Opportunities for reinforcement learning in stochastic dynamic vehicle routing
Computers & Operations Research(2022)
摘要
There has been a paradigm-shift in urban logistic services in the last years; demand for real-time, instant mobility and delivery services grows. This poses new challenges to logistic service providers as the underlying stochastic dynamic vehicle routing problems (SDVRPs) require anticipatory real-time routing actions. The complexity of finding efficient routing actions is multiplied by the challenge of evaluating such actions with respect to their effectiveness given future dynamism and uncertainty. Reinforcement learning (RL) is a promising tool for evaluating actions but it is not designed for searching the complex and combinatorial action space. Thus, past work on RL for SDVRP has either restricted the action space, that is solving only subproblems by RL and everything else by established heuristics, or focused on problems that reduce to resource allocation problems. For solving real-world SDVRPs, new strategies are required that address the combined challenge of combinatorial, constrained action space and future uncertainty, but as our findings suggest, such strategies are essentially non-existing. Our survey paper shows that past work relied either on action-space restriction or avoided routing actions entirely and highlights opportunities for more holistic solutions.
更多查看译文
关键词
Stochastic dynamic vehicle routing,Reinforcement learning,Approximate dynamic programming,Mixed integer programming,Combinatorial optimization,Survey
AI 理解论文
溯源树
样例
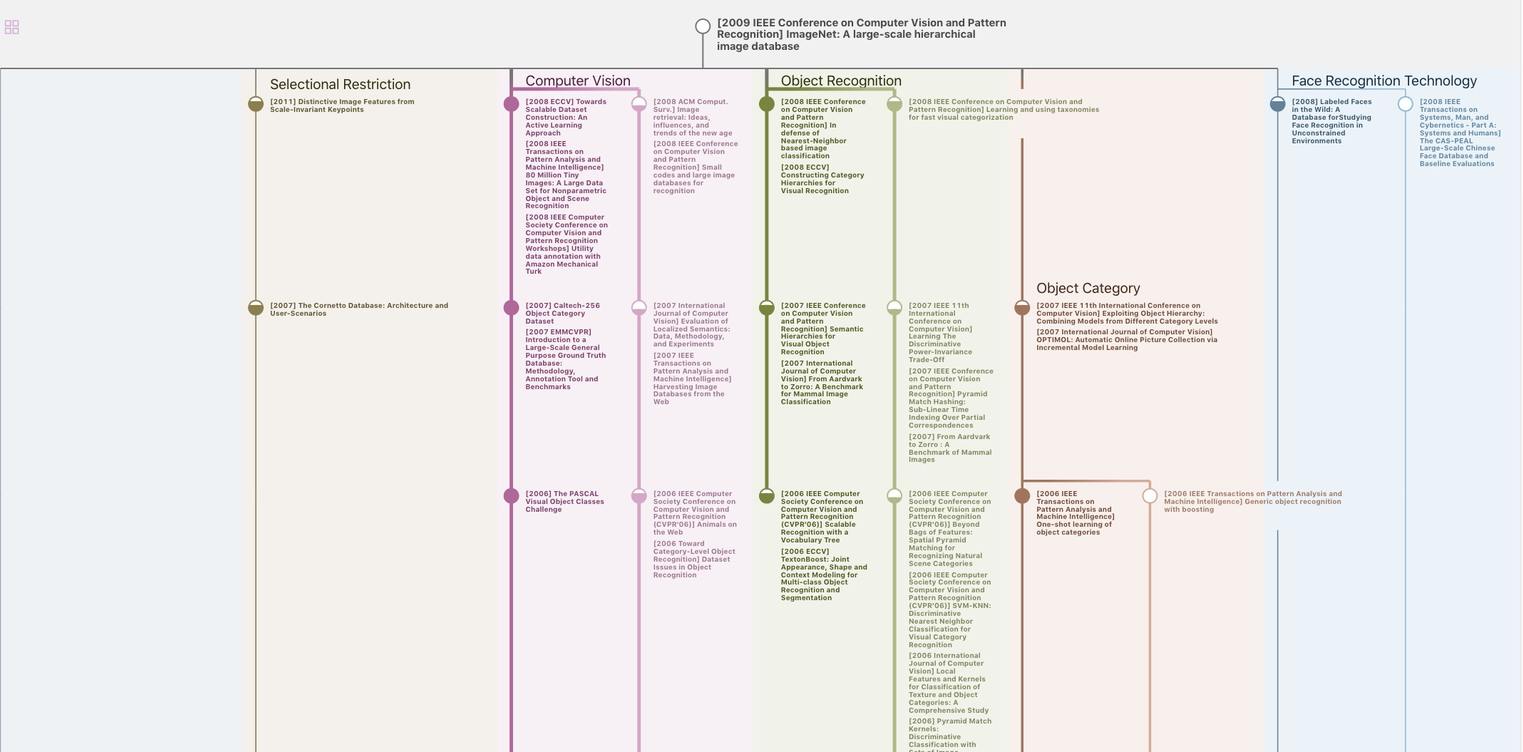
生成溯源树,研究论文发展脉络
Chat Paper
正在生成论文摘要