Hyperspectral Image Classification Based on Multi-Scale Feature Fusion Residual Network
Laser & Optoelectronics Progress(2022)
摘要
In order to fully extract the spectral-spatial features of hyperspectral image (HSI) and to achieve high-precision ground object classification of HSI, an end-to-end multi-scale feature fusion identity (MFFI) block is proposed. This block combines 3D multi-scale convolution, feature fusion and residual connection. Through this block, multi-scale spectral-spatial joint features of HSI can be extracted. Because of the end-to-end feature of the block, the final MFFI network can be obtained by stacking multiple MFFI blocks. The average overall accuracy of 99.73%, average accuracy of 99.84%, and Kappa coefficient of 0.9971 are obtained on three HSI datasets: Salinas, Indian Pines and University of Pavia. The results show that the proposed MFFI block can effectively extract the spectral-spatial features of different types of ground object datasets and achieve satisfactory classification results.
更多查看译文
关键词
hyperspectral image classification,residual structure,multi-scale feature fusion,spectral-spatial feature extraction,convolutional neural network
AI 理解论文
溯源树
样例
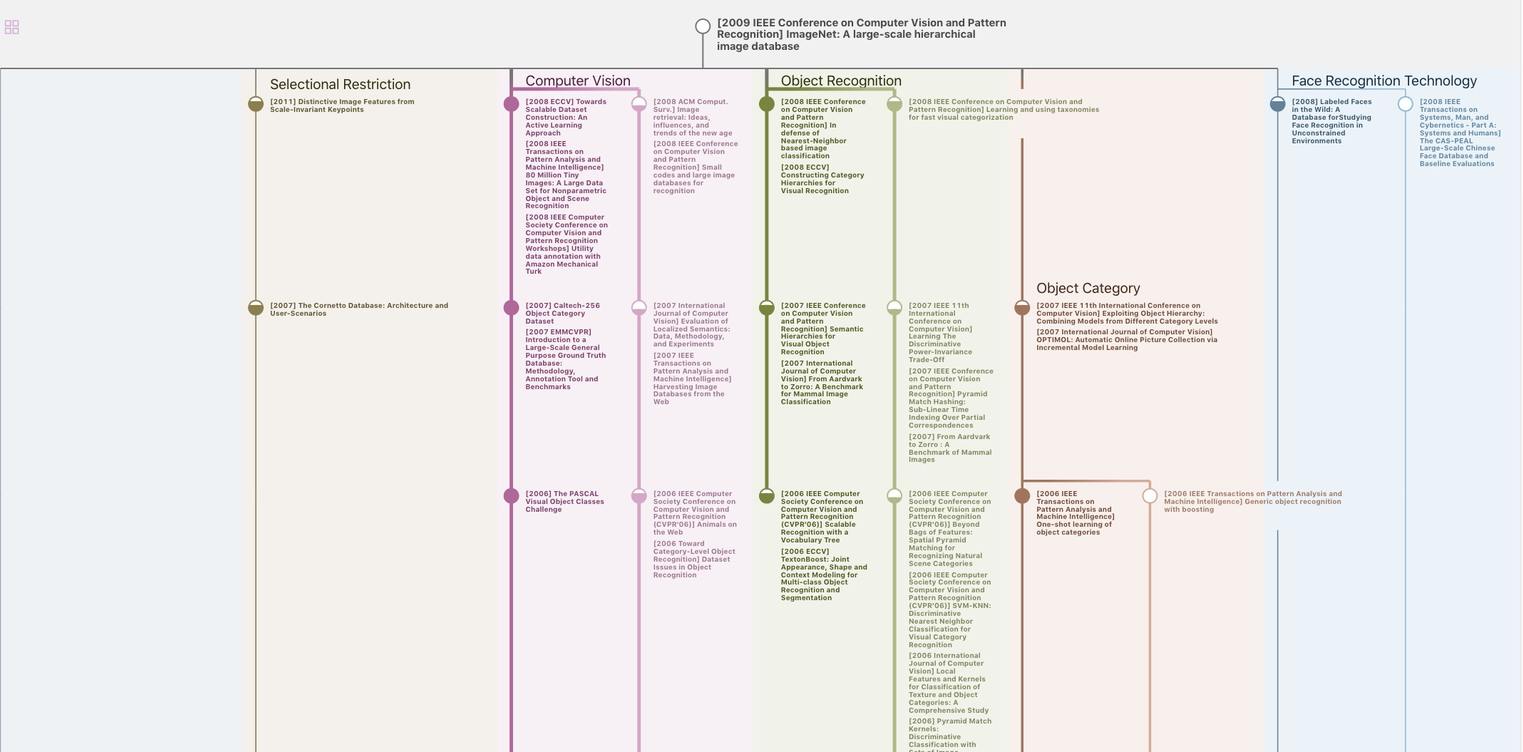
生成溯源树,研究论文发展脉络
Chat Paper
正在生成论文摘要