Cross-lingual Transfer from Large Multilingual Translation Models to Unseen Under-resourced Languages
BALTIC JOURNAL OF MODERN COMPUTING(2022)
摘要
Low-resource machine translation has been a challenging problem to solve, with the lack of data being a big obstacle in producing good quality neural machine translation (NMT) sys-tems. However, recent work on developing large multilingual translation models gives a platform for attempts to create NMT systems for extremely low-resource languages that can achieve rea-sonable and usable quality. We leverage the information in large multilingual translation models by performing cross-lingual transfer learning to extremely low-resource Finno-Ugric languages. Our experiments include seven languages with limited resources that are unseen by the original pre-trained translation model and five high-resource languages that have the potential to help during training, previously seen by the model during training. We report state-of-the-art results on multiple test sets and translation directions as well as analyze the low-resource languages in smaller language groups in order to track the source of our higher translation quality.
更多查看译文
关键词
multilingual, cross -lingual transfer learning, low -resource, Finno-Ugric languages
AI 理解论文
溯源树
样例
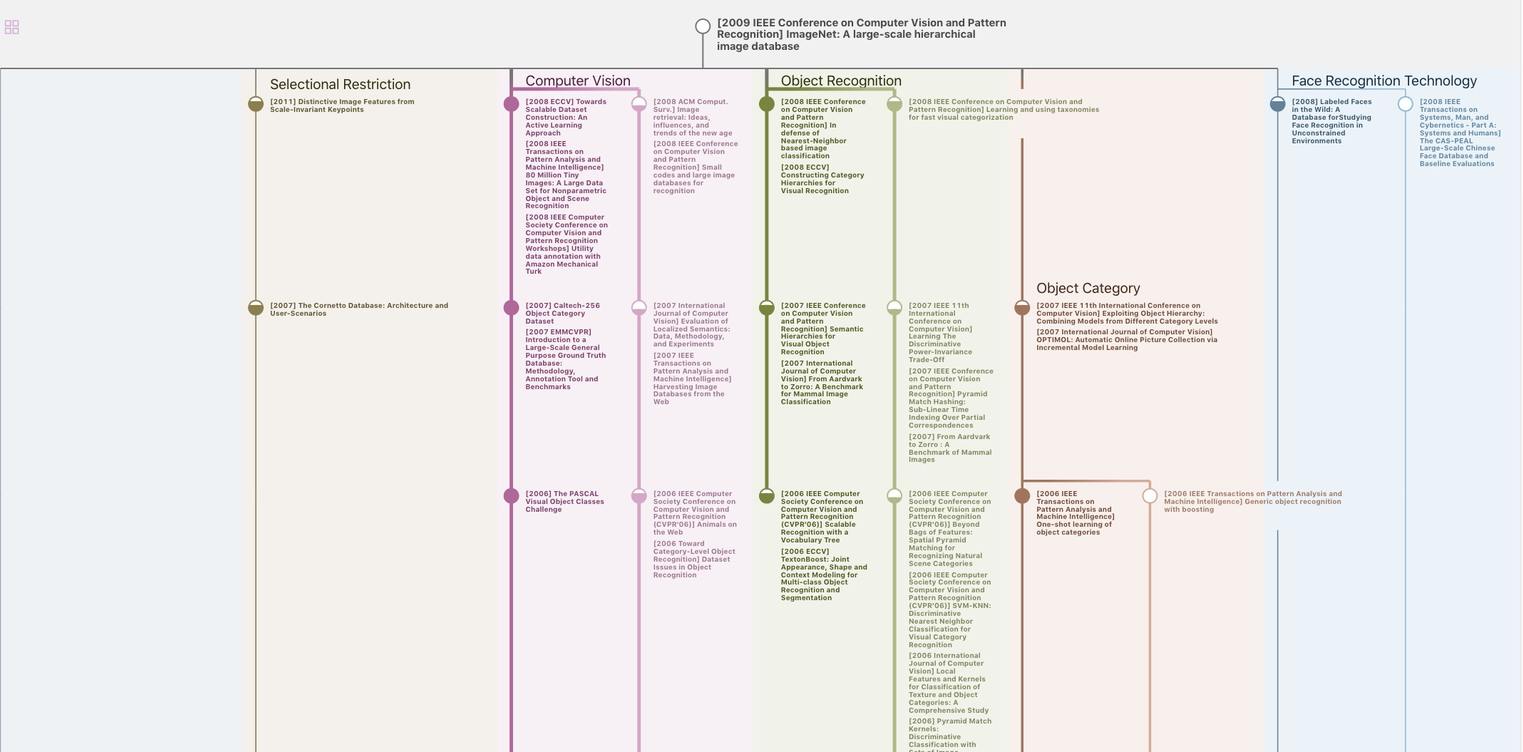
生成溯源树,研究论文发展脉络
Chat Paper
正在生成论文摘要