Shear thickening in dense bidisperse suspensions
JOURNAL OF RHEOLOGY(2023)
摘要
Discrete-particle simulations of bidisperse shear thickening suspensions are reported. The work considers two packing parameters, the large-to-small particle radius ratio ranging from delta = 1.4 (nearly monodisperse) to delta = 4, and the large particle fraction of the total solid loading with values zeta = 0.15, 0.5, and 0.85. Particle-scale simulations are performed over a broad range of shear stresses using a simulation model for spherical particles accounting for short-range lubrication forces, frictional interaction, and repulsion between particles. The variation of rheological properties and the maximum packing fraction phi(J) with shear stress sigma are reported. At a fixed volume fraction phi, bidispersity decreases the suspension relative viscosity eta(r) = eta(s)/eta(0), where eta(s) is the suspension viscosity and eta 0 is the suspending fluid viscosity, over the entire range of shear stresses studied. However, under low shear stress conditions, the suspension exhibits an unusual rheological behavior: the minimum viscosity does not occur as expected at zeta asymptotic to 0.5, but instead decreases with further increase of zeta to 0.85. The second normal stress difference N 2 acts similarly. This behavior is caused by particles ordering into a layered structure, as is also reflected by the zero slope with respect to time of the mean-square displacement in the velocity gradient direction. The relative viscosity eta(r) of bidisperse rate-dependent suspensions can be predicted by a power law linking it to phi(J), eta(r) = (1 - phi/phi(J))(-2) in both low and high shear stress regimes. The agreement between the power law and experimental data from literature demonstrates that the model captures well the effect of particle size distribution, showing that viscosity roughly collapses onto a single master curve when plotted against the reduced volume fraction phi/phi(J). (c) 2022 TheSociety of Rheology.
更多查看译文
关键词
bidispersity,dense suspensions,shear thickening
AI 理解论文
溯源树
样例
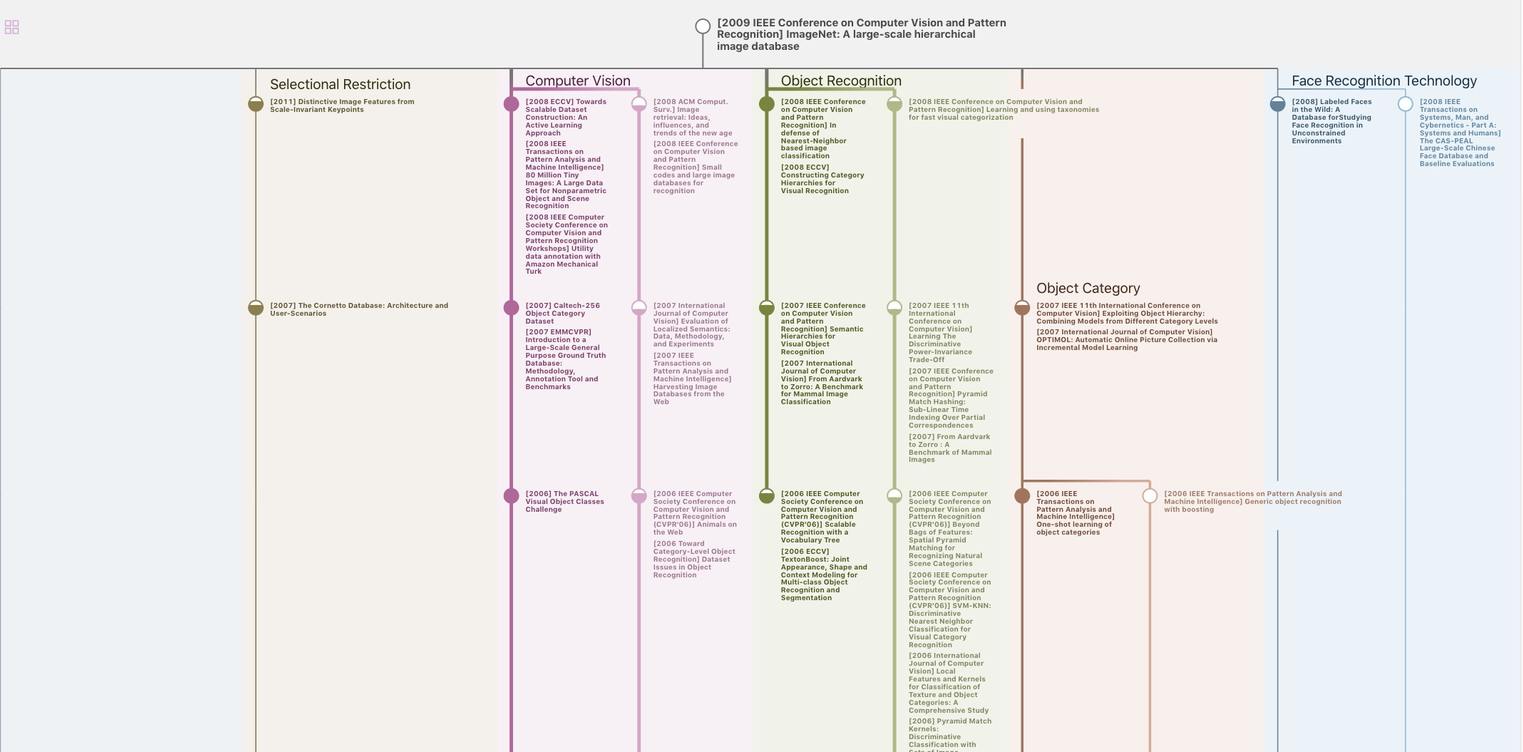
生成溯源树,研究论文发展脉络
Chat Paper
正在生成论文摘要