Enhancing Model Generalization for Substantia Nigra Segmentation Using a Test-time Normalization-Based Method
MEDICAL IMAGE COMPUTING AND COMPUTER ASSISTED INTERVENTION, MICCAI 2022, PT VII(2022)
摘要
Automatic segmentation of substantia nigra (SN), which is Parkinson's disease-related tissue, is an important step toward accurate computer-aided diagnosis systems. Conventional methods for SN segmentation depend heavily on limited magnetic resonance imaging (MRI) modalities such as neuromelanin and quantitative susceptibility mapping, which require longer imaging times and are rare in public datasets. To enable a multi-modal investigation for SN anatomic alterations based on medical bigdata researches, the need for automated SN segmentation arises from commonly investigated T2-weighted MRIs. To improve the performance of the automated SN segmentation from a T2-weighted MRI and enhance the model generalization for cross-center researches, this paper proposes a novel test-time normalization (TTN) method to increase the geometric and intensity similarity between the query data and the model's trained data. Our proposed method requires no additional training procedure or extra annotation for the unseen data. Our results showed that our proposed TTN achieved a mean Dice score of 71.08% in comparison with the baseline model's 69.87% score with in-house dataset. Additionally, improved SN segmentation performance was observed from the unseen and unlabeled datasets.
更多查看译文
关键词
Magnetic resonance imaging, Substantia nigra, Segmentation, Test-time normalization, Deep learning
AI 理解论文
溯源树
样例
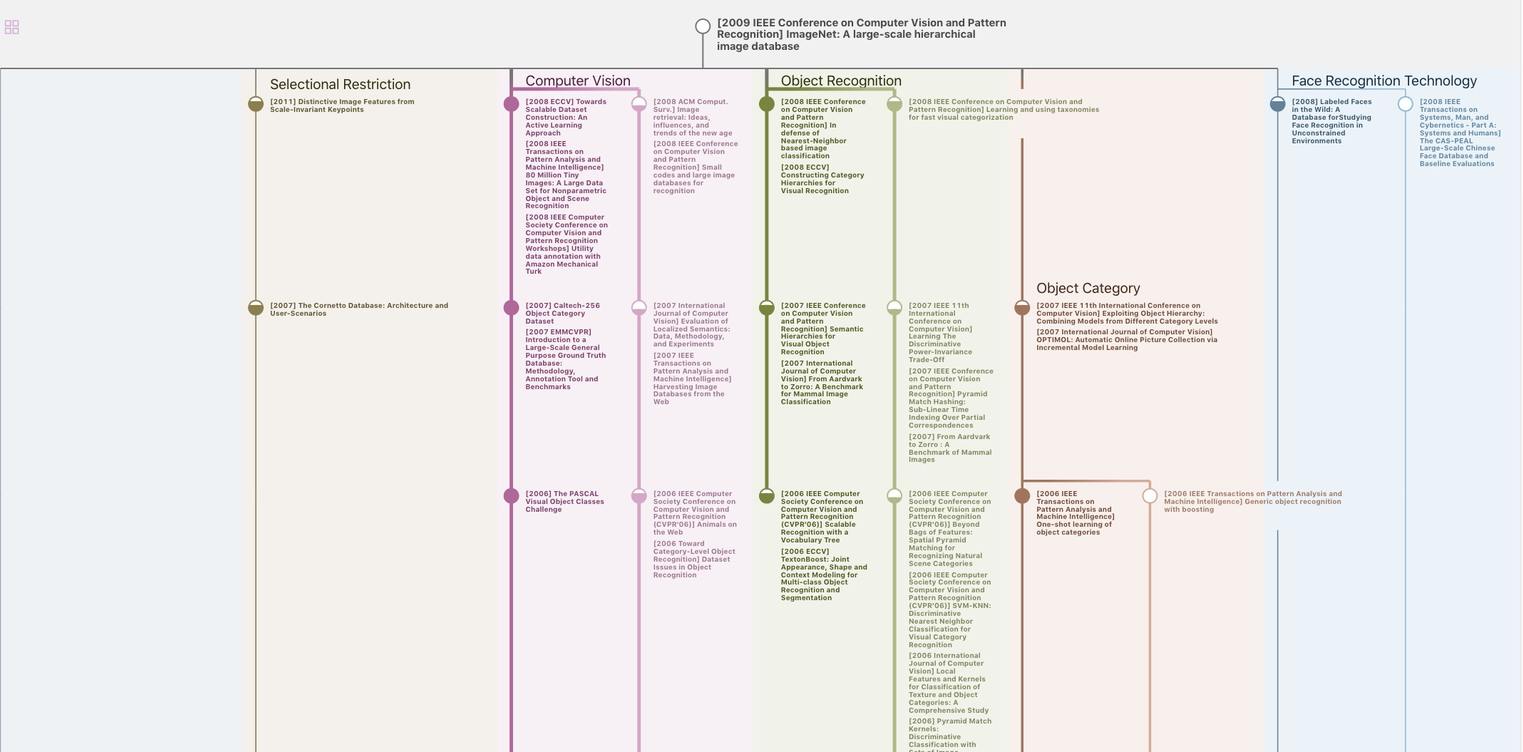
生成溯源树,研究论文发展脉络
Chat Paper
正在生成论文摘要