Parameter-Free Latent Space Transformer for Zero-Shot Bidirectional Cross-modality Liver Segmentation
MEDICAL IMAGE COMPUTING AND COMPUTER ASSISTED INTERVENTION, MICCAI 2022, PT IV(2022)
Abstract
In this paper, we address the domain shift in cross CT-MR liver segmentation task with a latent space investigation. Domain adaptation between modalities is of significant importance in clinical practice, as different diagnostic procedures require different imaging modalities, such as CT and MR. Thus, training a convolutional neural network (CNN) with one modality may not be sufficient for application in another one. Most domain adaptation methods need to use data and ground truths of both source and target domain in the training process. Different from these techniques, we propose a zero-shot bidirectional cross-modality liver segmentation method by investigating a parameter-free latent space through the prior knowledge from CT and MR images. Experiments on the CHAOS, the subset of LiTS and the local TACE datasets demonstrate that our method can well deal with the problem of CNN failure caused by domain shift and yields promising segmentation results.
MoreTranslated text
Key words
Cross-modality liver segmentation, Latent space transformer, Parameter-free, Zero-shot
AI Read Science
Must-Reading Tree
Example
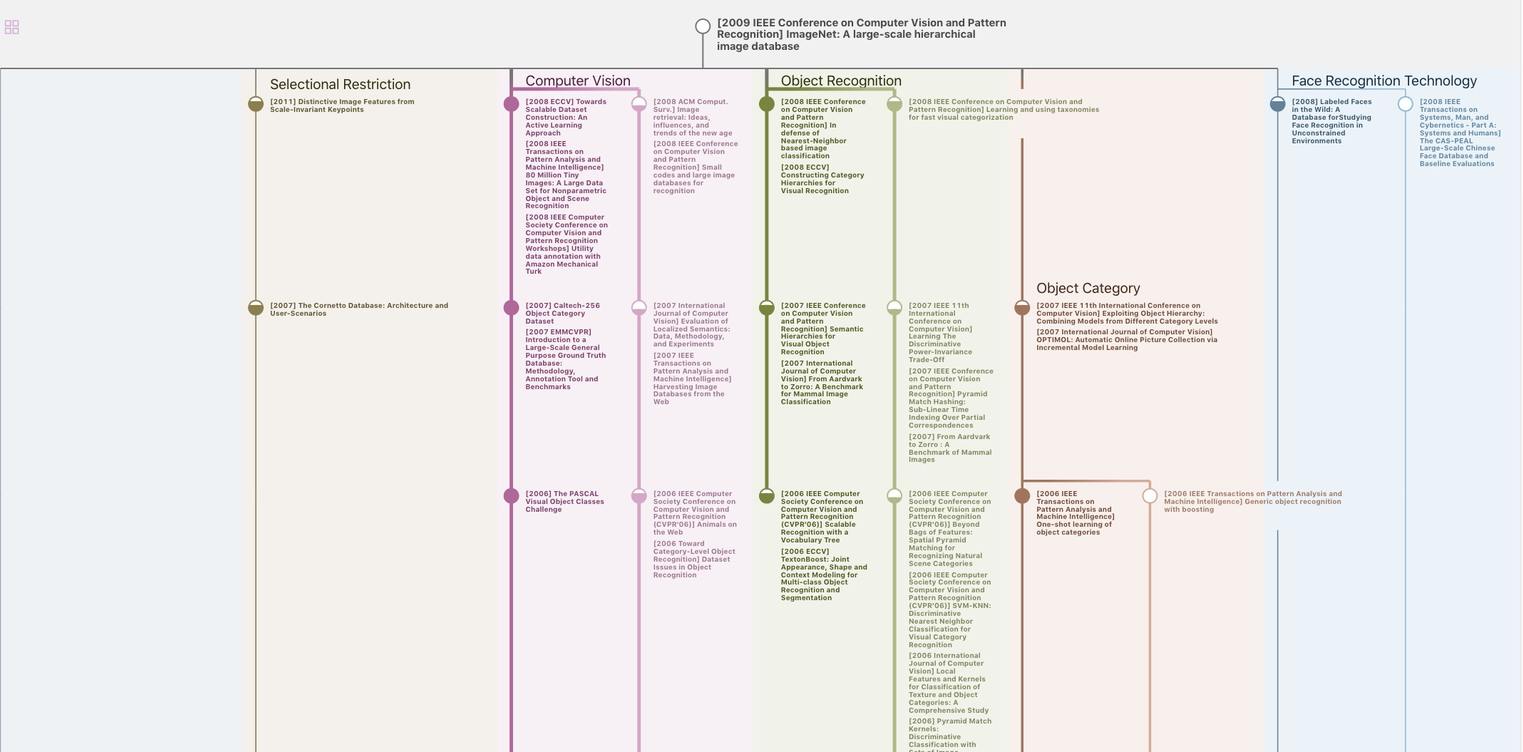
Generate MRT to find the research sequence of this paper
Chat Paper
Summary is being generated by the instructions you defined