A discriminatively deep fusion approach with improved conditional GAN (im-cGAN) for facial expression recognition
PATTERN RECOGNITION(2023)
Abstract
Considering most deep learning-based methods heavily depend on huge labels, it is still a challenging issue for facial expression recognition to extract discriminative features of training samples with limited labels. Given above, we propose a discriminatively deep fusion (DDF) approach based on an improved conditional generative adversarial network (im-cGAN) to learn abstract representation of facial expres-sions. First, we employ facial images with action units (AUs) to train the im-cGAN to generate more labeled expression samples. Subsequently, we utilize global features learned by the global-based module and the local features learned by the region-based module to obtain the fused feature representation. Finally, we design the discriminative loss function (D-loss) that expands the inter-class variations while minimizing the intra-class distances to enhance the discrimination of fused features. Experimental results on JAFFE, CK + , Oulu-CASIA, and KDEF datasets demonstrate the proposed approach is superior to some state-of-the-art methods.(c) 2022 Elsevier Ltd. All rights reserved.
MoreTranslated text
Key words
Facial expression recognition,Discriminatively deep fusion approach,Improved conditional generative adversarial network,Discriminative loss function
AI Read Science
Must-Reading Tree
Example
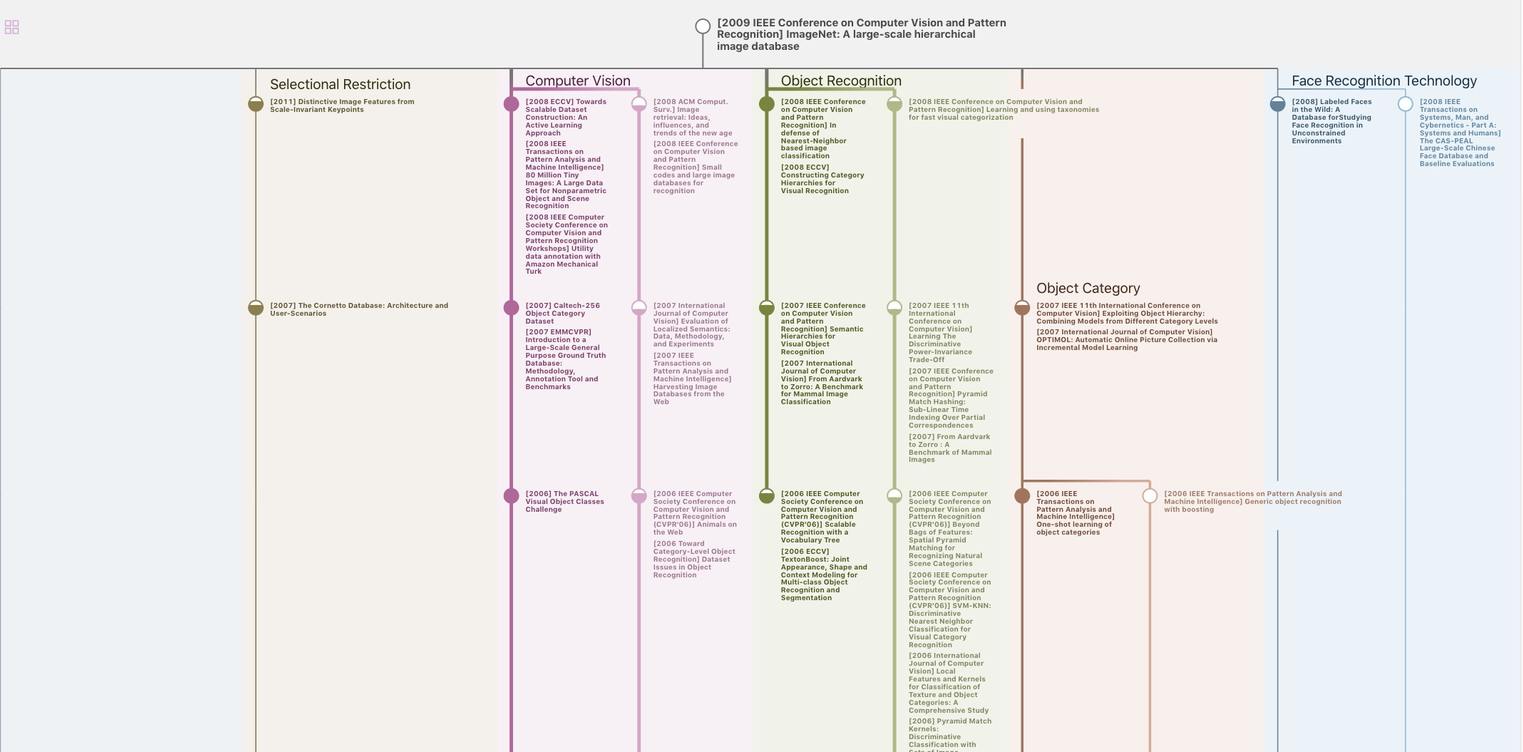
Generate MRT to find the research sequence of this paper
Chat Paper
Summary is being generated by the instructions you defined