High-Resolution Boundary Detection for Medical Image Segmentation with Piece-Wise Two-Sample T-Test Augmented Loss
arxiv(2022)
摘要
Deep learning methods have contributed substantially to the rapid advancement of medical image segmentation, the quality of which relies on the suitable design of loss functions. Popular loss functions, including the cross-entropy and dice losses, often fall short of boundary detection, thereby limiting high-resolution downstream applications such as automated diagnoses and procedures. We developed a novel loss function that is tailored to reflect the boundary information to enhance the boundary detection. As the contrast between segmentation and background regions along the classification boundary naturally induces heterogeneity over the pixels, we propose the piece-wise two-sample t-test augmented (PTA) loss that is infused with the statistical test for such heterogeneity. We demonstrate the improved boundary detection power of the PTA loss compared to benchmark losses without a t-test component.
更多查看译文
关键词
medical image segmentation,image segmentation,high-resolution,piece-wise,two-sample,t-test
AI 理解论文
溯源树
样例
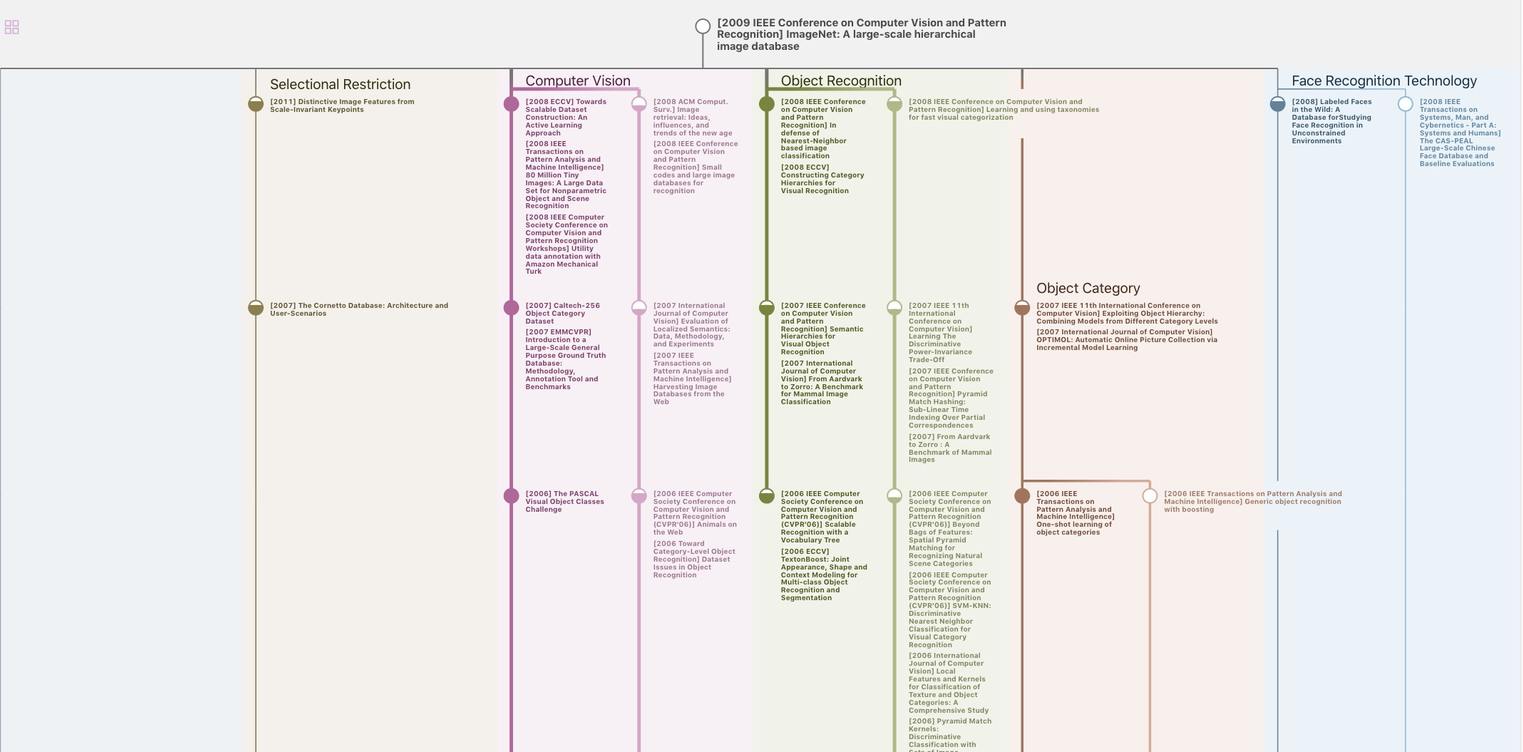
生成溯源树,研究论文发展脉络
Chat Paper
正在生成论文摘要