A machine learning-based framework to design capillary-driven networks
LAB ON A CHIP(2022)
摘要
We present a novel approach for the design of capillary-driven microfluidic networks using a machine learning genetic algorithm (ML-GA). This strategy relies on a user-friendly 1D numerical tool specifically developed to generate the necessary data to train the ML-GA. This 1D model was validated using analytical results issued from a Y-shaped capillary network and experimental data. For a given microfluidic network, we defined the objective of the ML-GA to obtain the set of geometric parameters that produces the closest matching results against two prescribed curves of delivered volume against time. We performed more than 20 generations of 10 000 simulations to train the ML-GA and achieved the optimal solution of the inverse design problem. The optimisation took less than 6 hours, and the results were successfully validated using experimental data. This work establishes the utility of the presented method for the fast and reliable design of complex capillary-driven devices, enabling users to optimise their designs via an easy-to-use 1D numerical tool and machine learning technique.
更多查看译文
关键词
networks,design,learning-based,capillary-driven
AI 理解论文
溯源树
样例
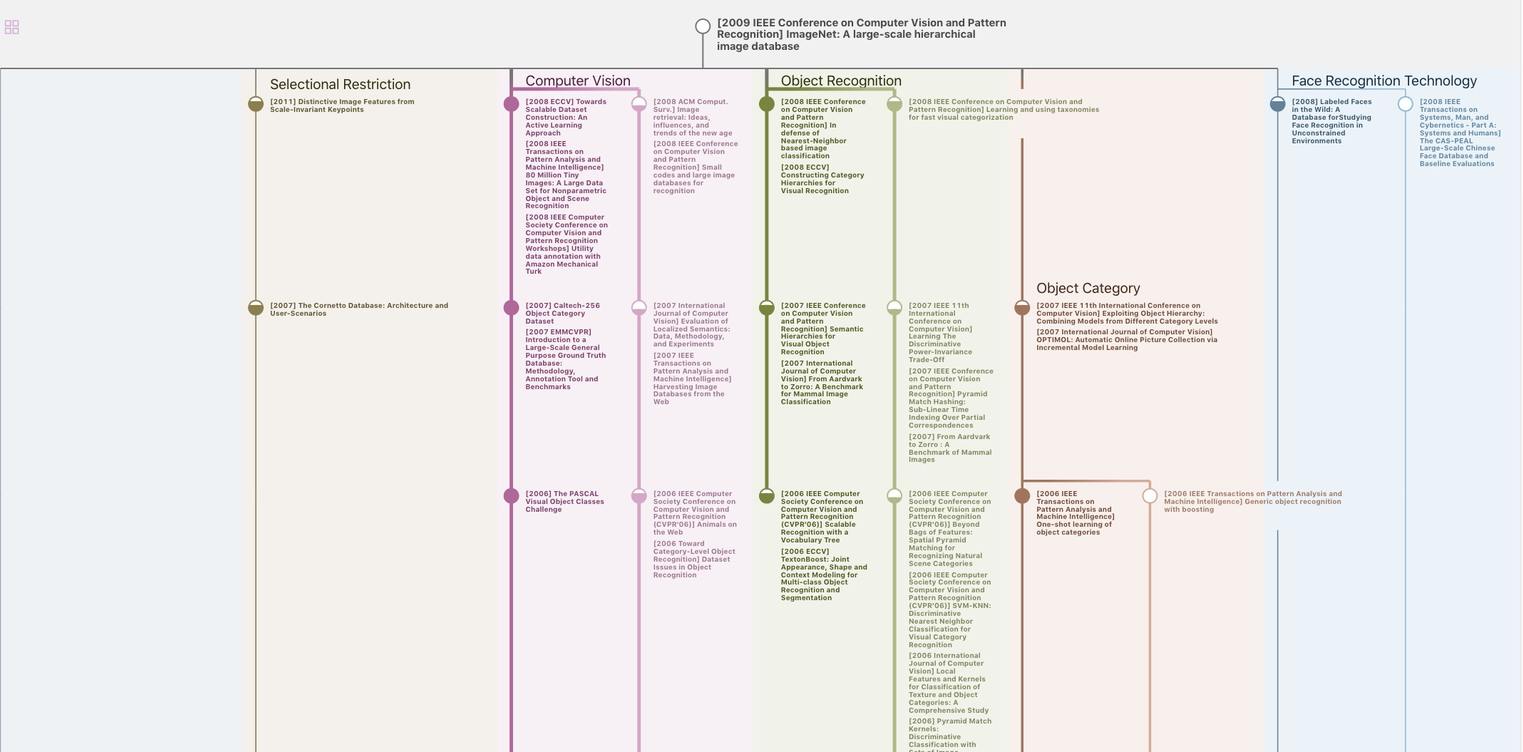
生成溯源树,研究论文发展脉络
Chat Paper
正在生成论文摘要