More Precise Runtime Analyses of Non-elitist Evolutionary Algorithms in Uncertain Environments
ALGORITHMICA(2024)
摘要
Real-world applications often involve "uncertain" objectives, i.e., where optimisation algorithms observe objective values as a random variables with positive variance. In the past decade, several rigorous analysis results for evolutionary algorithms (EAs) on discrete problems show that EAs can cope with low-level uncertainties, i.e. when the variance of the uncertain objective value is small, and sometimes even benefit from uncertainty. Previous work showed that a large population combined with a non-elitist selection mechanism is a promising approach to handle high levels of uncertainty. However, the population size and the mutation rate can dramatically impact the performance of non-elitist EAs, and the optimal choices of these parameters depend on the level of uncertainty in the objective function. The performance and the required parameter settings for non-elitist EAs in some common objective-uncertainty scenarios are still unknown. We analyse the runtime of non-elitist EAs on two classical benchmark problems OneMax and LeadingOnes in in the one-bit, the bitwise, the Gaussian, and the symmetric noise models, and the dynamic binary value problem (DynBV). Our analyses are more extensive and precise than previous analyses of non-elitist EAs. In several settings, we prove that the non-elitist EAs outperform the current state-of-the-art results. Furthermore, we provide more precise guidance on how to choose the mutation rate, the selective pressure, and the population size as a function of the level of uncertainty.
更多查看译文
关键词
Non-elitist evolutionary algorithms,Uncertain environments,Noisy optimisation,Dynamic optimisation,Runtime analysis
AI 理解论文
溯源树
样例
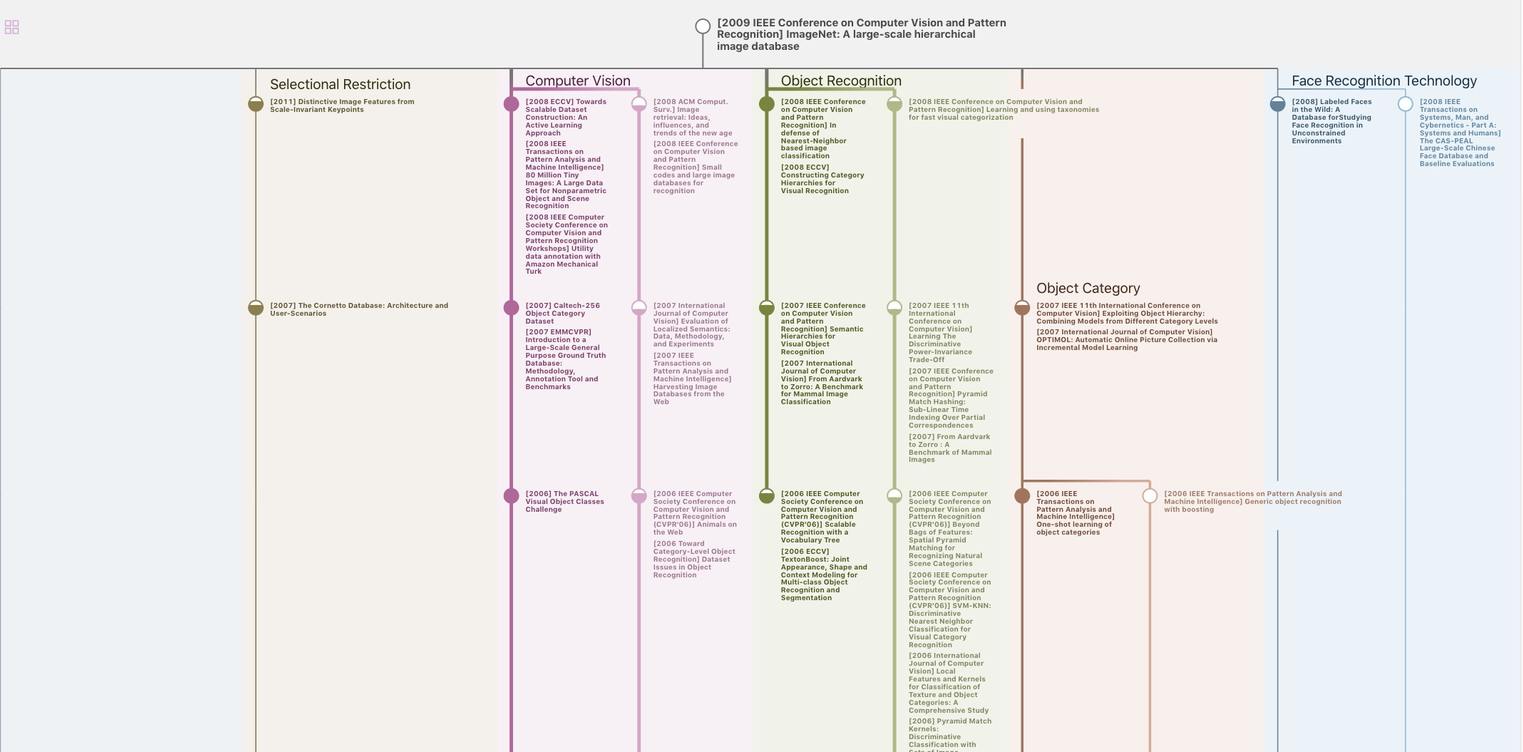
生成溯源树,研究论文发展脉络
Chat Paper
正在生成论文摘要