A New Framework of Swarm Learning Consolidating Knowledge From Multi-Center Non-IID Data for Medical Image Segmentation
IEEE TRANSACTIONS ON MEDICAL IMAGING(2023)
摘要
Large training datasets are important for deep learning-based methods. For medical image segmentation, it could be however difficult to obtain large number of labeled training images solely from one center. Distributed learning, such as swarm learning, has the potential to use multi-center data without breaching data privacy. However, data distributions across centers can vary a lot due to the diverse imaging protocols and vendors (known as feature skew). Also, the regions of interest to be segmented could be different, leading to inhomogeneous label distributions (referred to as label skew). With such non-independently and identically distributed (Non-IID) data, the distributed learning could result in degraded models. In this work, we propose a novel swarm learning approach, which assembles local knowledge from each center while at the same time overcomes forgetting of global knowledge during local training. Specifically, the approach first leverages a label skew-awared loss to preserve the global label knowledge, and then aligns local feature distributions to consolidate global knowledge against local feature skew. We validated our method in three Non-IID scenarios using four public datasets, including the Multi-Centre, Multi-Vendor and Multi-Disease Cardiac Segmentation (M & Ms) dataset, the Federated Tumor Segmentation (FeTS) dataset, the Multi-Modality Whole Heart Segmentation (MMWHS) dataset and the Multi-Site Prostate T2-weighted MRI segmentation (MSProsMRI) dataset. Results show that our method could achieve superior performance over existing methods. Code will be released via https://zmiclab.github.io/projects.html once the paper gets accepted.
更多查看译文
关键词
Medical image,non-IID,segmentation,swarm learning
AI 理解论文
溯源树
样例
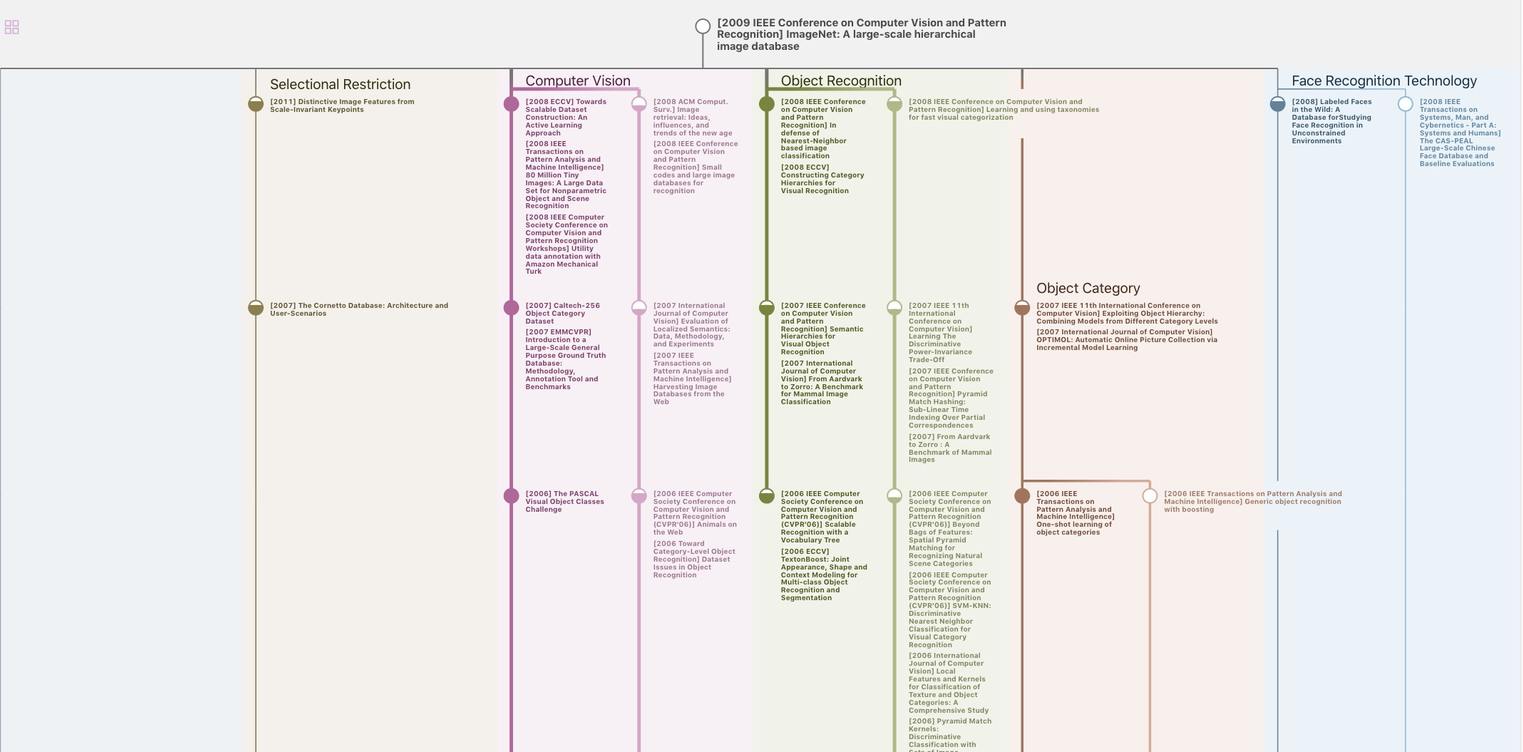
生成溯源树,研究论文发展脉络
Chat Paper
正在生成论文摘要