Adversarial Differential Evolution for Multimodal Optimization Problems
2022 IEEE CONGRESS ON EVOLUTIONARY COMPUTATION (CEC)(2022)
摘要
Multimodal optimization problems (MMOPs) are sorts of optimization problems that have many global optima. To discover as many peaks as possible and increase the accuracy of the solutions, MMOP requires algorithms with great exploration and exploitation abilities. However, exploration and exploitation are in an adversarial relationship, since exploration aims to locate more optima via searching the global space rather than small regions, whereas exploitation targets to enhance the accuracy of solutions via searching in small areas. The key to efficiently solving MMOPs lies in striking a balance between exploration and exploitation. To achieve the goal, this paper proposes an adversarial differential evolution (ADE), containing an adversarial reproduction strategy and an adversarial selection strategy. Firstly, adversarial reproduction strategy generates offspring for exploration and offspring for exploitation and lets these two types of offspring compete for survival. Secondly, adversarial selection strategy employs a diversity-optimization-based selection and a crowding-based selection to select the offspring with both good diversity and good fitness. Diversity-optimization-based selection transforms the problem of selecting diverse individuals into an optimizing problem and solves it via an extra genetic algorithm to get the offspring with optimal diversity. Extensive experiments are conducted on CEC2013 MMOP benchmark to verify the effectiveness and efficiency of the proposed ADE. Experimental results show that ADE has advantages over the state-of-the-art MMOP algorithms.
更多查看译文
关键词
Multimodal Optimization, Differential Evolution, Evolutionary Computation, Adversarial Strategies
AI 理解论文
溯源树
样例
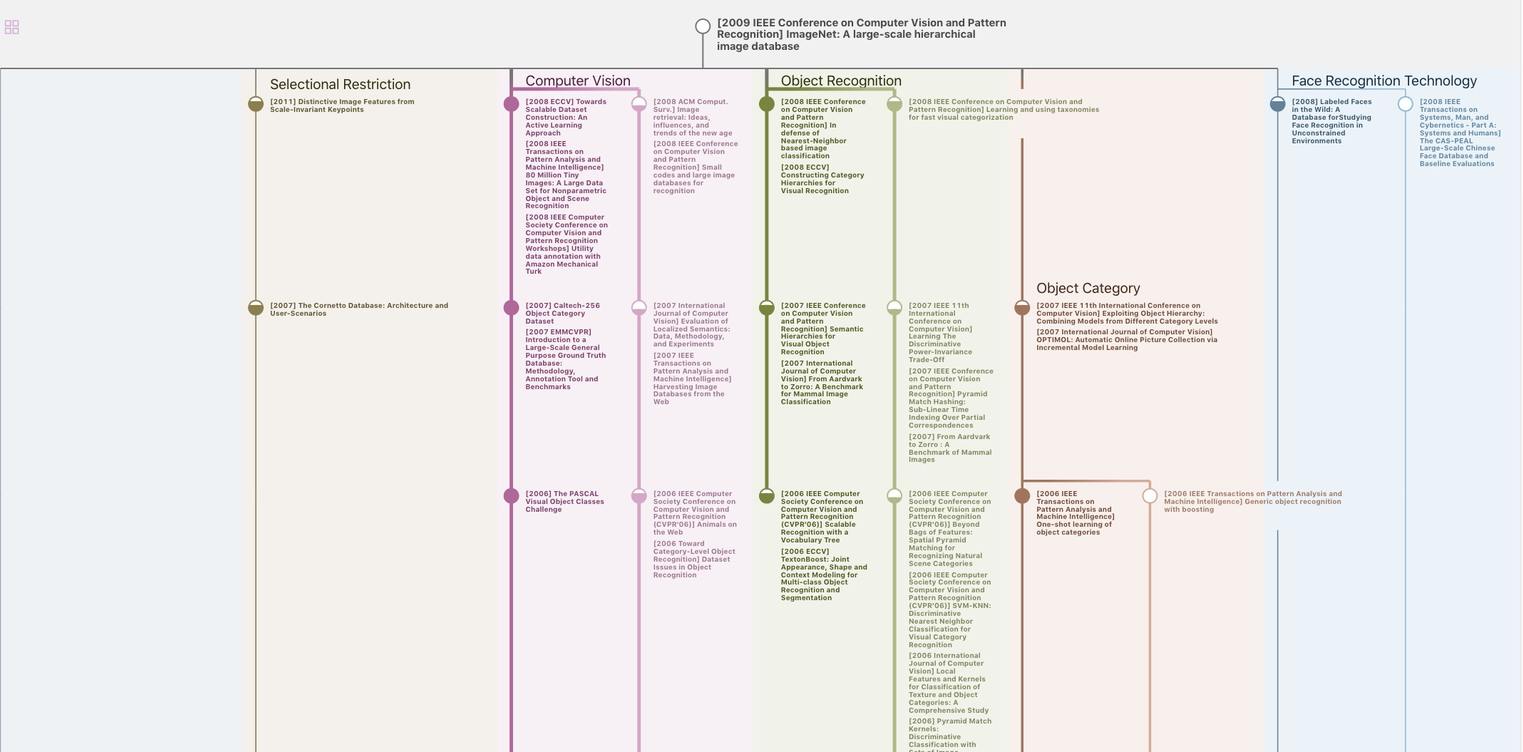
生成溯源树,研究论文发展脉络
Chat Paper
正在生成论文摘要