Comparison of CLDAS and Machine Learning Models for Reference Evapotranspiration Estimation under Limited Meteorological Data
SUSTAINABILITY(2022)
摘要
The accurate calculation of reference evapotranspiration (ET0) is the fundamental basis for the sustainable use of water resources and drought assessment. In this study, we evaluate the performance of the second-generation China Meteorological Administration Land Data Assimilation System (CLDAS) and two simplified machine learning models to estimate ET0 when meteorological data are insufficient in China. The results show that, when a weather station lacks global solar radiation (R-s) data, the machine learning methods obtain better results in their estimation of ET0. However, when the meteorological station lacks relative humidity (RH) and 2 m wind speed (U-2) data, using RHCLD and U-2CLD from the CLDAS to estimate ET0 and to replace the meteorological station data obtains better results. When all the data from the meteorological station are missing, estimating ET0 using the CLDAS data still produces relevant results. In addition, the PM-CLDAS method (a calculation method based on the Penman-Monteith formula and using the CLDAS data) exhibits a relatively stable performance under different combinations of meteorological inputs, except in the southern humid tropical zone and the Qinghai-Tibet Plateau zone.
更多查看译文
关键词
reference evapotranspiration,reanalysis dataset,CLDAS,machine learning,limited meteorological data
AI 理解论文
溯源树
样例
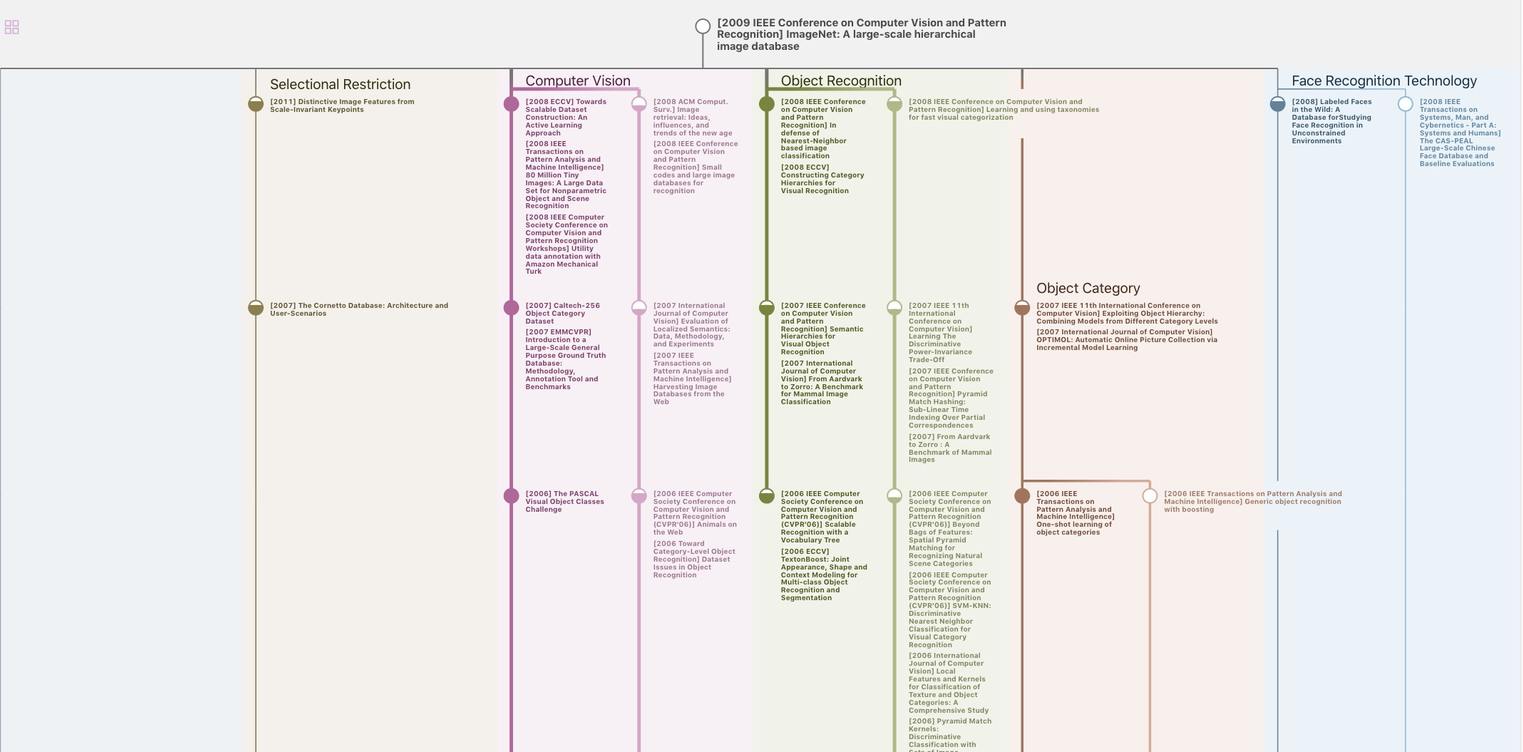
生成溯源树,研究论文发展脉络
Chat Paper
正在生成论文摘要