Surgical Scene Segmentation Using Semantic Image Synthesis with a Virtual Surgery Environment
MEDICAL IMAGE COMPUTING AND COMPUTER ASSISTED INTERVENTION, MICCAI 2022, PT VII(2022)
Abstract
The previous image synthesis research for surgical vision had limited results for real-world applications with simple simulators, including only a few organs and surgical tools and outdated segmentation models to evaluate the quality of the image. Furthermore, none of the research released complete datasets to the public enabling the open research. Therefore, we release a new dataset to encourage further study and provide novel methods with extensive experiments for surgical scene segmentation using semantic image synthesis with a more complex virtual surgery environment. First, we created three cross-validation sets of real image data considering demographic and clinical information from 40 cases of real surgical videos of gastrectomy with the da Vinci Surgical System (dVSS). Second, we created a virtual surgery environment in the Unity engine with five organs from real patient CT data and 22 the da Vinci surgical instruments from actual measurements. Third, We converted this environment photo-realistically with representative semantic image synthesis models, SEAN and SPADE. Lastly, we evaluated it with various state-of-the-art instance and semantic segmentation models. We succeeded in highly improving our segmentation models with the help of synthetic training data. More methods, statistics, and visualizations on https://sisyse.githubio/.
MoreTranslated text
Key words
Surgical instrument localization, Class imbalance, Domain randomization, Synthetic data, Semantic image snythesis
AI Read Science
Must-Reading Tree
Example
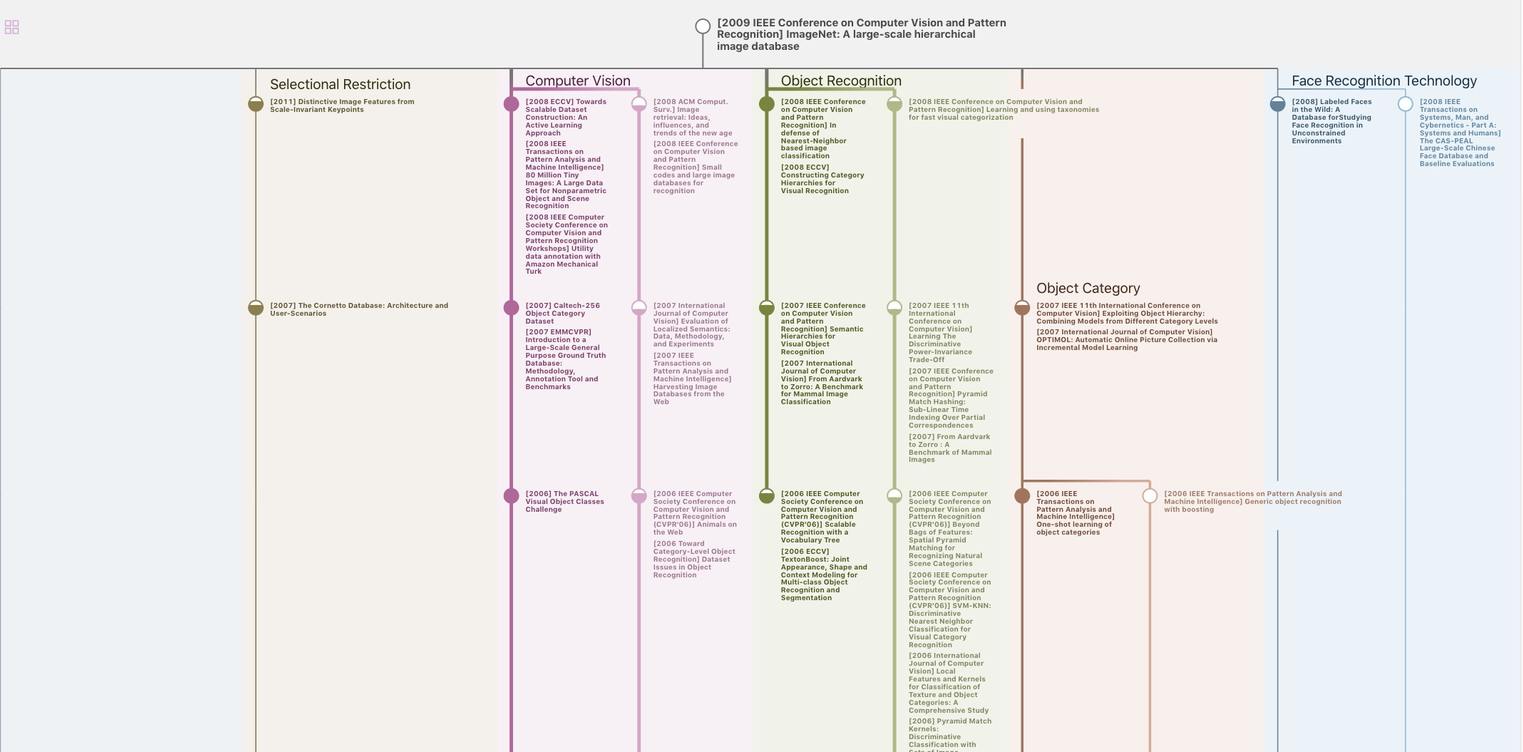
Generate MRT to find the research sequence of this paper
Chat Paper
Summary is being generated by the instructions you defined