Composite quantile regression for heteroscedastic partially linear varying-coefficient models with missing censoring indicators
JOURNAL OF STATISTICAL COMPUTATION AND SIMULATION(2023)
Abstract
This paper is concerned with composite quantile regression for partially linear varying-coefficient models with heteroscedasticity when data are right censored and censoring indicators are missing at random. We construct estimators of parametric regression coefficients and nonparametric varying-coefficient functions in the proposed models based on regression calibration, imputation and inverse probability weighted approaches. The asymptotic normality of the proposed estimators is proved. Meanwhile, an adaptive LASSO penalized variable selection method and its oracle property are considered. We also demonstrate the performance of the proposed estimation method and variable selection procedure through comprehensive simulations and a real-data application.
MoreTranslated text
Key words
Adaptive LASSO penalty, censoring indicators, missing at random, oracle property, partially linear varying-coefficient model
AI Read Science
Must-Reading Tree
Example
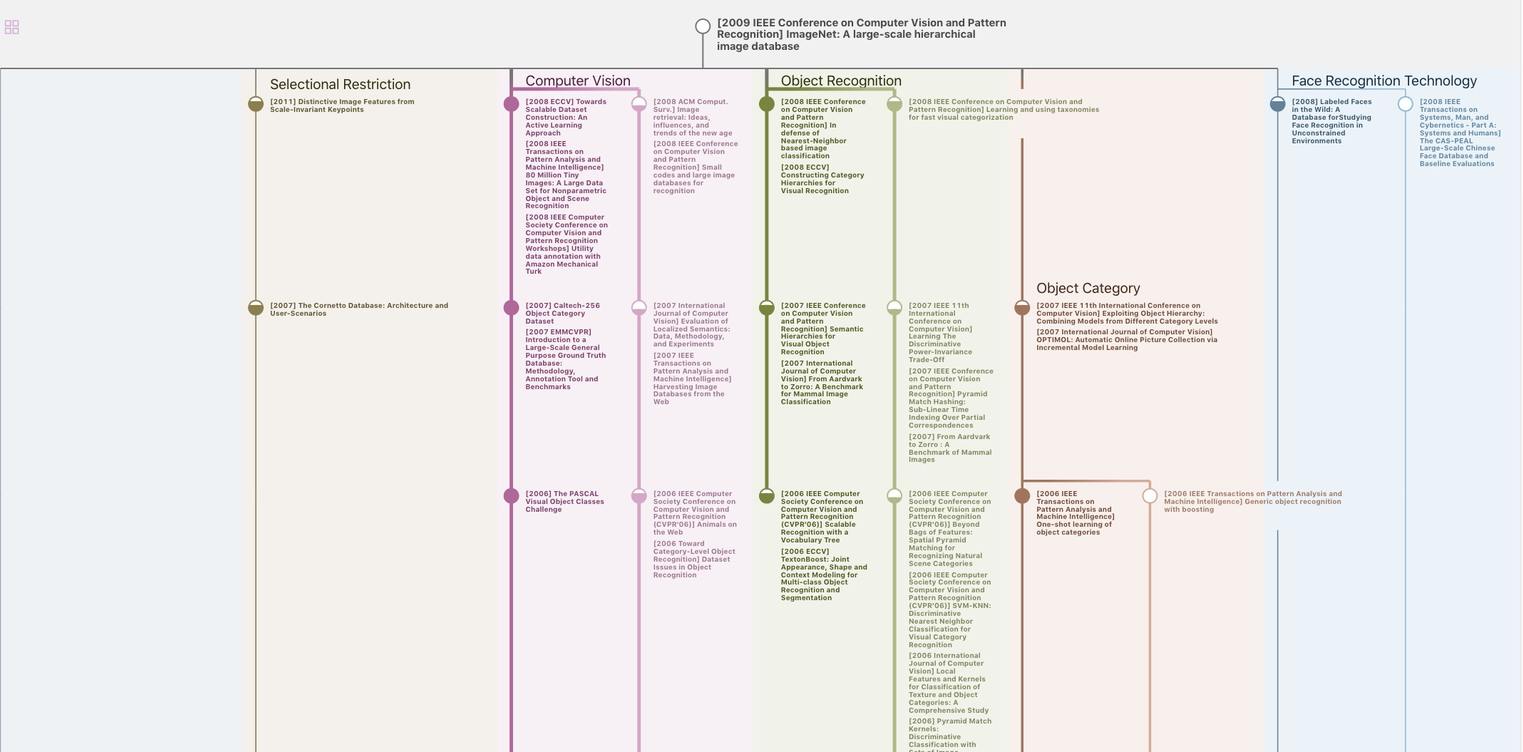
Generate MRT to find the research sequence of this paper
Chat Paper
Summary is being generated by the instructions you defined