MetaLoc: Learning to Learn Wireless Localization
IEEE JOURNAL ON SELECTED AREAS IN COMMUNICATIONS(2023)
摘要
Existing localization methods that intensively leverage the environment-specific received signal strength (RSS) or channel state information (CSI) of wireless signals are rather accurate in certain environments. However, these methods, whether based on pure statistical signal processing or data-driven approaches, often struggle to generalize to new environments, which results in considerable time and effort being wasted. To address this challenge, we propose MetaLoc, which is the first fingerprinting-based localization framework that leverages the Model-Agnostic Meta-Learning (MAML). Specifically, built on a deep neural network with strong representation capabilities, MetaLoc is trained on historical data sourced from well-calibrated environments, employing a two-loop optimization mechanism to obtain the meta-parameters. These meta-parameters act as the initialization for quick adaptation in new environments, reducing the need for much human effort. The framework introduces two paradigms for the optimization of meta-parameters: a centralized paradigm that simplifies the process by sharing data from all historical environments, and a distributed paradigm that maintains data privacy by training meta-parameters for each specific environment separately. Furthermore, the advanced distributed paradigm modifies the vanilla MAML loss function to ensure that the reduction of loss occurs in a consistent direction across various training domains, thus facilitating faster convergence during training. Our experiments on both synthetic and real datasets demonstrate that MetaLoc outperforms baseline methods in terms of localization accuracy, robustness, and cost-effectiveness. The code and datasets used in this study are publicly available at: https://github.com/WU-Dongze/MetaLoc.
更多查看译文
关键词
CSI,meta-learning,RSS,sample efficiency,wireless localization
AI 理解论文
溯源树
样例
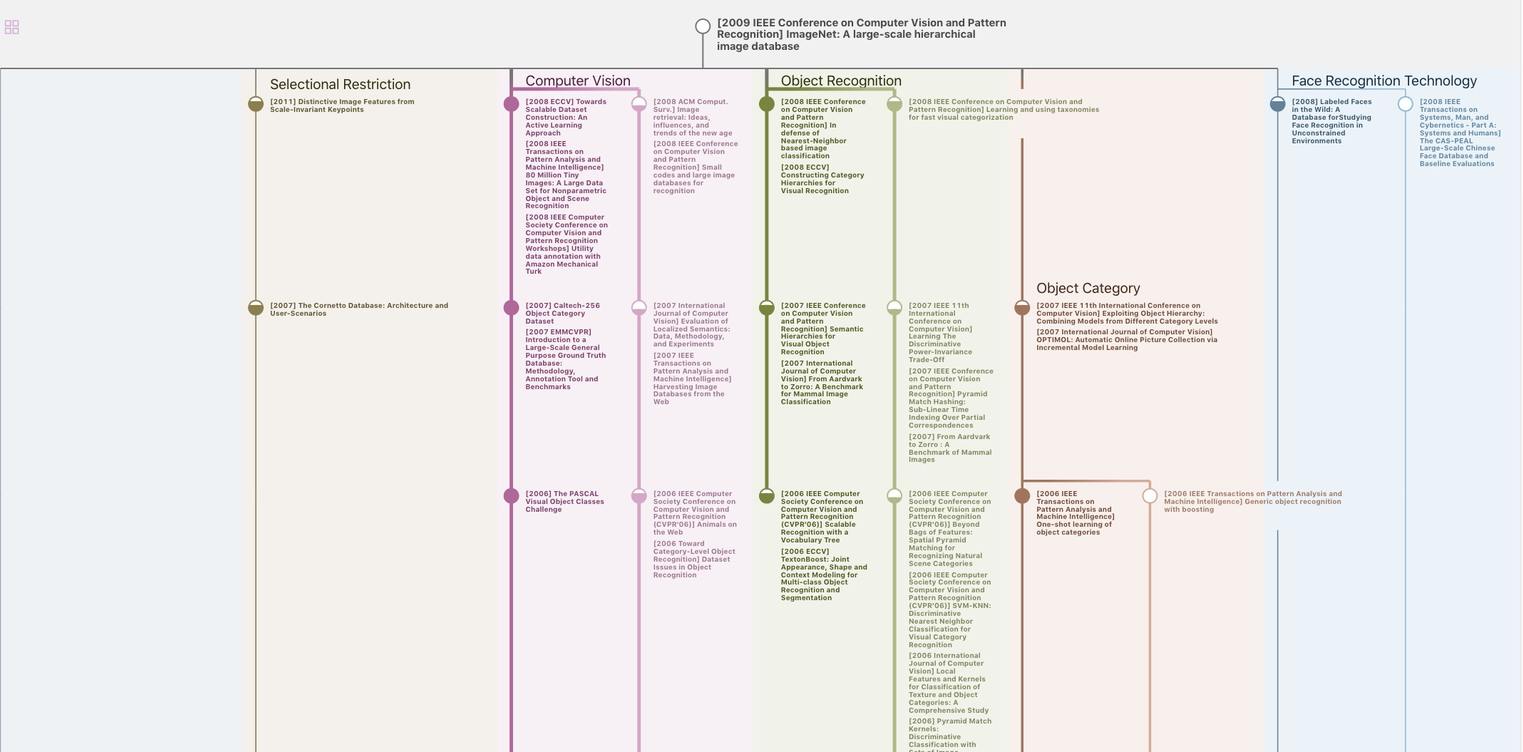
生成溯源树,研究论文发展脉络
Chat Paper
正在生成论文摘要