Scalable multi-class sampling via filtered sliced optimal transport
ACM TRANSACTIONS ON GRAPHICS(2022)
Abstract
We propose a multi-class point optimization formulation based on continuous Wasserstein barycenters. Our formulation is designed to handle hundreds to thousands of optimization objectives and comes with a practical optimization scheme. We demonstrate the effectiveness of our framework on various sampling applications like stippling, object placement, and Monte-Carlo integration. We a derive multi-class error bound for perceptual rendering error which can be minimized using our optimization. We provide source code at https://github.com/iribis/filtered-sliced-optimal-transport.
MoreTranslated text
Key words
Multi-class sampling,blue noise,optimal transport,Monte Carlo,rendering,perceptual error
AI Read Science
Must-Reading Tree
Example
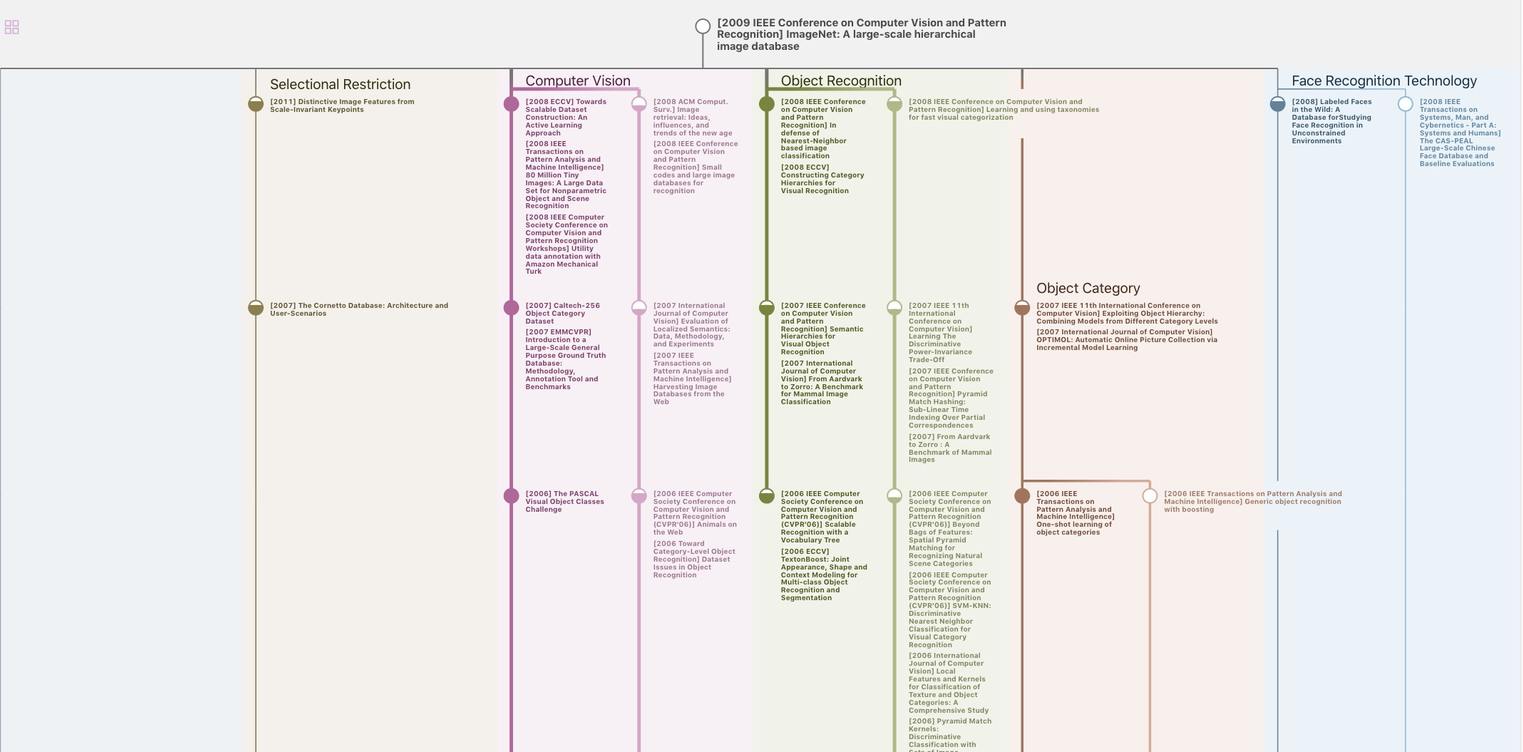
Generate MRT to find the research sequence of this paper
Chat Paper
Summary is being generated by the instructions you defined