Distributionally Robust Chance-Constrained Optimization with Deep Kernel Ambiguity Set
2022 IEEE International Symposium on Advanced Control of Industrial Processes (AdCONIP)(2022)
Abstract
A distributionally robust chance-constrained programming (DRCCP) approach based on the deep kernel ambiguity set is proposed in this paper. The kernel ambiguity set possesses notable advantages over other existing ambiguity sets from the literature, and it is constructed by using the kernel mean embedding (KME) and the maximum mean discrepancy (MMD) between distributions. In the proposed method, the worst-case Conditional Value-at-Risk (CVaR) approximation is employed to approximate the distributionally robust joint chance constraint (DRJCC). Additionally, the performance of the presented method can be significantly enhanced by using the multi-layer deep arc-cosine kernel (MLACK), compared to the use of shallow kernels. The presented DRCCP approach is applied to a numeral example and a nonlinear process optimization problem to demonstrate its efficacy.
MoreTranslated text
Key words
kernel mean embedding,multilayer deep arc-cosine kernel,shallow kernels,distributionally robust chance-constrained optimization,deep kernel ambiguity set,DRCCP approach,DRJCC,maximum mean discrepancy,MMD,worst-case conditional value-at-risk approximation,CVaR approximation,distributionally robust chance-constrained programming approach,MLACK,nonlinear process optimization problem,KME
AI Read Science
Must-Reading Tree
Example
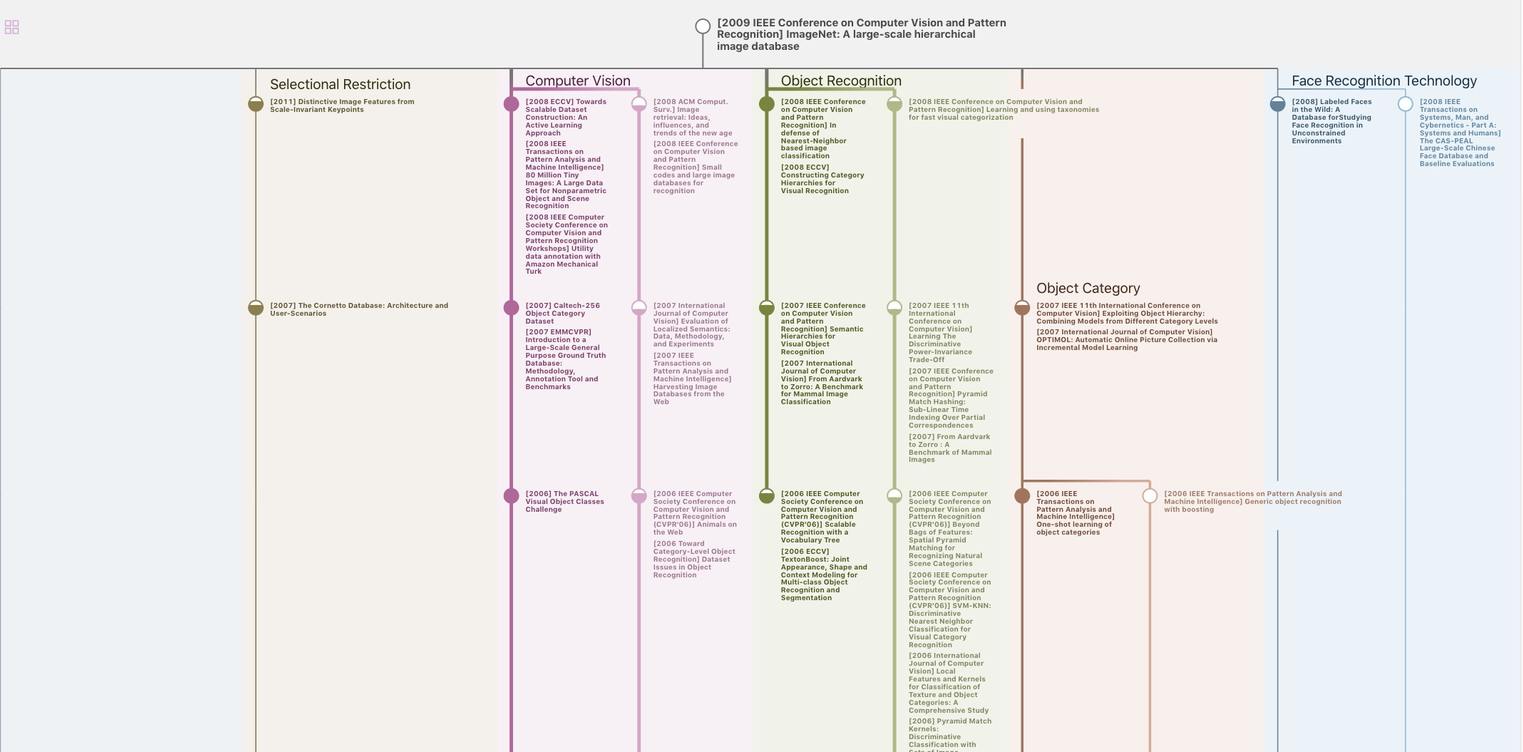
Generate MRT to find the research sequence of this paper
Chat Paper
Summary is being generated by the instructions you defined