Learning High-Order Semantic Representation for Intent Classification and Slot Filling on Low-Resource Language via Hypergraph
MATHEMATICAL PROBLEMS IN ENGINEERING(2022)
Abstract
Representation of language is the first and critical task for Natural Language Understanding (NLU) in a dialogue system. Pretraining, embedding model, and fine-tuning for intent classification and slot-filling are popular and well-performing approaches but are time consuming and inefficient for low-resource languages. Concretely, the out-of-vocabulary and transferring to different languages are two tough challenges for multilingual pretrained and cross-lingual transferring models. Furthermore, quality-proved parallel data are necessary for the current frameworks. Stepping over these challenges, different from the existing solutions, we propose a novel approach, the Hypergraph Transfer Encoding Network "HGTransEnNet. The proposed model leverages off-the-shelf high-quality pretrained word embedding models of resource-rich languages to learn the high-order semantic representation of low-resource languages in a transductive clustering manner of hypergraph modeling, which does not need parallel data. The experiments show that the representations learned by "HGTransEnNet" for low-resource language are more effective than the state-of-the-art language models, which are pretrained on a large-scale multilingual or monolingual corpus, in intent classification and slot-filling tasks on Indonesian and English datasets.
MoreTranslated text
Key words
intent classification,slot filling,high-order,low-resource
AI Read Science
Must-Reading Tree
Example
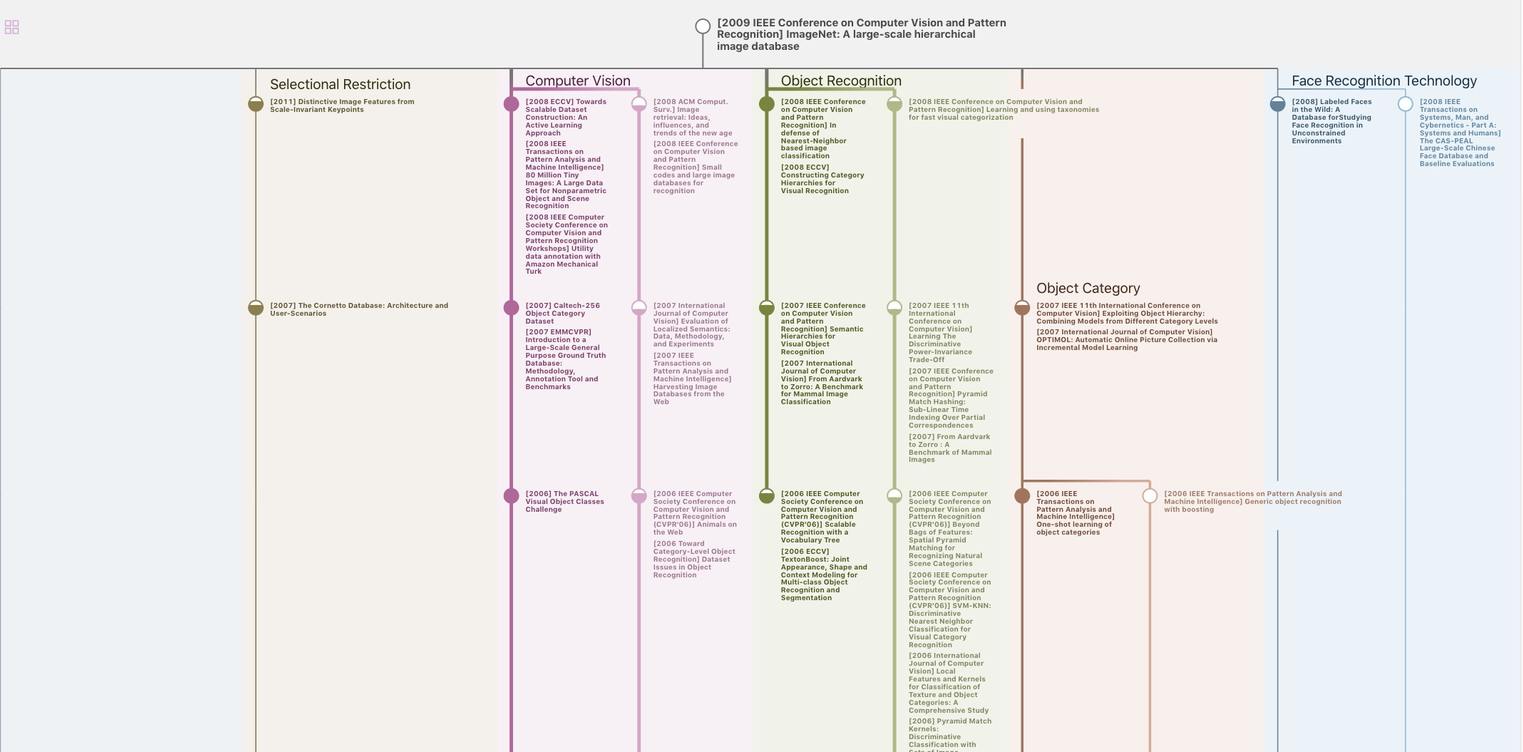
Generate MRT to find the research sequence of this paper
Chat Paper
Summary is being generated by the instructions you defined