A machine learning approach to drawing phase diagrams of topological lasing modes
arxiv(2023)
摘要
Identifying phases and analyzing the stability of dynamic states are ubiquitous and important problems which appear in various physical systems. Nonetheless, drawing a phase diagram in high-dimensional and large parameter spaces has remained challenging. Here, we propose a data-driven method to derive the phase diagram of lasing modes in topological insulator lasers. The classification is based on the temporal behaviour of the topological modes obtained via numerical integration of the rate equation. A semi-supervised learning method is used and an adaptive library is constructed in order to distinguish the different topological modes present in the generated parameter space. The proposed method successfully distinguishes the different topological phases in the Su-Schrieffer-Heeger lattice with saturable gain. This demonstrates the possibility of classifying the topological phases without needing for expert knowledge of the system and may give valuable insight into the fundamental physics of topological insulator lasers via reverse engineering of the derived phase diagram.
更多查看译文
关键词
drawing phase diagrams,modes,machine learning
AI 理解论文
溯源树
样例
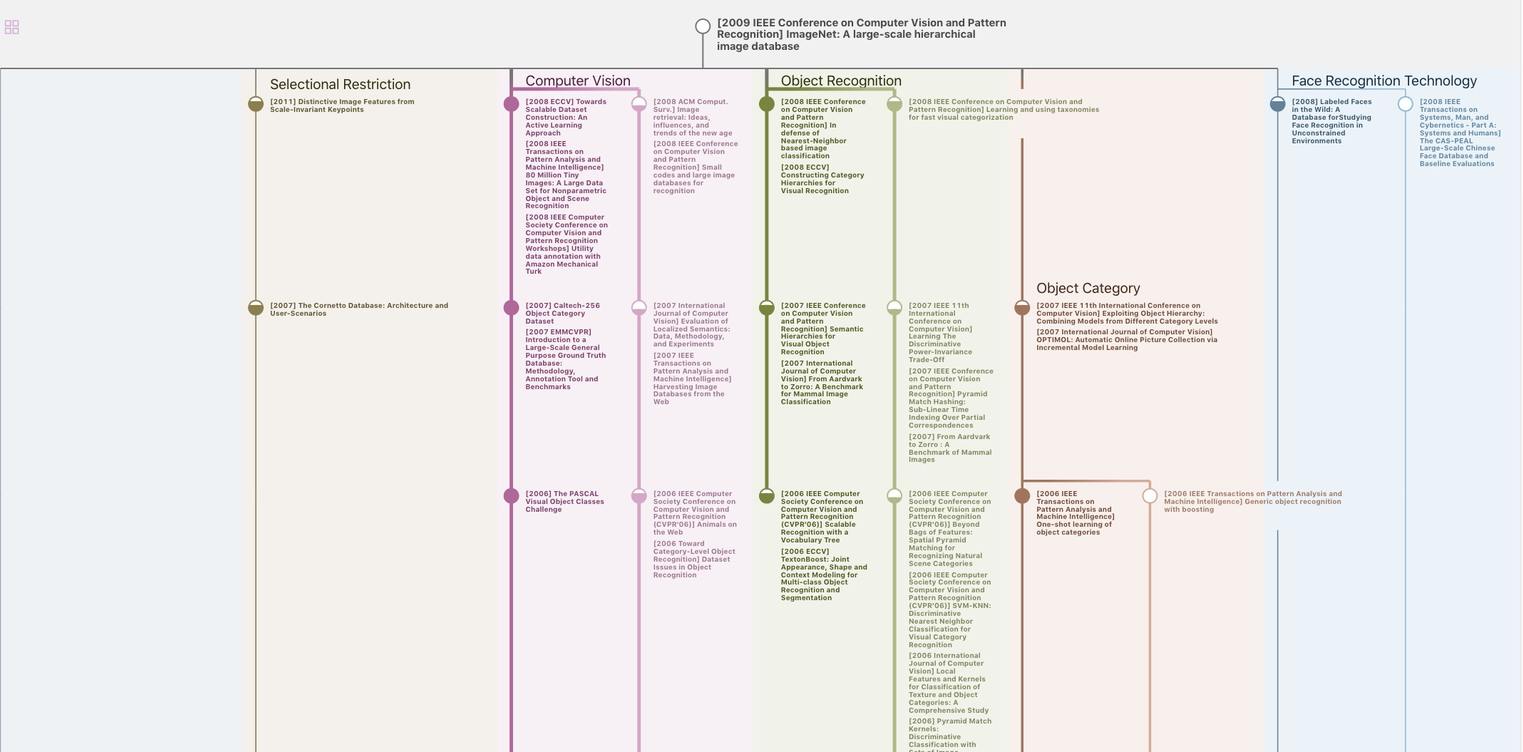
生成溯源树,研究论文发展脉络
Chat Paper
正在生成论文摘要