Machine learning for the prediction of post-ERCP pancreatitis risk: A proof-of-concept study.
Digestive and liver disease : official journal of the Italian Society of Gastroenterology and the Italian Association for the Study of the Liver(2022)
摘要
BACKGROUND:Predicting Post-Endoscopic Retrograde Cholangiopancreatography (ERCP) pancreatitis (PEP) risk can be determinant in reducing its incidence and managing patients appropriately, however studies conducted thus far have identified single-risk factors with standard statistical approaches and limited accuracy.
AIM:To build and evaluate performances of machine learning (ML) models to predict PEP probability and identify relevant features.
METHODS:A proof-of-concept study was performed on ML application on an international, multicenter, prospective cohort of ERCP patients. Data were split in training and test set, models used were gradient boosting (GB) and logistic regression (LR). A 10-split random cross-validation (CV) was applied on the training set to optimize parameters to obtain the best mean Area Under Curve (AUC). The model was re-trained on the whole training set with the best parameters and applied on test set. Shapley-Additive-exPlanation (SHAP) approach was applied to break down the model and clarify features impact.
RESULTS:One thousand one hundred and fifty patients were included, 6.1% developed PEP. GB model outperformed LR with AUC in CV of 0.7 vs 0.585 (p-value=0.012). GB AUC in test was 0.671. Most relevant features for PEP prediction were: bilirubin, age, body mass index, procedure time, previous sphincterotomy, alcohol units/day, cannulation attempts, gender, gallstones, use of Ringer's solution and periprocedural NSAIDs.
CONCLUSION:In PEP prediction, GB significantly outperformed LR model and identified new clinical features relevant for the risk, most being pre-procedural.
更多查看译文
关键词
Artificial intelligence,ERCP,Machine learning,Pancreatitis
AI 理解论文
溯源树
样例
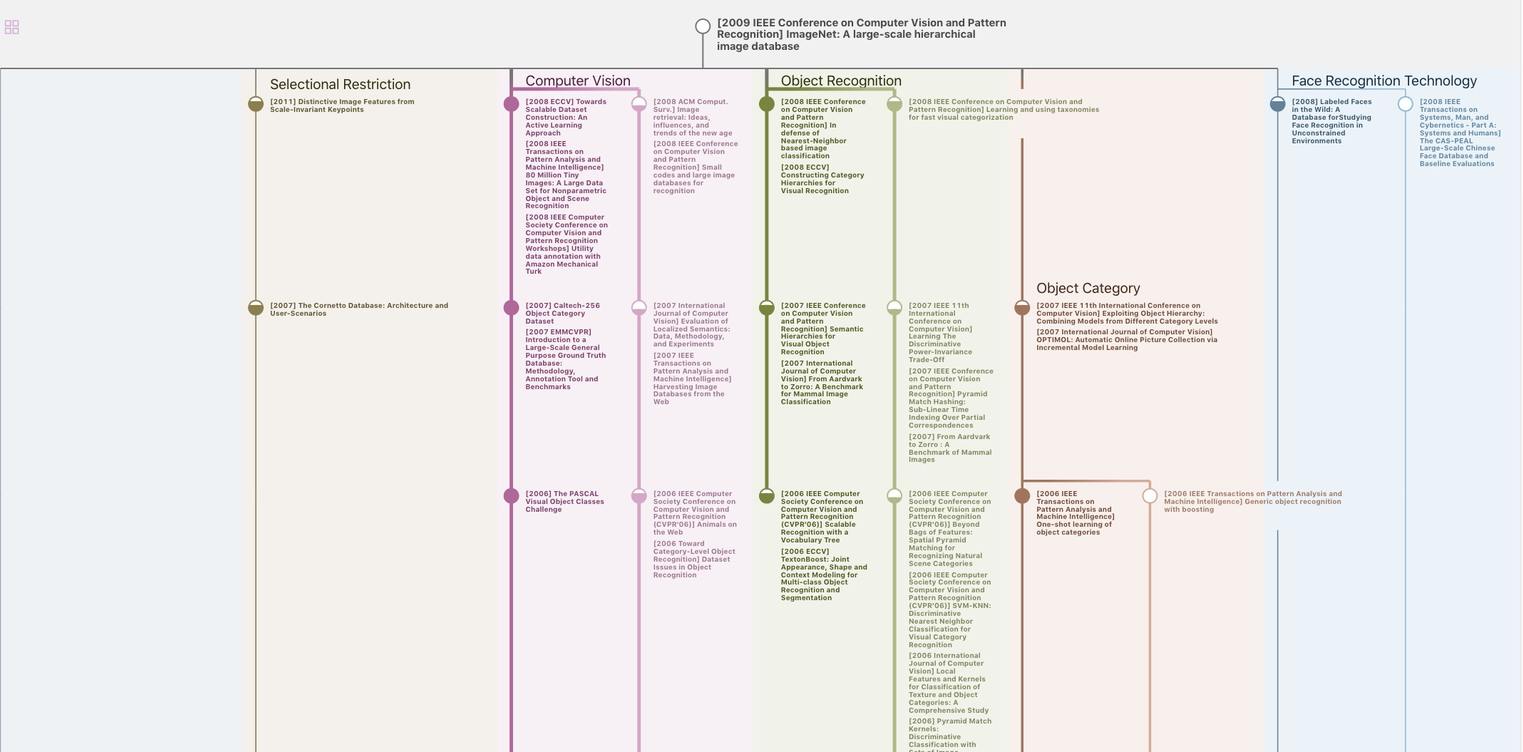
生成溯源树,研究论文发展脉络
Chat Paper
正在生成论文摘要