CNN-Based Copy-Move Forgery Detection Using Rotation-Invariant Wavelet Feature
IEEE ACCESS(2022)
摘要
This paper introduces a machine learning based copy-move forgery (CMF) localization method. The basic convolutional neural network cannot be applied to CMF detection because CMF frequently involves rotation transformation. Therefore, we propose a rotation-invariant feature based on the root-mean squared energy using high-frequency wavelet coefficients. Instead of using three color image channels, two-scale energy features and low-frequency subband image are fed into the conventional VGG16 network. A correlation module is used by employing small feature patches generated by the VGG16 network to obtain the possible copied and moved patch pairs. The all-to-all similarity score is computed using the correlation module. To generate the final binary localization map, a simplified mask decoder module is introduced, which is composed of two simple bilinear upsampling and two batch-normalized-inception-based mask deconvolution followed by bilinear upsampling. We perform experiments on four test datasets and compare the proposed method with state-of-the-art tampering localization methods. The results demonstrate that the proposed scheme outperforms the existing approaches.
更多查看译文
关键词
Feature extraction, Forgery, Location awareness, Convolutional neural networks, Correlation, Decoding, Image segmentation, Root mean square, Wavelet transforms, Copy-move forgery, copy-move forgery localization, convolutional neural network, rotation-invariant, stationary wavelet transform, root-mean squared energy, simplified mask decoder module
AI 理解论文
溯源树
样例
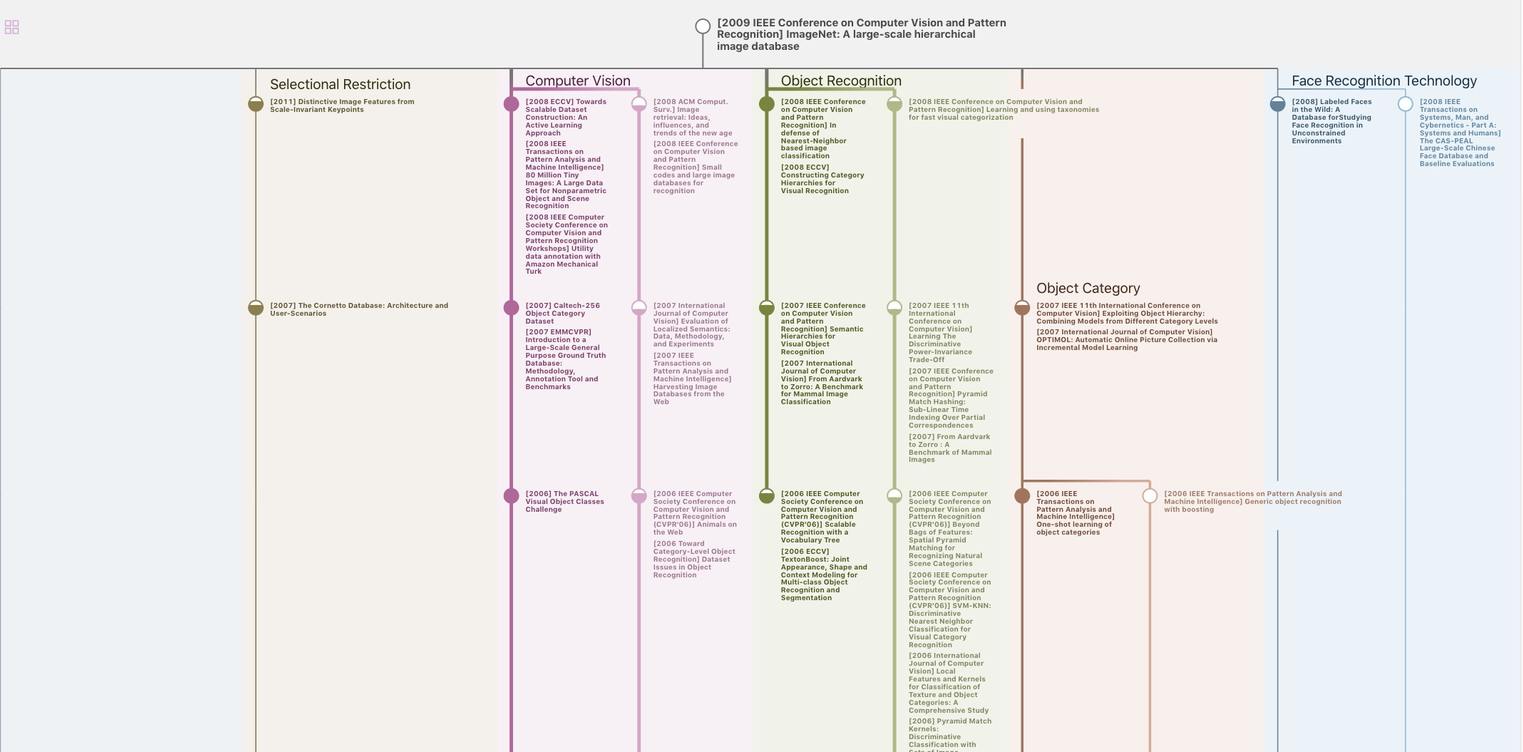
生成溯源树,研究论文发展脉络
Chat Paper
正在生成论文摘要