A monitoring framework for deployed machine learning models with supply chain examples
arxiv(2022)
摘要
Actively monitoring machine learning models during production operations helps ensure prediction quality and detection and remediation of unexpected or undesired conditions. Monitoring models already deployed in big data environments brings the additional challenges of adding monitoring in parallel to the existing modelling workflow and controlling resource requirements. In this paper, we describe (1) a framework for monitoring machine learning models; and, (2) its implementation for a big data supply chain application. We use our implementation to study drift in model features, predictions, and performance on three real data sets. We compare hypothesis test and information theoretic approaches to drift detection in features and predictions using the Kolmogorov-Smirnov distance and Bhattacharyya coefficient. Results showed that model performance was stable over the evaluation period. Features and predictions showed statistically significant drifts; however, these drifts were not linked to changes in model performance during the time of our study.
更多查看译文
关键词
machine learning models,monitoring framework,machine learning,chain examples
AI 理解论文
溯源树
样例
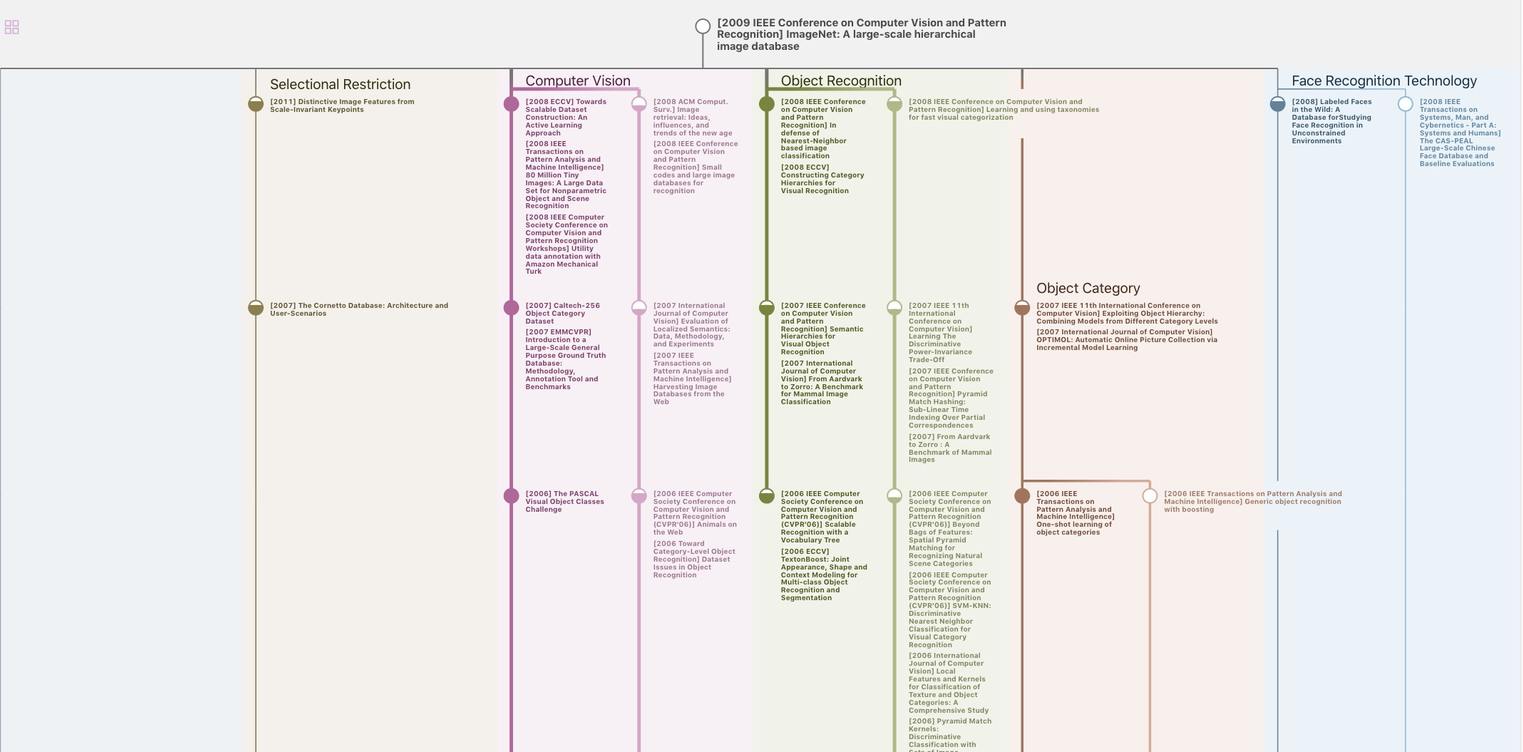
生成溯源树,研究论文发展脉络
Chat Paper
正在生成论文摘要