Deep W-Networks: Solving Multi-Objective Optimisation Problems With Deep Reinforcement Learning
arxiv(2023)
摘要
In this paper, we build on advances introduced by the Deep Q-Networks (DQN) approach to extend the multi-objective tabular Reinforcement Learning (RL) algorithm W-learning to large state spaces. W-learning algorithm can naturally solve the competition between multiple single policies in multi-objective environments. However, the tabular version does not scale well to environments with large state spaces. To address this issue, we replace underlying Q-tables with DQN, and propose an addition of W-Networks, as a replacement for tabular weights (W) representations. We evaluate the resulting Deep W-Networks (DWN) approach in two widely-accepted multi-objective RL benchmarks: deep sea treasure and multi-objective mountain car. We show that DWN solves the competition between multiple policies while outperforming the baseline in the form of a DQN solution. Additionally, we demonstrate that the proposed algorithm can find the Pareto front in both tested environments.
更多查看译文
关键词
reinforcement learning,optimisation,w-networks,multi-objective
AI 理解论文
溯源树
样例
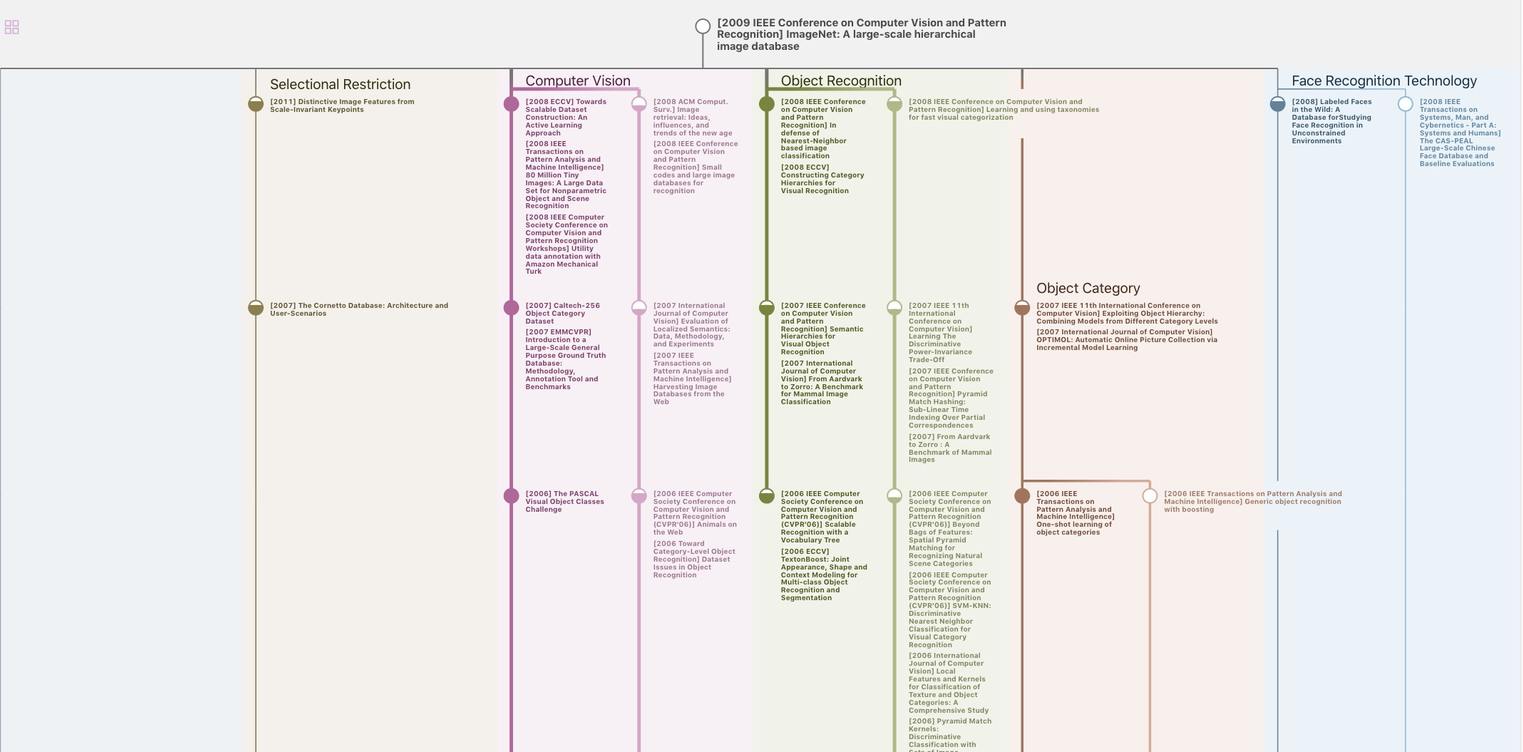
生成溯源树,研究论文发展脉络
Chat Paper
正在生成论文摘要