Prediction of shear stress induced by shoaling internal solitary waves based on machine learning method
MARINE GEORESOURCES & GEOTECHNOLOGY(2023)
摘要
Recently, the interactions between internal solitary waves (ISWs) and the seabed have directed increasing attention to ocean engineering and offshore energy. In particular, ISWs induce bottom currents and pressure fluctuations in deep water. In this paper, we propose a method for predicting the shear stress induced by shoaling ISWs based on machine learning, and the developed approach can be used to quickly determine the safety and stability of ocean engineering. First, we provided a basic dataset for model training. Four machine learning models were selected to predict the shear stress induced by shoaling ISWs under different trim conditions. The results indicated that the performance of the convolutional neural network-long short-term memory (CNN-LSTM) forest prediction model was significantly better than the three other tested models, including long short-term memory (LSTM), support vector regression (SVR) and deep neural network (DNN) models. Therefore, the CNN-LSTM forest prediction model was the optimal model for predicting the shear stress induced by shoaling ISWs. Specifically, each metric of the CNN-LSTM model was smaller than that of the other three, and the root mean squared error to the standard deviation ratio was closest to 0.7. In addition, the CNN-LSTM model significantly outperformed the SVR and DNN models in terms of the length of prediction time. The predicted values by the CNN-LSTM model were consistent with the experimental values. The method for predicting shear stress based on machine learning in this paper can be used to predict the shear stress induced by shoaling ISWs, guide future field experiment designs, reduce damage to the seabed caused by ISWs, and promote the development of ocean engineering in deep water.
更多查看译文
关键词
Shear stress,prediction,internal solitary waves,machine learning,sediment
AI 理解论文
溯源树
样例
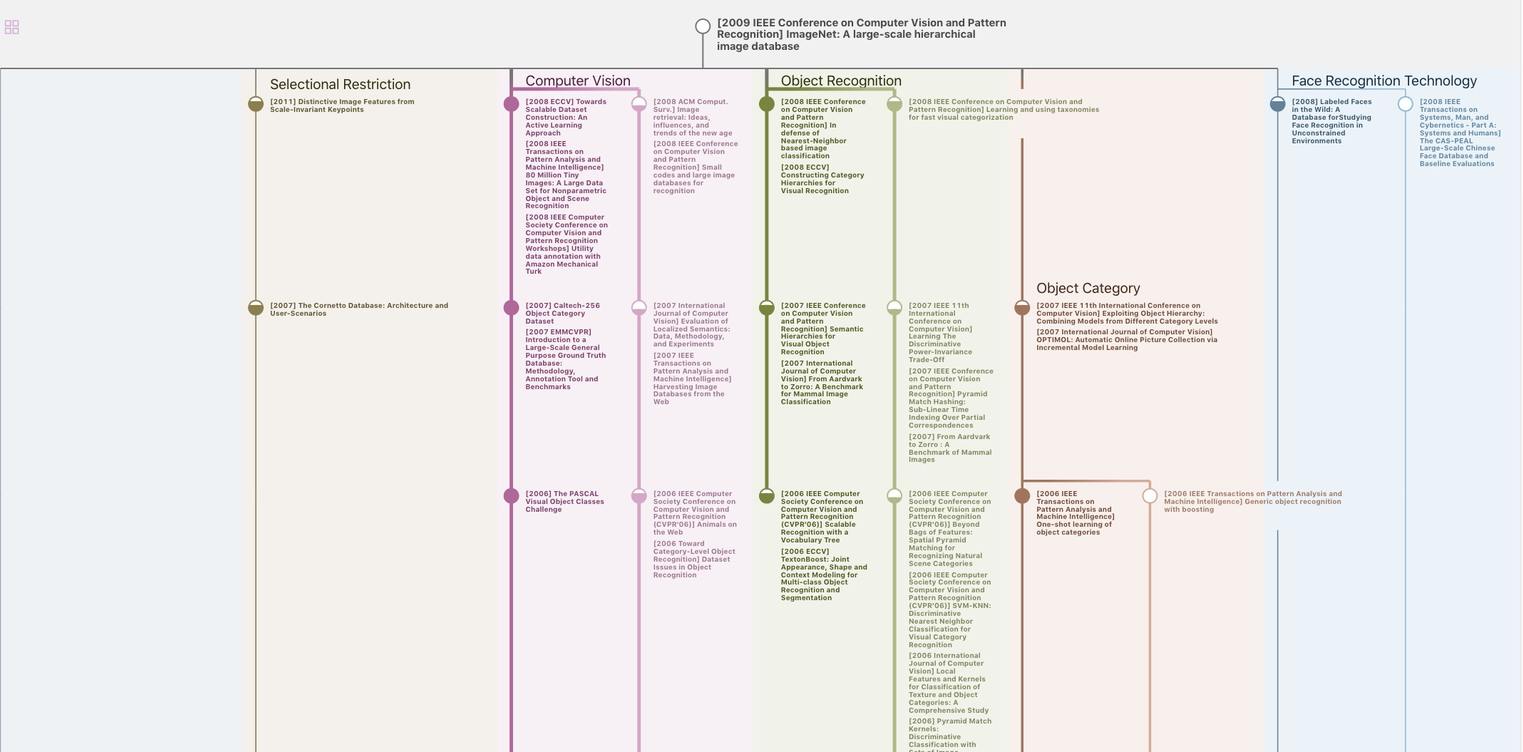
生成溯源树,研究论文发展脉络
Chat Paper
正在生成论文摘要