Efficient Domain Coverage for Vehicles with Second-Order Dynamics via Multi-Agent Reinforcement Learning
2023 IEEE/RSJ INTERNATIONAL CONFERENCE ON INTELLIGENT ROBOTS AND SYSTEMS (IROS)(2023)
摘要
Collaborative autonomous multi-agent systems covering a specified area have many potential applications. Traditional approaches for such problems involve designing model-based control policies; however, state-of-the-art classical control policy still exhibits a large degree of sub-optimality. We present a combined reinforcement learning (RL) and control approach for the multi-agent coverage problem involving agents with second-order dynamics, with the RL component being based on the Multi-Agent Proximal Policy Optimization Algorithm (MAPPO). Our proposed network architecture includes the incorporation of LSTM and self-attention, which allows the trained policy to adapt to a variable number of agents. Our trained policy significantly outperforms the state-of-the-art classical control policy. We demonstrate our proposed method in a variety of simulated experiments.
更多查看译文
AI 理解论文
溯源树
样例
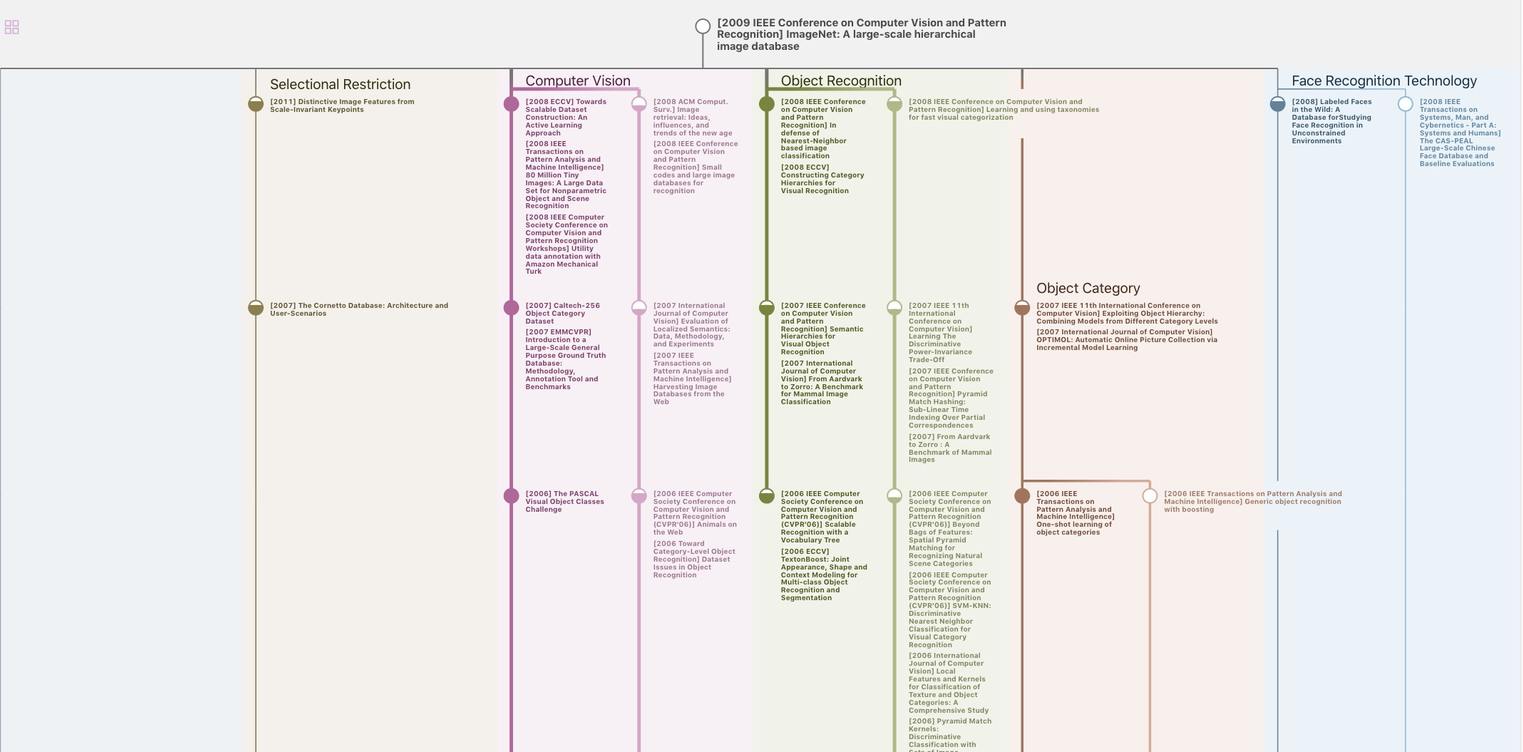
生成溯源树,研究论文发展脉络
Chat Paper
正在生成论文摘要