Fine-Tuning BERT for Generative Dialogue Domain Adaptation
TEXT, SPEECH, AND DIALOGUE (TSD 2022)(2022)
摘要
Current data-driven Dialogue State Tracking (DST) models exhibit a poor capacity to adapt themselves to domain changes, resulting in a significant degradation in performance. We propose a methodology, called Generative Dialogue Domain Adaptation, which significantly simplifies the creation of training data when a number of changes (e.g., new slot-values or new instances) occur in a domain Knowledge Base. We start from dialogues for a source domain and apply generative methods based on language models such as BERT, fine-tuned on task-related data and generate slot-values substitutions for a target domain. We have experimented dialogue domain adaptation in a few-shot setting showing promising results, although the task is still very challenging. We provide a deep analysis of the quality of the generated data and of the features that affect this task, and we emphasise that DST models are very sensitive to the distribution of slot-values in the corpus.
更多查看译文
关键词
Dialogue State Tracking, Task-oriented Dialogue, Domain Adaptation
AI 理解论文
溯源树
样例
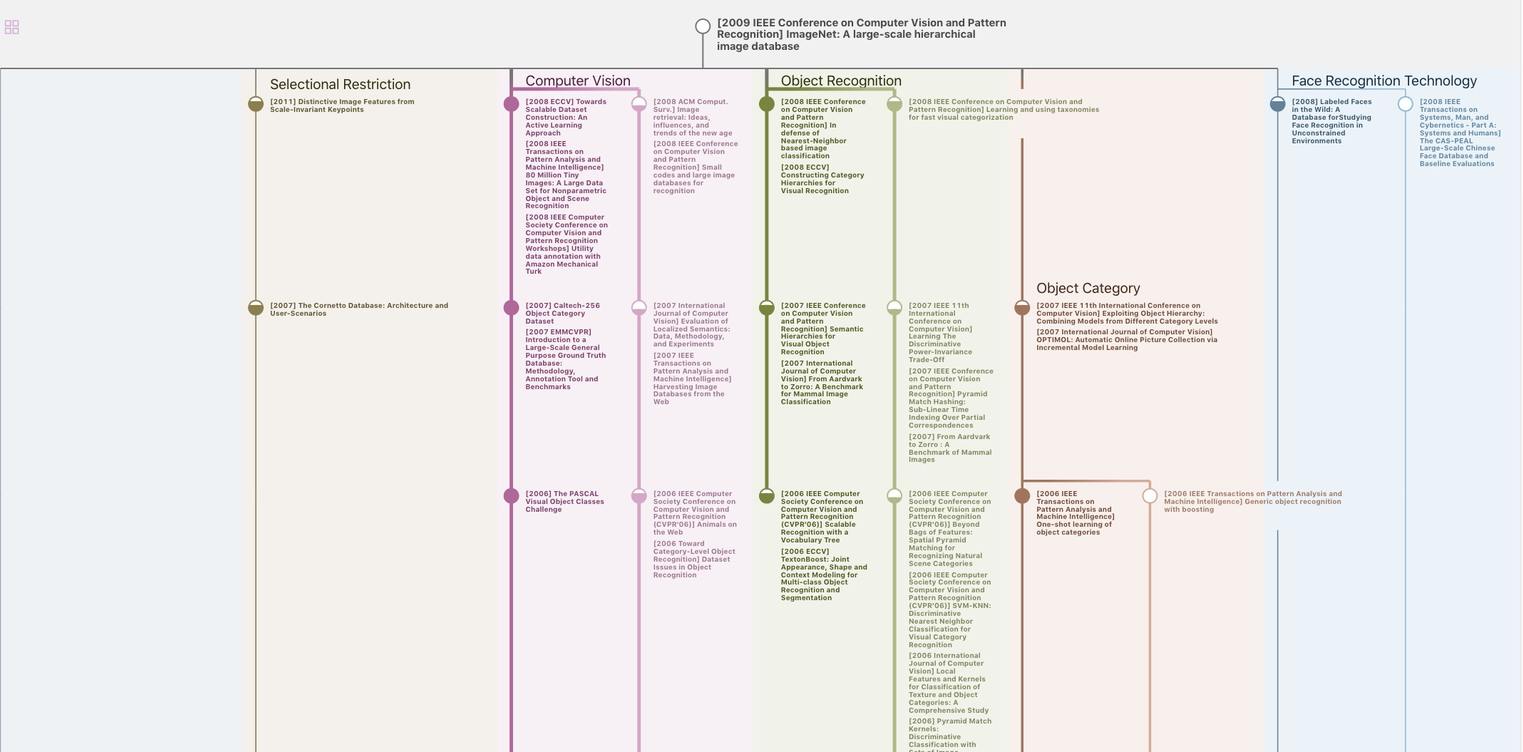
生成溯源树,研究论文发展脉络
Chat Paper
正在生成论文摘要