Alternating minimization algorithm with initialization analysis for r-local and k-sparse unlabeled sensing
arxiv(2022)
摘要
The unlabeled sensing problem is to recover an unknown signal from permuted linear measurements. We propose an alternating minimization algorithm with a suitable initialization for the widely considered k-sparse permutation model. Assuming either a Gaussian measurement matrix or a sub-Gaussian signal, we upper bound the initialization error for the r-local and k-sparse permutation models in terms of the block size $r$ and the number of shuffles k, respectively. Our algorithm is computationally scalable and, compared to baseline methods, achieves superior performance on real and synthetic datasets.
更多查看译文
关键词
minimization algorithm,initialization analysis,r-local,k-sparse
AI 理解论文
溯源树
样例
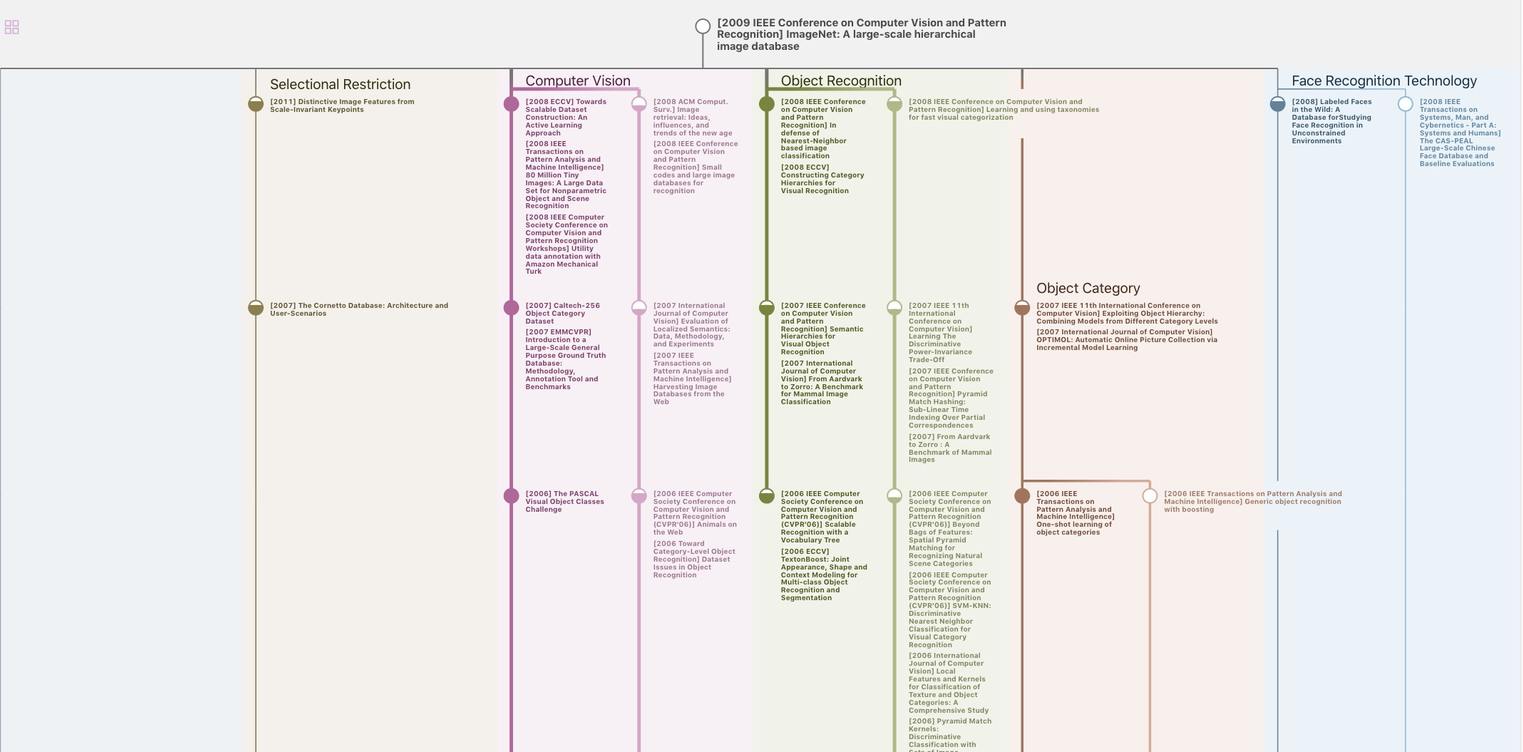
生成溯源树,研究论文发展脉络
Chat Paper
正在生成论文摘要