Design Exploration of An Energy-Efficient Acceleration System for CNNs on Low-Cost Resource-Constraint SoC-FPGAs
2022 IEEE INTERNATIONAL CONFERENCE ON ARTIFICIAL INTELLIGENCE CIRCUITS AND SYSTEMS (AICAS 2022): INTELLIGENT TECHNOLOGY IN THE POST-PANDEMIC ERA(2022)
摘要
Deep convolutional neural networks (CNNs) require enormous computation capacity, great amounts of memory accesses and data movement among parallel processing elements (PEs). From an energy perspective, CNNs are difficult to be fully deployed to low-cost resource-constraint edge devices because of both memory-intensive and computation-intensive workloads. In this paper, energy-efficient software/hardware co-design is explored for CNN acceleration on a Xilinx resource-constraint SoC-FPGA device. The acceleration system is optimized based on the constraints of DRAM bandwidths, BRAM resources, computing resources, optimal frequency and the complexity of wire routing. Moreover, the efficient workload distribution and dataflow control are also implemented by both software and hardware to achieve the maximum resource utilization. Based on a low-cost Xilinx Zynq XC7Z020 SoC-FPGA device, the proposed acceleration system achieves the throughput of VGG16 and YOLOv3-tiny by 4.3 frame/s and 21 frame/s, respectively. Moreover, 34 GOPS/W and 38.9 GOPS/W can be realized for VGG16 and YOLOv3-tiny. Compared to other state-of-art designs on resource-constraint SoC-FPGA devices, the proposed acceleration system achieves the best energy efficiency with high resource utilization.
更多查看译文
关键词
cnns,energy-efficient,low-cost,resource-constraint,soc-fpgas
AI 理解论文
溯源树
样例
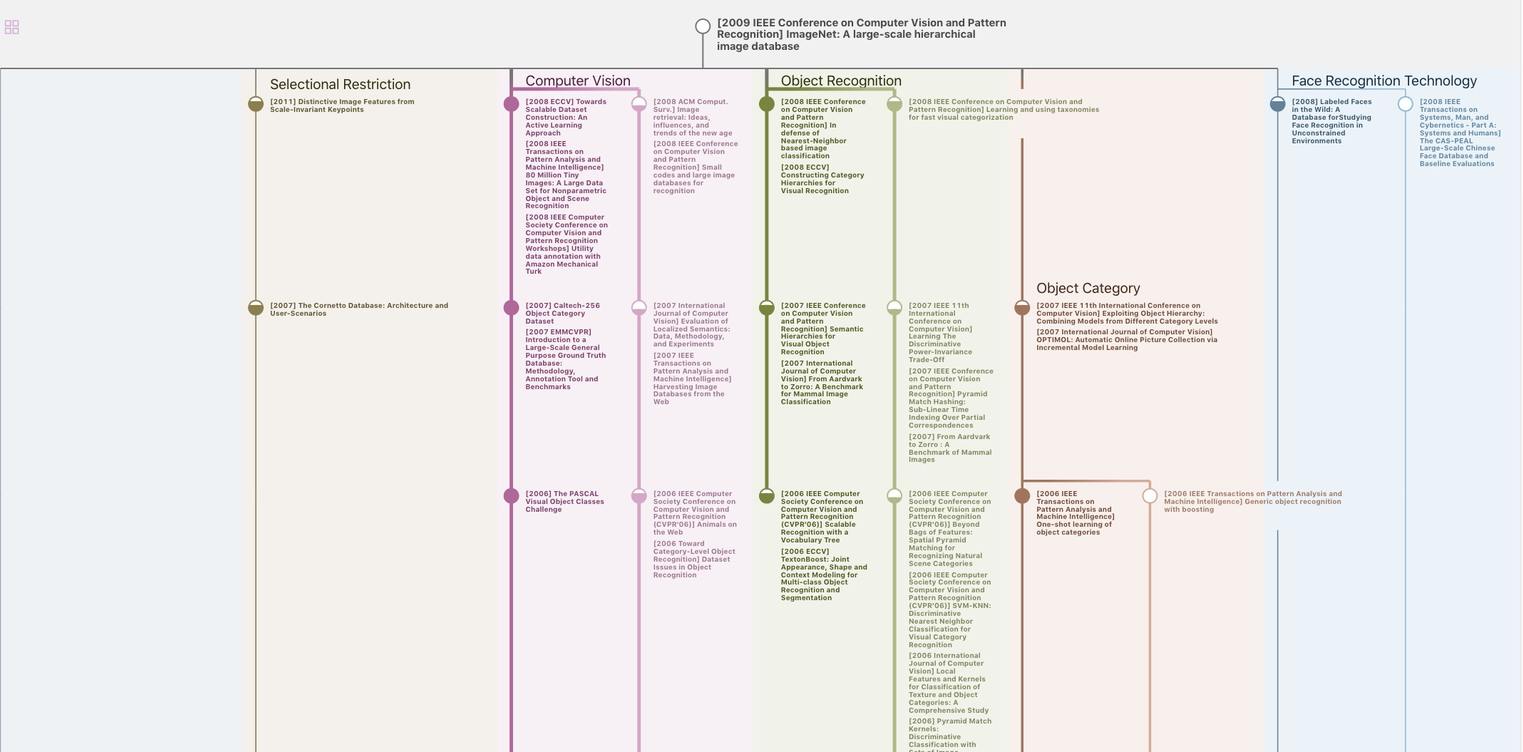
生成溯源树,研究论文发展脉络
Chat Paper
正在生成论文摘要