Link Prediction for Statistical Collaboration Networks Incorporating Institutes and Research Interests
IEEE ACCESS(2022)
Abstract
An interesting application of the link prediction technique is detecting the potential new links in collaboration networks. In this study, we construct collaboration networks based on the co-authorship information of the papers published in 43 statistical journals from 2001 to 2018. We construct training and testing networks according to the timestamps of the papers and construct a classification dataset for link prediction. We calculate 20 similarity indices based on the training network to perform link prediction. Additionally, we consider nodal attributes (institutes and research interests) to develop two novel predictors called the same institute (SIN) and keywords match count (KMC). Several machine-learning classifiers including support vector machine, XGBoost and random forest are implemented to combine all predictors. After incorporating SIN and KMC, we observe that the area under the receiver operating characteristic curve values of all classifiers improved, indicating that SIN and KMC can significantly improve classification accuracy. Finally, we provide collaborative recommendations for researchers based on the proposed model.
MoreTranslated text
Key words
Collaboration, Measurement, Machine learning, Finance, Predictive models, Stochastic processes, Collaboration network, link prediction, nodal attribute, similarity-based approach
AI Read Science
Must-Reading Tree
Example
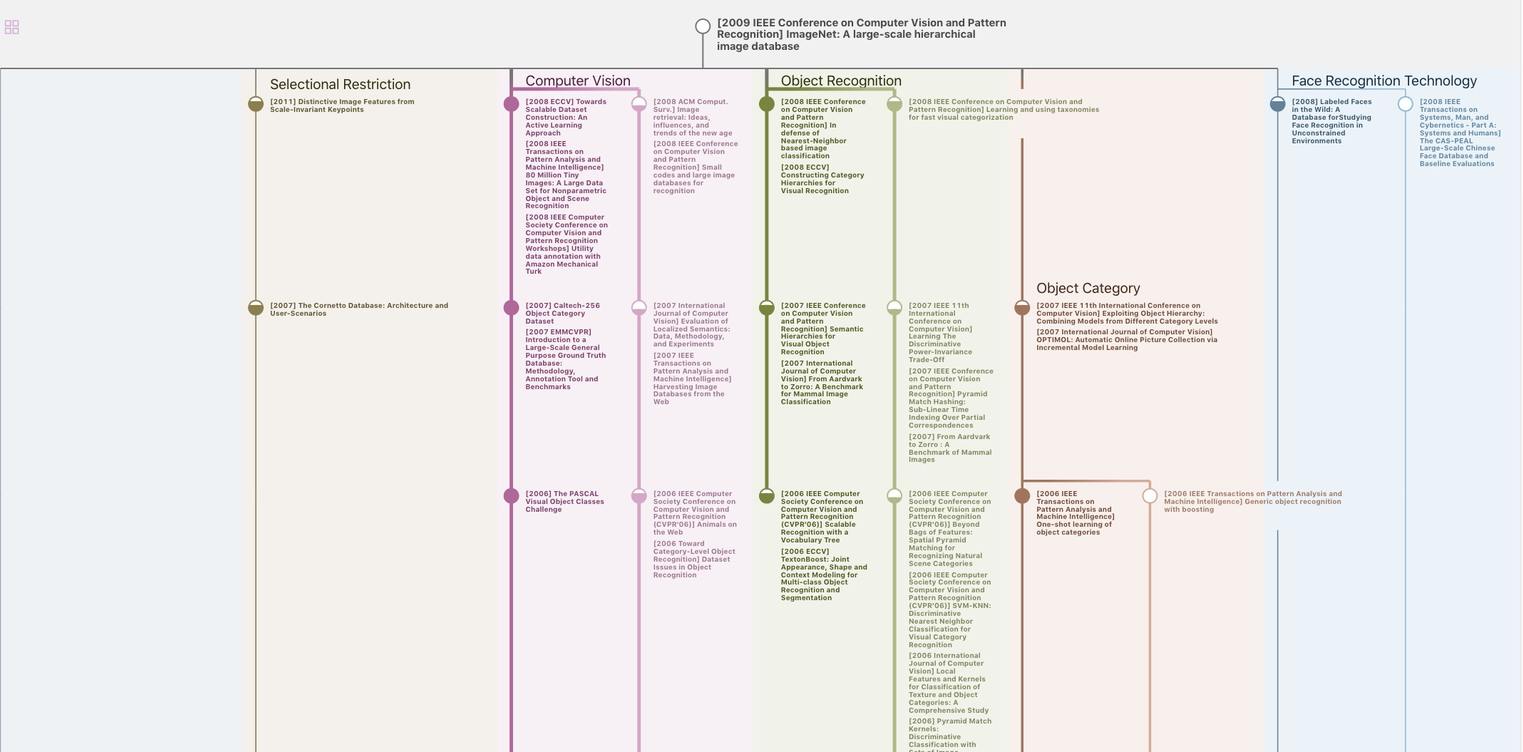
Generate MRT to find the research sequence of this paper
Chat Paper
Summary is being generated by the instructions you defined