Soft hypergraph regularized weighted low rank subspace clustering for hyperspectral image band selection
INTERNATIONAL JOURNAL OF REMOTE SENSING(2022)
摘要
Recently, the graph regularized low-rank representation (GLRR) has been introduced in Hyperspectral Image (HSI) to explore global structures information by exploring the lowest-rank representation of all the data jointly and the local geometrical structure by the graph regularization. However, the traditional graph models are mostly based on a simple intrinsic structure. In this paper, to represent the complex intrinsic band information and further enhance the low rank of the matrix, we propose a soft hypergraph regularized weighted low-rank subspace clustering (HGWLRSC) method for HSI band selection. On the one hand, considering the complex correlation between adjacent bands, hypergraph technique is introduced, which take advantage of the band similarity properties to extract more valuable information and reveals the intrinsic multiple relationships of HSI band sets. On the other hand, the weighted low-rank subspace clustering model is introduced to not only capture the global structure information for the learned representation coefficient matrix but also to consider the importance of different rank components. The proposed algorithm was tested on three widely used hyperspectral data sets, and the experimental results indicate that the proposed HGWLRSC algorithm outperforms the other state-of-the art methods and achieves a very competitive band selection performance for HSI.
更多查看译文
关键词
Band selection, hyperspectral image, hypergraph, low rank subspace clustering
AI 理解论文
溯源树
样例
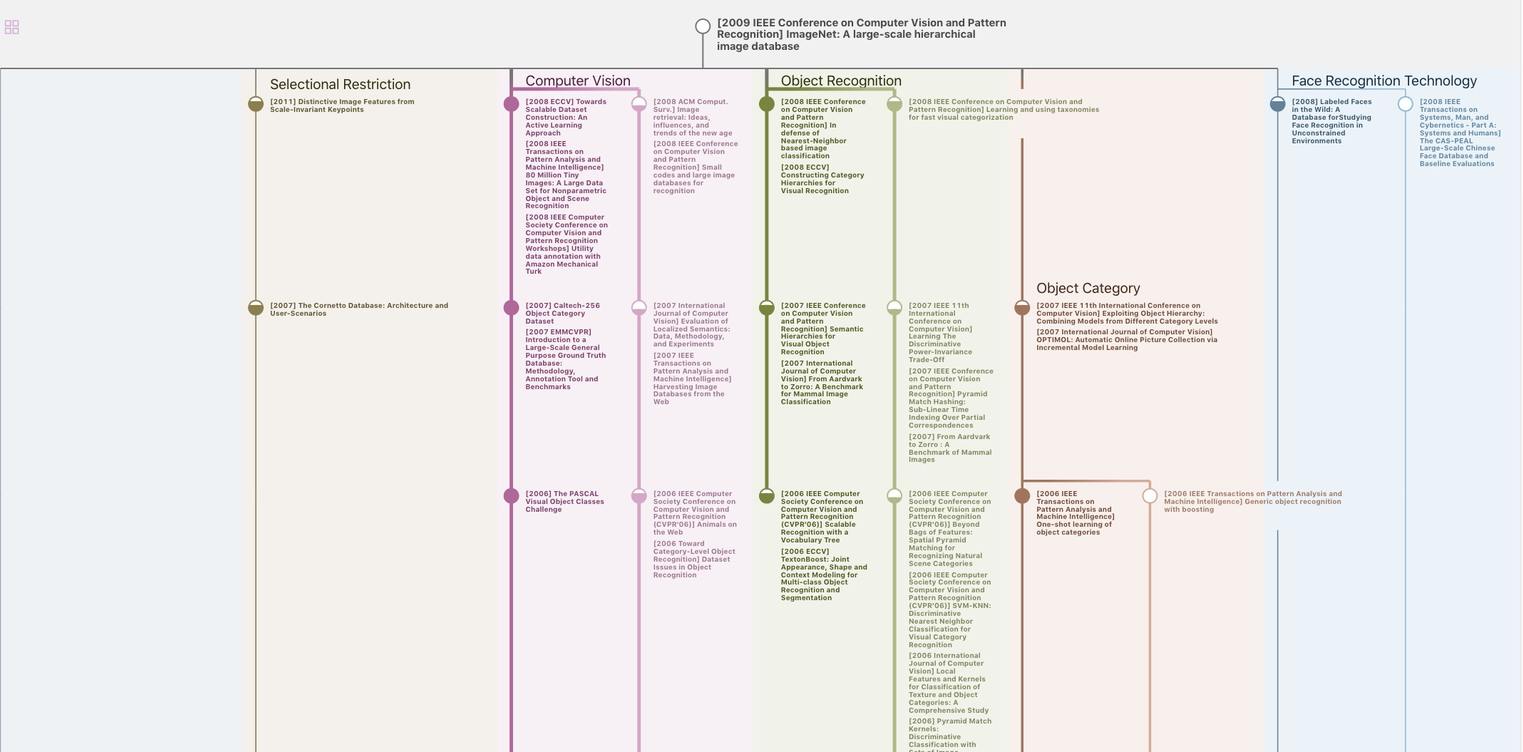
生成溯源树,研究论文发展脉络
Chat Paper
正在生成论文摘要