A Transfer Learning-Based Method to Detect Insulator Faults of High-Voltage Transmission Lines via Aerial Images: Distinguishing Intact and Broken Insulator Images
IEEE Systems, Man, and Cybernetics Magazine(2022)
Abstract
Deep learning methods have shown great promise in high-voltage transmission lines’ (TLs’) intelligent inspections. The expansion of power systems, including TLs, has brought the problem of insulator fault detection into account more than before. In this article, a novel transfer learning framework based on a pretrained VGG-19 deep convolutional neural network (CNN) is proposed to detect “missing faults” (broken insulators) in aerial images. In this procedure, a well-known large imagery dataset called
ImageNet
is used to train VGG-19, and then the knowledge of this deep CNN is transferred. By using a few layers for a fine-tuning purpose, the newly built deep CNN is capable of distinguishing the corrupted and intact insulators. This method is able to diagnose these faults using the aerial images taken from TLs in different environments. The original dataset used in this article is the Chinese Power Line Insulator Dataset (CPLID), which is an imbalanced dataset and includes only 3,808 insulator images. Therefore, a random image-augmentation procedure is proposed and applied to generate a more suitable dataset with 16,720 images. This new dataset allows us to offer higher detection accuracy than the original one because it is a balanced dataset. Training a deep CNN by using it gives more power to the system for detecting the corrupted insulators in different situations such as rotated, dark, and blurry images with complex backgrounds. The comparison results of this study show the advantages of the proposed method over various existing ones.
MoreTranslated text
AI Read Science
Must-Reading Tree
Example
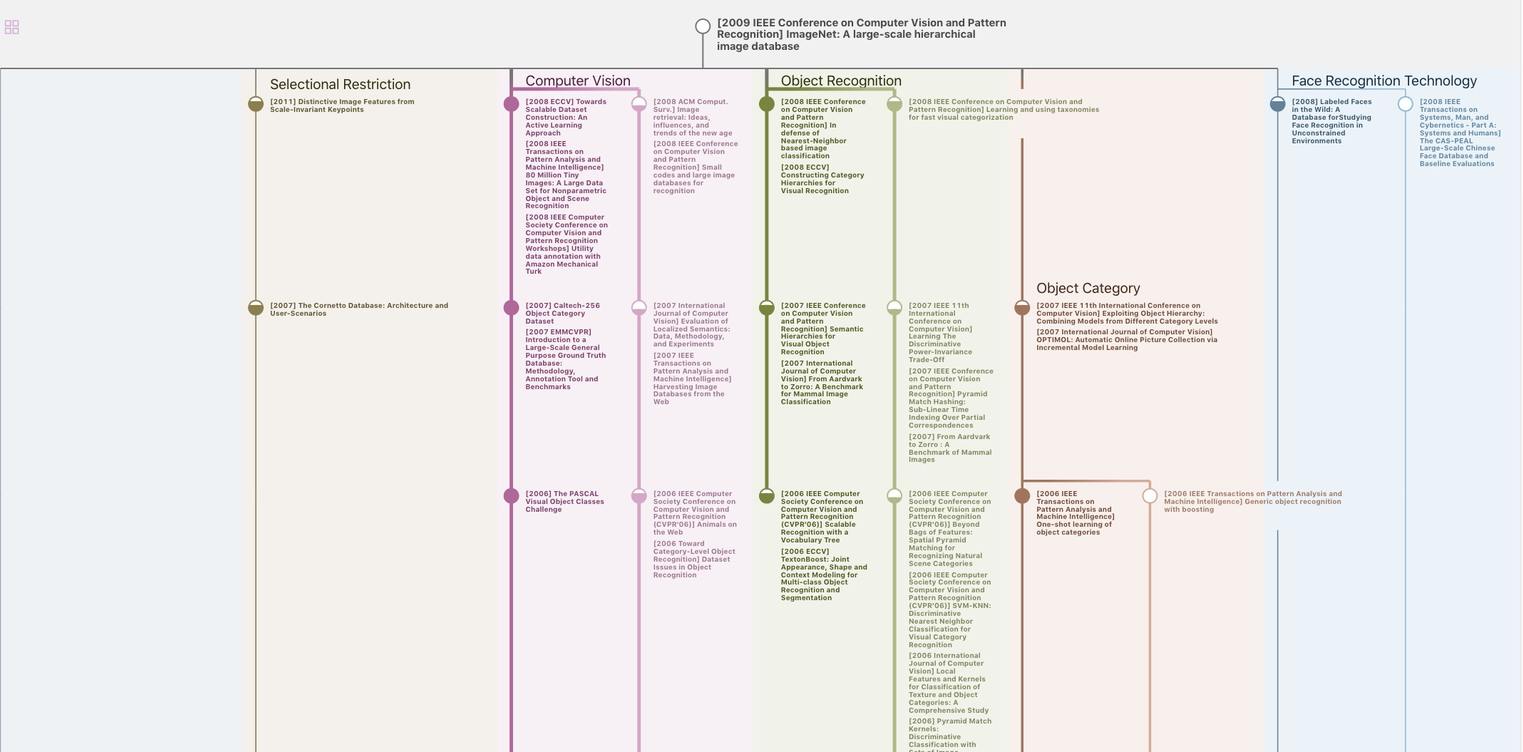
Generate MRT to find the research sequence of this paper
Chat Paper
Summary is being generated by the instructions you defined