A novel deep learning pipeline for cell typing and phenotypic marker quantification in multiplex imaging
bioRxiv (Cold Spring Harbor Laboratory)(2022)
摘要
Background Multiplex immunofluorescence (mIF) can provide invaluable insights into spatial biology and the complexities of the immune tumor microenvironment (iTME). However, existing analysis approaches are both laborious and highly user-dependent. In order to overcome these limitations we developed a novel, end-to-end deep learning (DL) pipeline for rapid and accurate analysis of both tumor-microarray (TMA) and whole slide mIF images.
Methods Our pipeline consists of two DL models: a multi-classifier for classifying multi-channel cell images into 12 different cell types, and a binary classifier for determining the positivity of a given marker in single-channel images. The DL multi-classifier was trained on 7,000 tiles labeled with cell annotations from a publicly available CODEX dataset, consisting of 140 tissue cores from 35 colorectal cancer (CRC) patients. For the binary classifier training, the multi-channel tiles were further split into ∼100,000 single-channel tiles, for which the ground truth was inferred from the known expression of these markers in each cell-type. This DL binary classifier was then utilized to quantify the positivity of various cell state (phenotypic) markers. In addition, the binary classifier was exploited as a cell-typing tool, by predicting the positivity of individual lineage cell markers. The performance of our DL models was evaluated on 1,800 annotations from 14 test tissue cores. The models were further evaluated on a new 6-plex melanoma cohort, stained with PhenoImager®, and were compared to the performance of clustering, manual thresholding or machine learning-based cell-typing methods applied on the same test sets.
Results Our DL multi-classifier achieved highly accurate results, outperforming all of the tested cell-typing methods, including clustering, manual-thresholding and ML-based approaches, in both CODEX CRC and PhenoImager melanoma cohorts (accuracy of 91% and 87%, respectively), with F1-scores above 80% in the vast majority of cell types. Our DL binary classifier, which was trained solely on the lineage markers of the CRC dataset, also outperformed existing methods, demonstrating excellent F1-scores (>80%) for determining the positivity of unseen phenotypic and lineage markers across the two tumor types and imaging modalities. Notably, as little as 20 annotations were required in order to boost the performance on an unseen dataset to above 85% accuracy and 80% F1-scores. As a result, the DL binary classifier could successfully be used as a cell-typing model, in a manner that is transferable between experimental approaches.
Conclusions We present a novel state-of-the-art DL-based framework for multiplex imaging analysis, that enables accurate cell typing and phenotypic marker quantification, which is robust across markers, tumor indications, and imaging modalities.
### Competing Interest Statement
The authors have declared no competing interest.
更多查看译文
关键词
Bioimage Analysis,Cellular Imaging,Phenotypic Profiling,Lineage Tracking,Deep Learning
AI 理解论文
溯源树
样例
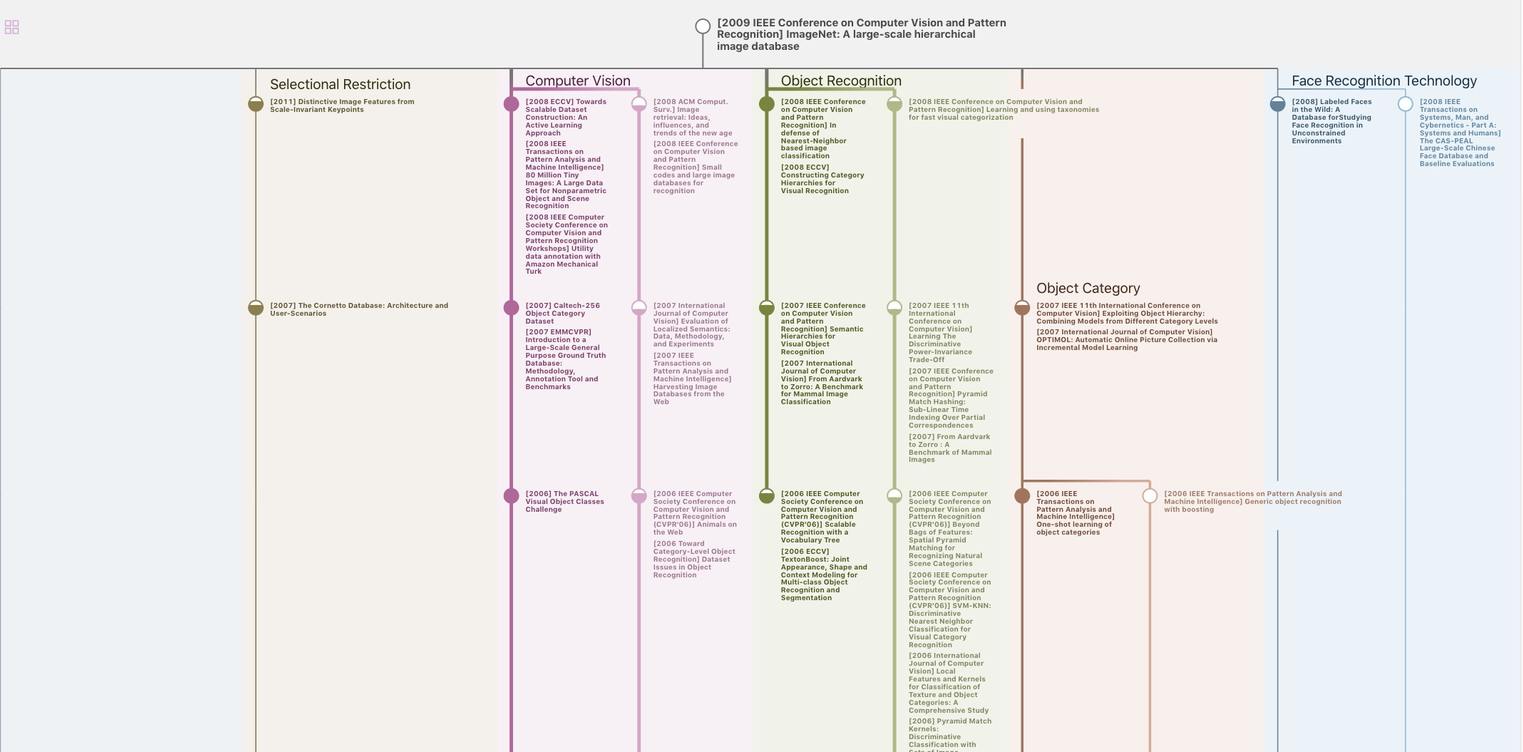
生成溯源树,研究论文发展脉络
Chat Paper
正在生成论文摘要