
更多查看译文
关键词
channel estimation imperfections,channel aging,fixed channel probability assumption,practical MIMO systems,data-driven minimum BER based precoder,deep reinforcement learning,channel model distribution,finite-rate MIMO feedback systems,data-driven user-specific codebook design,unsupervised clustering based quantizer,data-driven codebook performs,genie-aided channel state information,data-driven precoder codebook design,predefined codebook,bit error ratio,channel probability distribution,hardware imperfections,nonlinear components,SU-MIMO precoding systems,UE,MBER precoders,DRL
AI 理解论文
溯源树
样例
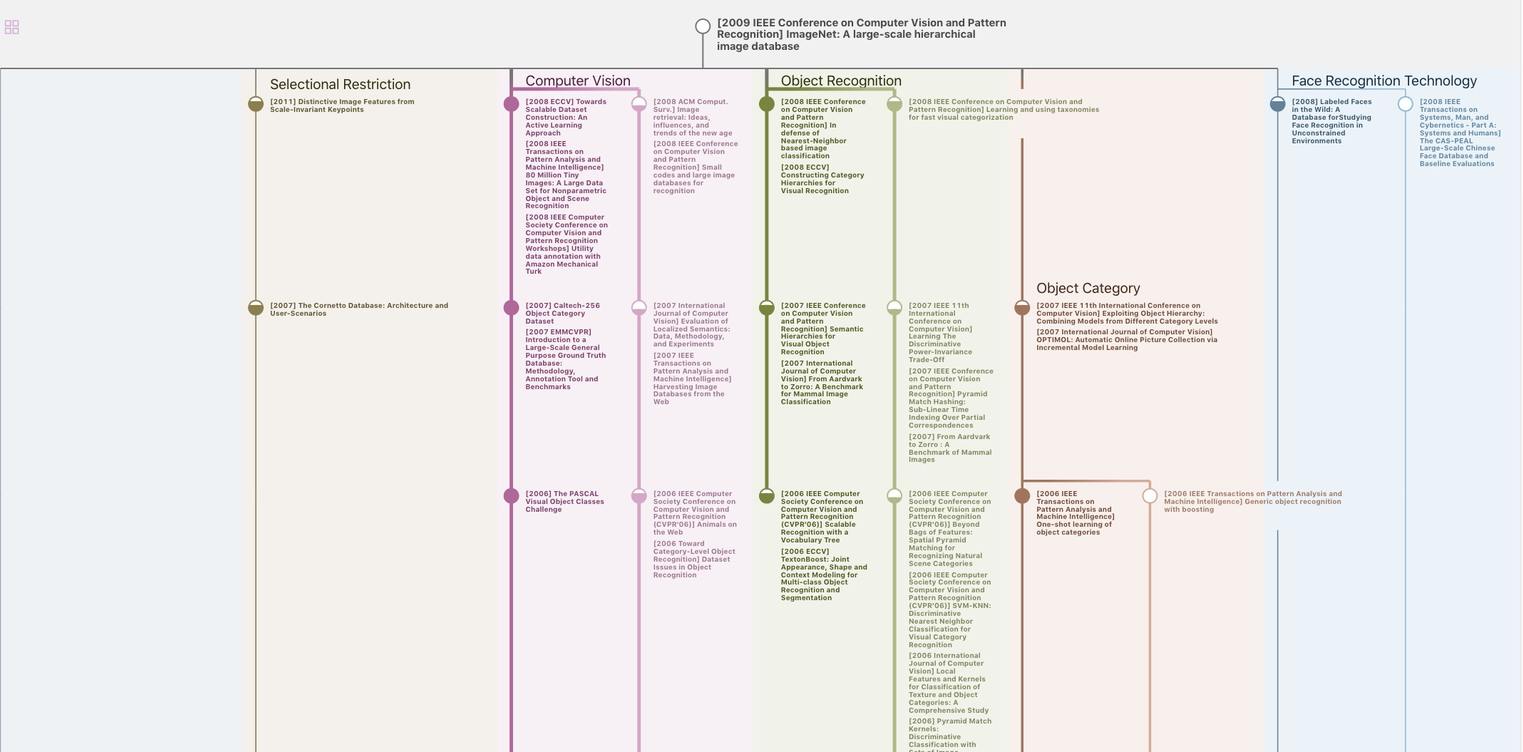
生成溯源树,研究论文发展脉络
Chat Paper
正在生成论文摘要