Federated learning based on stratified sampling and regularization
COMPLEX & INTELLIGENT SYSTEMS(2022)
摘要
Federated learning (FL) is a new distributed learning framework that is different from traditional distributed machine learning: (1) differences in communication, computing, and storage performance among devices (device heterogeneity), (2) differences in data distribution and data volume (data heterogeneity), and (3) high communication consumption. Under heterogeneous conditions, the data distribution of clients varies greatly, which leads to the problem that the convergence speed of the training model decreases and the training model cannot converge to the global optimal solution. In this work, an FL algorithm based on stratified sampling and regularization (FedSSAR) is proposed. In FedSSAR, a density-based clustering method is used to divide the overall client into different clusters, then, some available clients are proportionally extracted from different clusters to participate in training which realizes unbiased sampling for the overall client and reduces the aggregation weight variance of the client. At the same time, when calculating the model local loss function, we limit the update direction of the model by a regular term, so that heterogeneous clients are optimized in the globally optimal direction. We prove the convergence of FedSSAR theoretically and experimentally, and demonstrate the superiority of FedSSAR by comparing it with other FL algorithms on public datasets.
更多查看译文
关键词
Federated learning,Clustering,Stratified sampling,Distributed optimization,Convergence analysis,Regularization
AI 理解论文
溯源树
样例
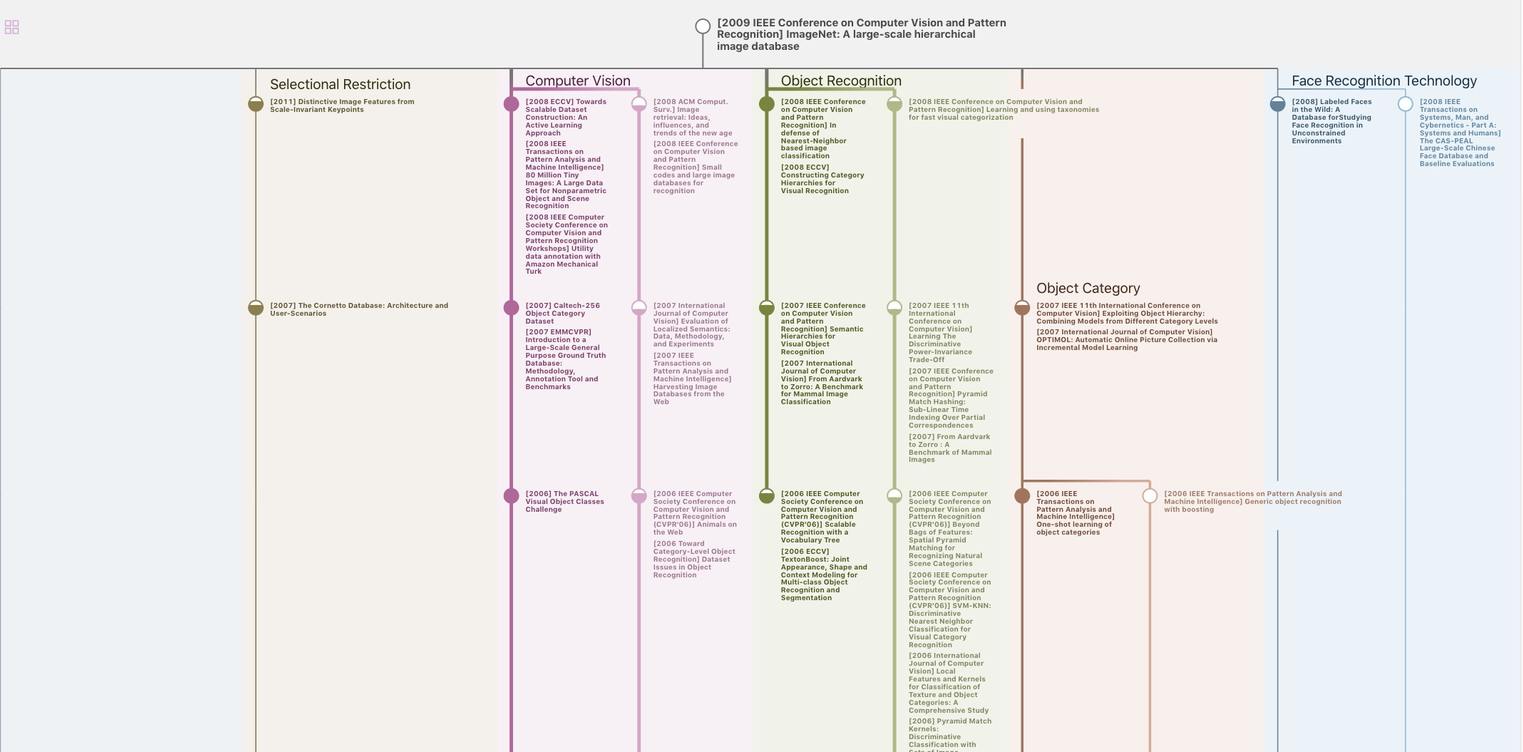
生成溯源树,研究论文发展脉络
Chat Paper
正在生成论文摘要