Relation Extraction based on Data Partition and Representation Integration
2021 IEEE Sixth International Conference on Data Science in Cyberspace (DSC)(2021)
摘要
Relation extraction (RE) is the cornerstone of many natural language processing applications. The success of machine learning algorithms generally depends on the embedding representation of data, since it involves different explanatory factors of variation behind the data. This paper explores integrating multiple representations to improve relation extraction. Note that the shortest dependency path (SDP) can retain relevant information and eliminate irrelevant words in the sentence, we partition the input dataset into multiple subsets based on the deformed SDP. For the input dataset and each subset, we train different encoders respectively. The input dataset encoder pays more attention to words in SDP, while the subset encoders pay more attention to words beyond SDP. In this way, we can get multiple embedding representations of diversity for the same sentence and improve the performance of RE by integrating them with predefined strategies. Experimental results on a widely used dataset demonstrate the effectiveness of our approach.
更多查看译文
关键词
relation extraction,dataset partition,representation integration,shortest dependency path,representation learning
AI 理解论文
溯源树
样例
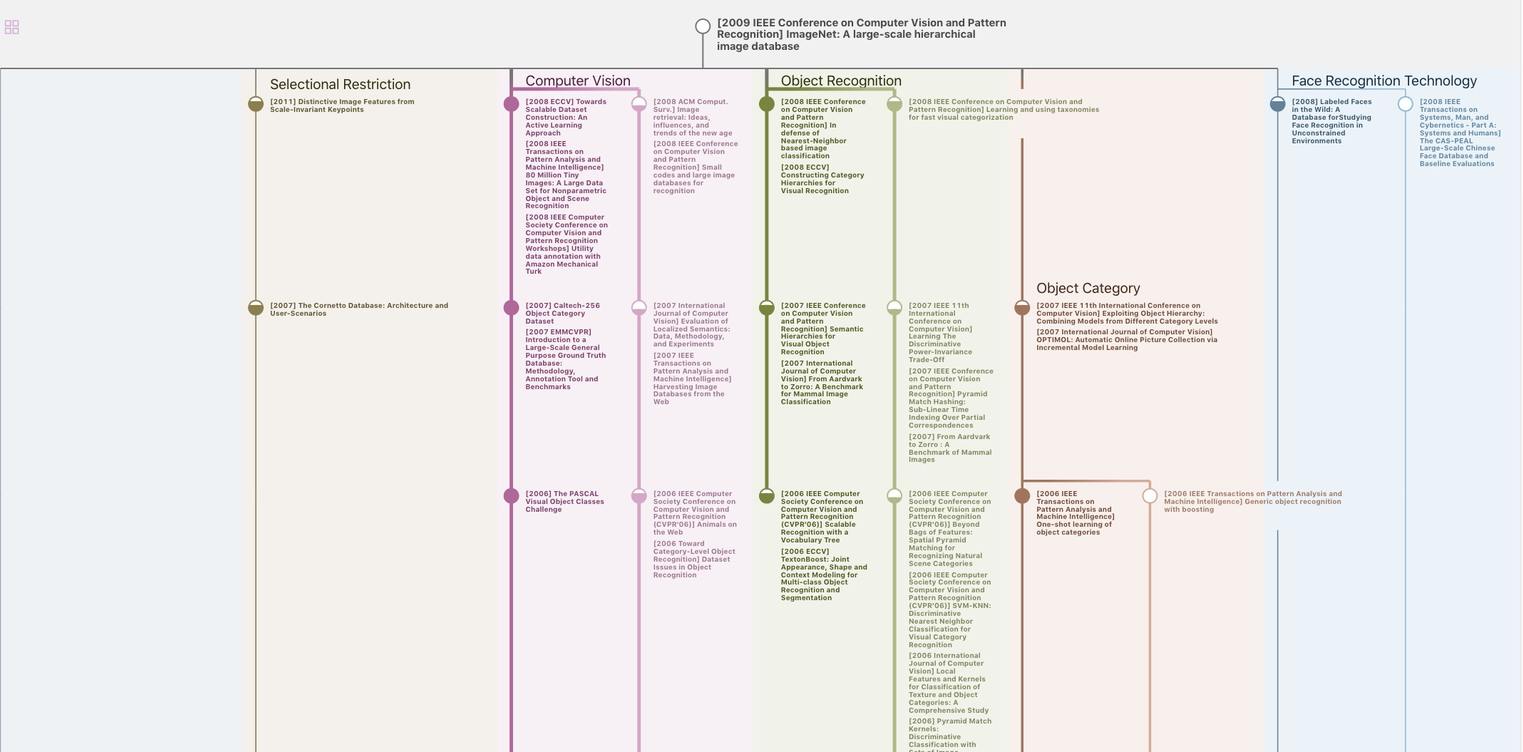
生成溯源树,研究论文发展脉络
Chat Paper
正在生成论文摘要