Fundamental Limits on the Computational Accuracy of Resistive Crossbar-based In-memory Architectures
2022 IEEE International Symposium on Circuits and Systems (ISCAS)(2022)
摘要
In-memory computing (IMC) architectures exhibit an intrinsic trade-off between computational accuracy and energy efficiency. This paper determines the fundamental limits on the compute SNR of MRAM-, ReRAM-, and FeFET-based crossbars by employing statistical signal and noise models. For a specific dot-product dimension N, the maximum compute SNR (SNR
max
) is shown to occur at an optimum value of sensing resistance $R_{s}^{*}$ where clipping and quantization noise contributions from the analog-to-digital converter (ADC) are balanced out. SNR
max
can be further improved by choosing devices with higher resistive contrast R
off
/R
on
, e.g., FeFET, but only until it attains a value in the range 12-15. Beyond this point, mismatch in the input digital-to-analog converters (DACs) and bitcell variations begin to dominate the compute SNR. Finally, by mapping a ResNet20 (CIFAR-10) network onto resistive crossbars, it is shown that the array-level compute SNR maximizing circuit parameters also maximizes the network-level accuracy.
更多查看译文
关键词
eNVM,MRAM,ReRAM,FeFET,SNR,in-memory computing,crossbar
AI 理解论文
溯源树
样例
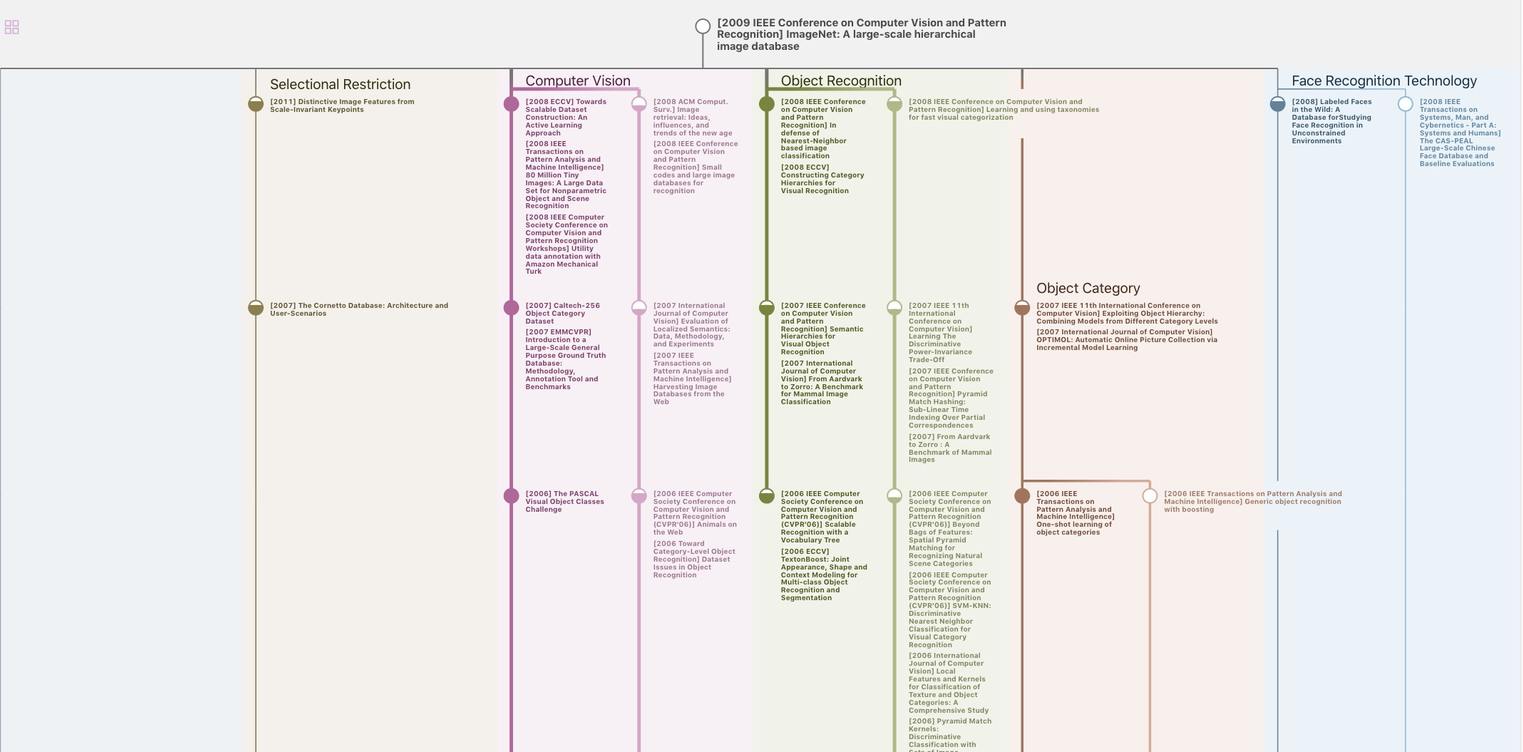
生成溯源树,研究论文发展脉络
Chat Paper
正在生成论文摘要