Comprehensive characterization of costimulatory molecule gene for diagnosis, prognosis and recognition of immune microenvironment features in sepsis.
Clinical immunology (Orlando, Fla.)(2022)
摘要
The present study, which involved 10 GEO datasets and 3 ArrayExpress datasets, comprehensively characterized the potential effects of CMGs in sepsis. Based on machine learning algorithms (Lasso, SVM and ANN), the CMG classifier was constructed by integrating 6 hub CMGs (CD28, CD40, LTB, TMIGD2, TNFRSF13C and TNFSF4). The CMG classifier exhibit excellent diagnostic values across multiple datasets and time points, and was able to distinguish sepsis from other critical diseases. The CMG classifier performed better in predicting mortality than other clinical characteristics or endotypes. More importantly, from clinical specimens, the CMG classifier showed more superior diagnostic values than PCT and CRP. Alternatively, the CMG classifier/hub CMGs is significantly correlated with immune cells infiltration (B cells, T cells, Tregs, and MDSC), pivotal immune and molecular pathways (inflammation-promoting, complement and coagulation cascades), and several cytokines. Collectively, CMG classifier was a robust tool for diagnosis, prognosis and recognition of immune microenvironment features in sepsis.
更多查看译文
关键词
Costimulatory molecule,Immune microenvironment,Machine learning approach,Model,Multi-transcriptome,sepsis
AI 理解论文
溯源树
样例
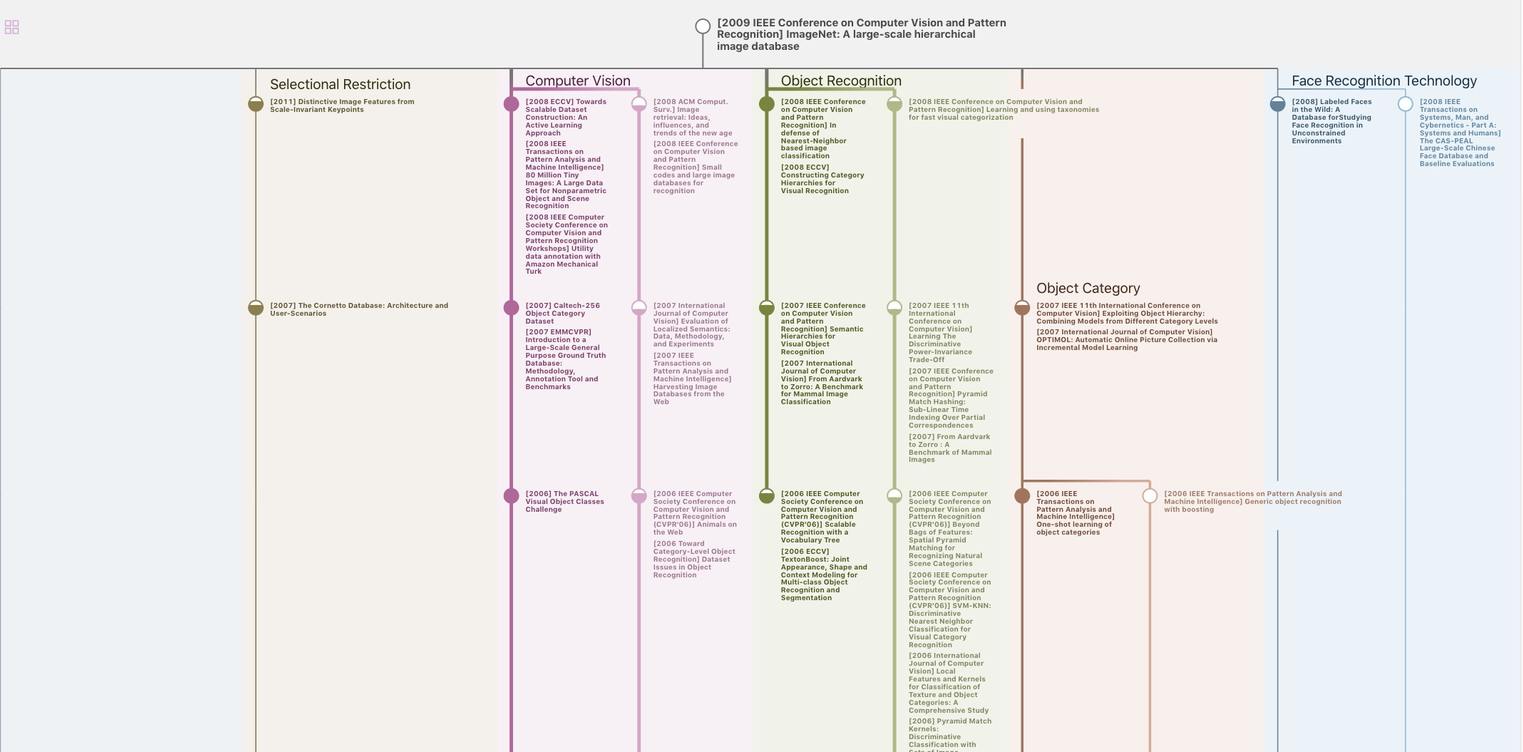
生成溯源树,研究论文发展脉络
Chat Paper
正在生成论文摘要