Exploiting exotic LHC datasets for long-lived new particle searches
arxiv(2022)
摘要
bstract Motivated by the expectation that new physics may manifest itself in the form of very heavy new particles, most of the operation time of the Large Hadron Collider (LHC) is devoted to proton-proton ( pp ) collisions at the highest achievable energies and collision rates. The large collision rates imply tight trigger requirements that include high thresholds on the final-state particles’ transverse momenta p T and an intrinsic background in the form of particle pileup produced by different collisions occurring during the same bunch crossing. This strategy is potentially sub-optimal for several well-motivated new physics models where new particles are not particularly heavy and can escape the online selection criteria of the multi-purpose LHC experiments due to their light mass and small coupling. A solution may be offered by complementary datasets that are routinely collected by the LHC experiments. These include heavy ion collisions, low-pileup runs for precision physics, and the so-called “parking” and “scouting” datasets. While some of them are motivated by other physics goals, they all have the usage of mild p T thresholds at the trigger-level in common. In this study, we assess the relative merits of these datasets for a representative model whose particular clean signature features long-lived resonances yielding displaced dimuon vertices. We compare the reach across those datasets for a simple analysis, simulating LHC data in Run 2 and Run 3 conditions with the Delphes simulation. We show that the scouting and parking datasets, which afford low- p T trigger thresholds by only using partial detector information and delaying the event reconstruction, respectively, have a reach comparable to the standard pp dataset with conventional thresholds. We also show that heavy ion and low-pileup datasets are far less competitive for this signature.
更多查看译文
关键词
New Light Particles,Specific BSM Phenomenology,Axions and ALPs
AI 理解论文
溯源树
样例
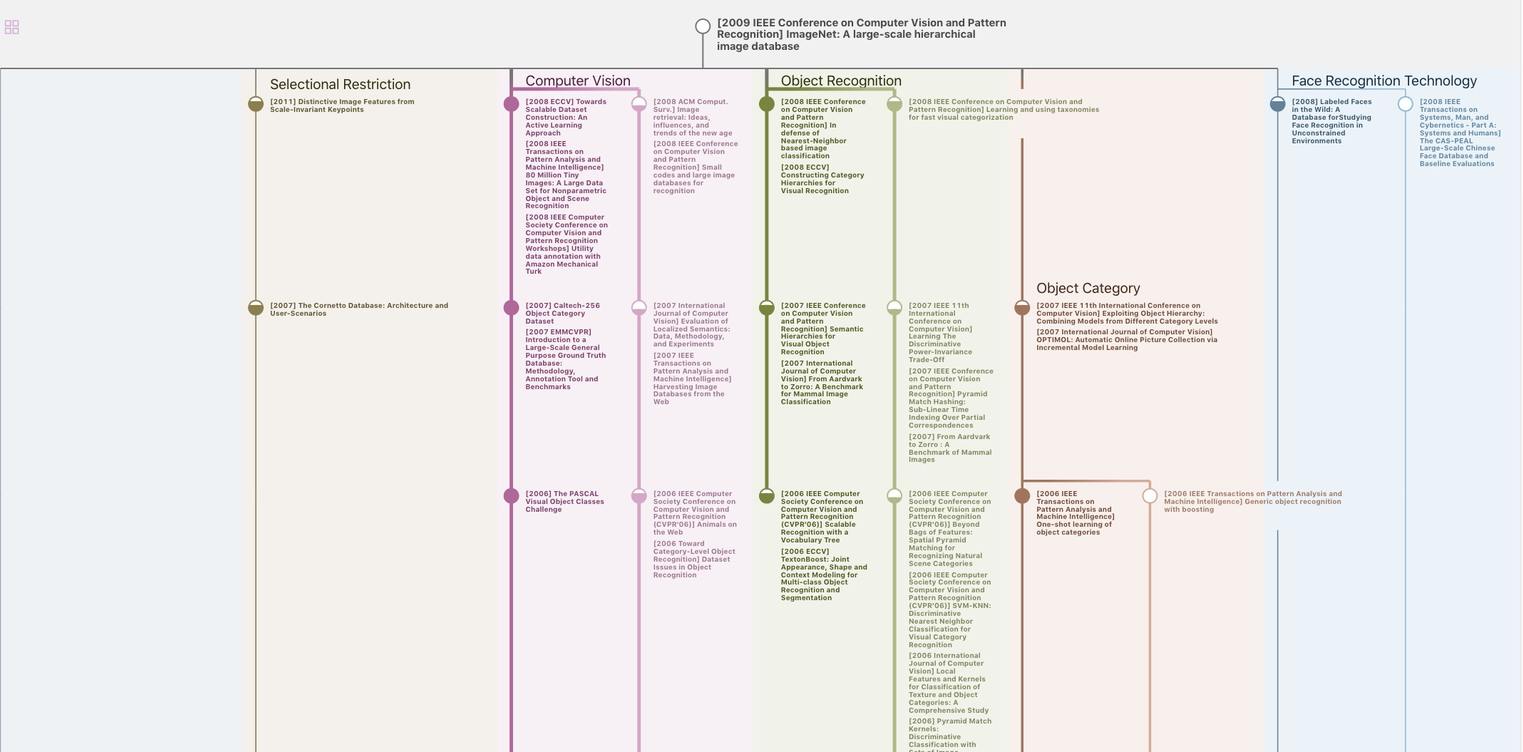
生成溯源树,研究论文发展脉络
Chat Paper
正在生成论文摘要