Balancing the Style-Content Trade-Off in Sentiment Transfer Using Polarity-Aware Denoising
TEXT, SPEECH, AND DIALOGUE (TSD 2022)(2022)
摘要
Text sentiment transfer aims to flip the sentiment polarity of a sentence (positive to negative or vice versa) while preserving its sentiment-independent content. Although current models show good results at changing the sentiment, content preservation in transferred sentences is insufficient. In this paper, we present a sentiment transfer model based on polarity-aware denoising, which accurately controls the sentiment attributes in generated text, preserving the content to a great extent and helping to balance the style-content trade-off. Our proposed model is structured around two key stages in the sentiment transfer process: better representation learning using a shared encoder and sentiment-controlled generation using separate sentiment-specific decoders. Empirical results show that our methods outperforms state-of-the-art baselines in terms of content preservation while staying competitive in terms of style transfer accuracy and fluency. Source code, data, and all other related details are available on Github (https://github.com/SOURO/polarity-denoising-sentiment-transfer).
更多查看译文
关键词
Sentiment Transfer, Text Style Transfer, Natural Language Generation
AI 理解论文
溯源树
样例
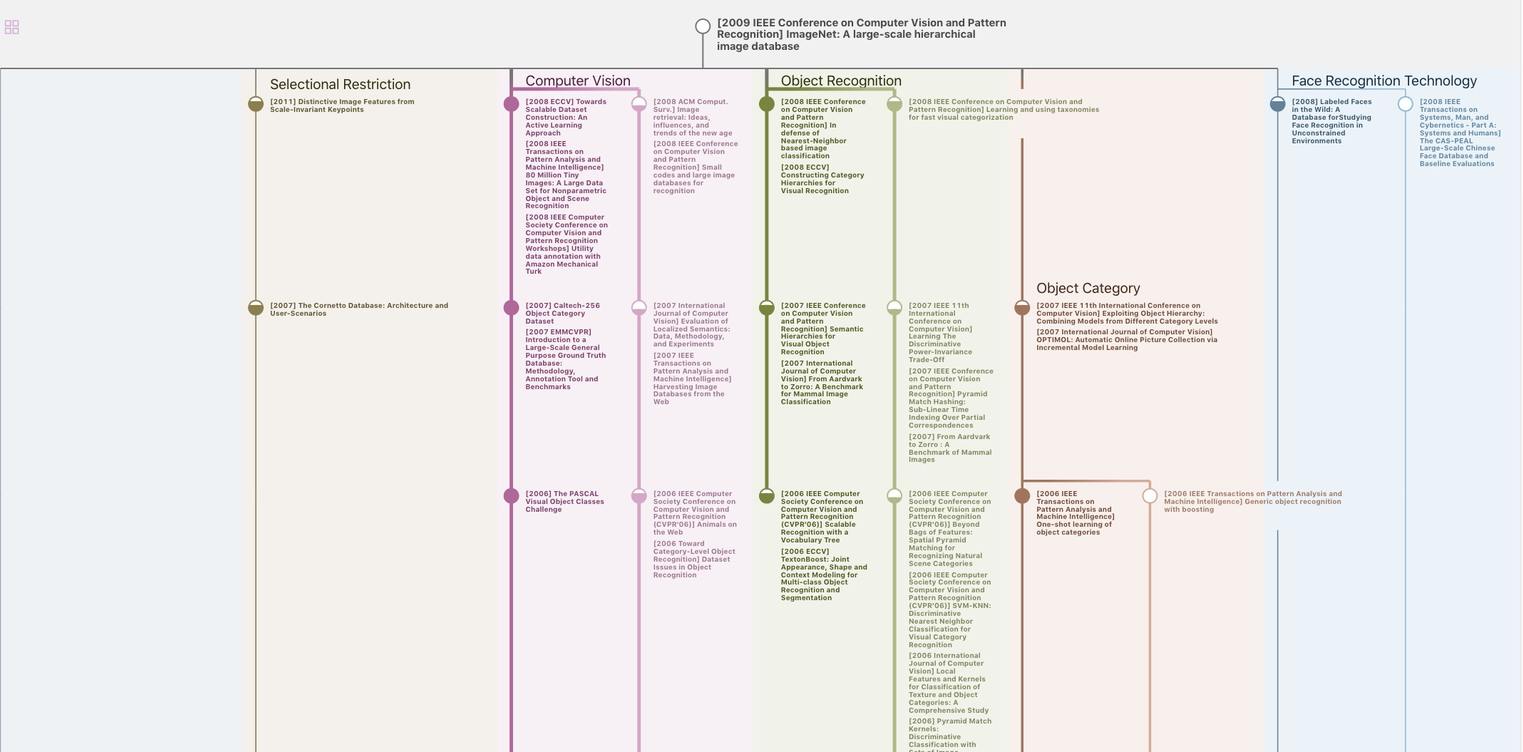
生成溯源树,研究论文发展脉络
Chat Paper
正在生成论文摘要