Identifying minimal set of Exploratory Landscape Analysis features for reliable algorithm performance prediction
2022 IEEE CONGRESS ON EVOLUTIONARY COMPUTATION (CEC)(2022)
摘要
Exploratory Landscape Analysis (ELA) enables the characterization of black-box optimization problem instances in the form of numerical features. Such features can be used to train a Machine Learning (ML) model to automatically predict the performance of an optimization algorithm on a specific problem instance. However, computing ELA features is a time consuming process and relatively expensive. In this paper, we aim to evaluate the usefulness of ELA features and identify features which are the most informative in automated algorithm performance prediction. The goal is to find a subset of features which are sufficient to train a reliable ML model for algorithm performance prediction, with reduced computational costs for calculating the ELA features. We focus on the performance prediction of the Covariance Matrix Adaptation Evolution Strategy (CMA-ES) algorithm on the COCO benchmark problems. The results showed that the number of ELA features that lead to a reliable algorithm performance prediction depends on the modular CMA-ES configuration under consideration. However, the set of features that are selected to be useful across different modular CMA-ES configurations are similar.
更多查看译文
关键词
automated algorithm performance prediction, exploratory landscape analysis, explainable models, feature selection
AI 理解论文
溯源树
样例
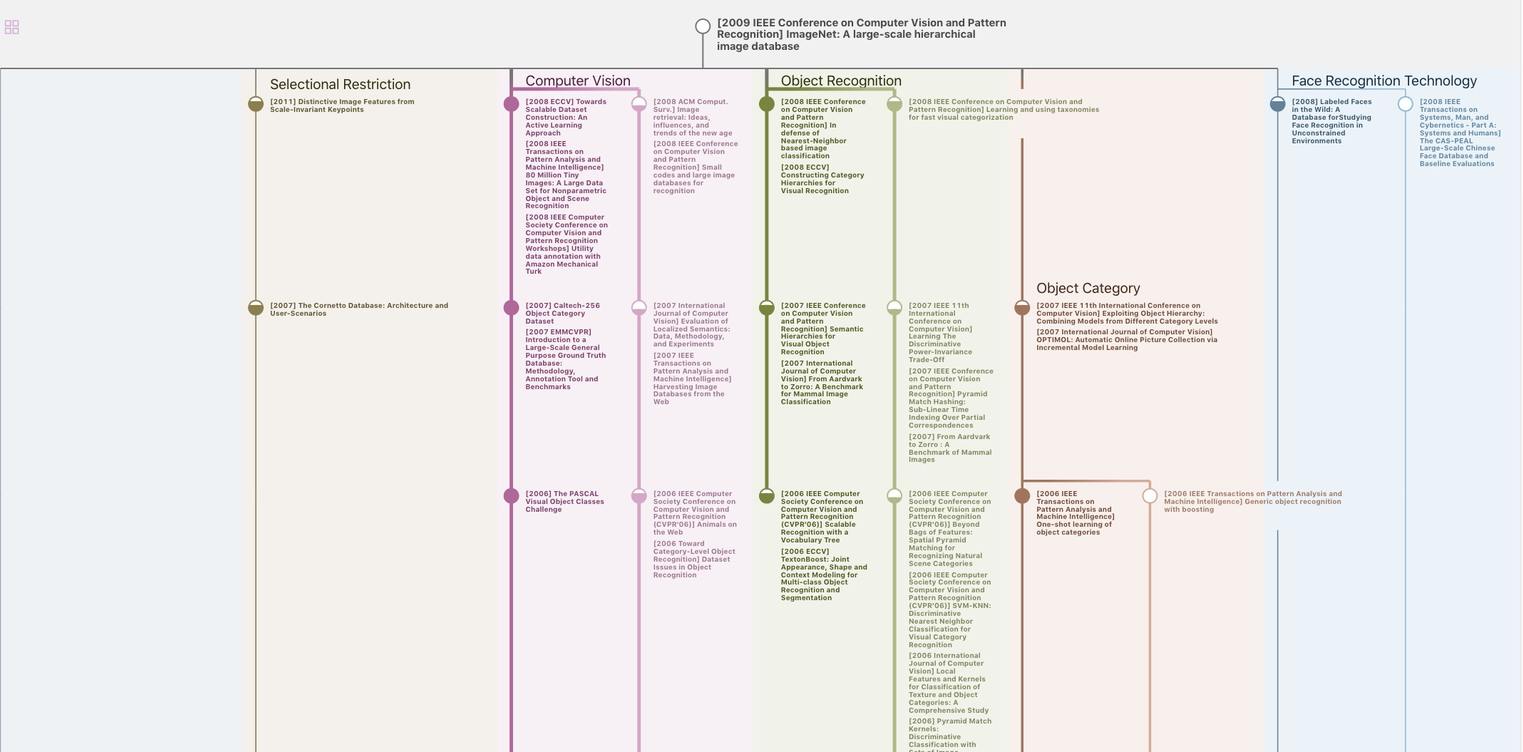
生成溯源树,研究论文发展脉络
Chat Paper
正在生成论文摘要