Can Querying for Bias Leak Protected Attributes? Achieving Privacy With Smooth Sensitivity
PROCEEDINGS OF THE 6TH ACM CONFERENCE ON FAIRNESS, ACCOUNTABILITY, AND TRANSPARENCY, FACCT 2023(2022)
Abstract
Existing regulations prohibit model developers from accessing protected attributes (gender, race, etc.), often resulting in fairness assessments on populations without knowing their protected groups. In such scenarios, institutions often adopt a separation between the model developers (who train models with no access to the protected attributes) and a compliance team (who may have access to the entire dataset for auditing purpose). However, the model developers might be allowed to test their models for bias by querying the compliance team for group fairness metrics. In this paper, we first demonstrate that simply querying for fairness metrics, such as statistical parity and equalized odds can leak the protected attributes of individuals to the model developers. We demonstrate that there always exist strategies by which the model developers can identify the protected attribute of a targeted individual in the test dataset from just a single query. In particular, we show that one can reconstruct the protected attributes of all the individuals from O(Nk log n/Nk) queries when Nk<More
Translated text
Key words
bias leak protected attributes,privacy,querying
AI Read Science
Must-Reading Tree
Example
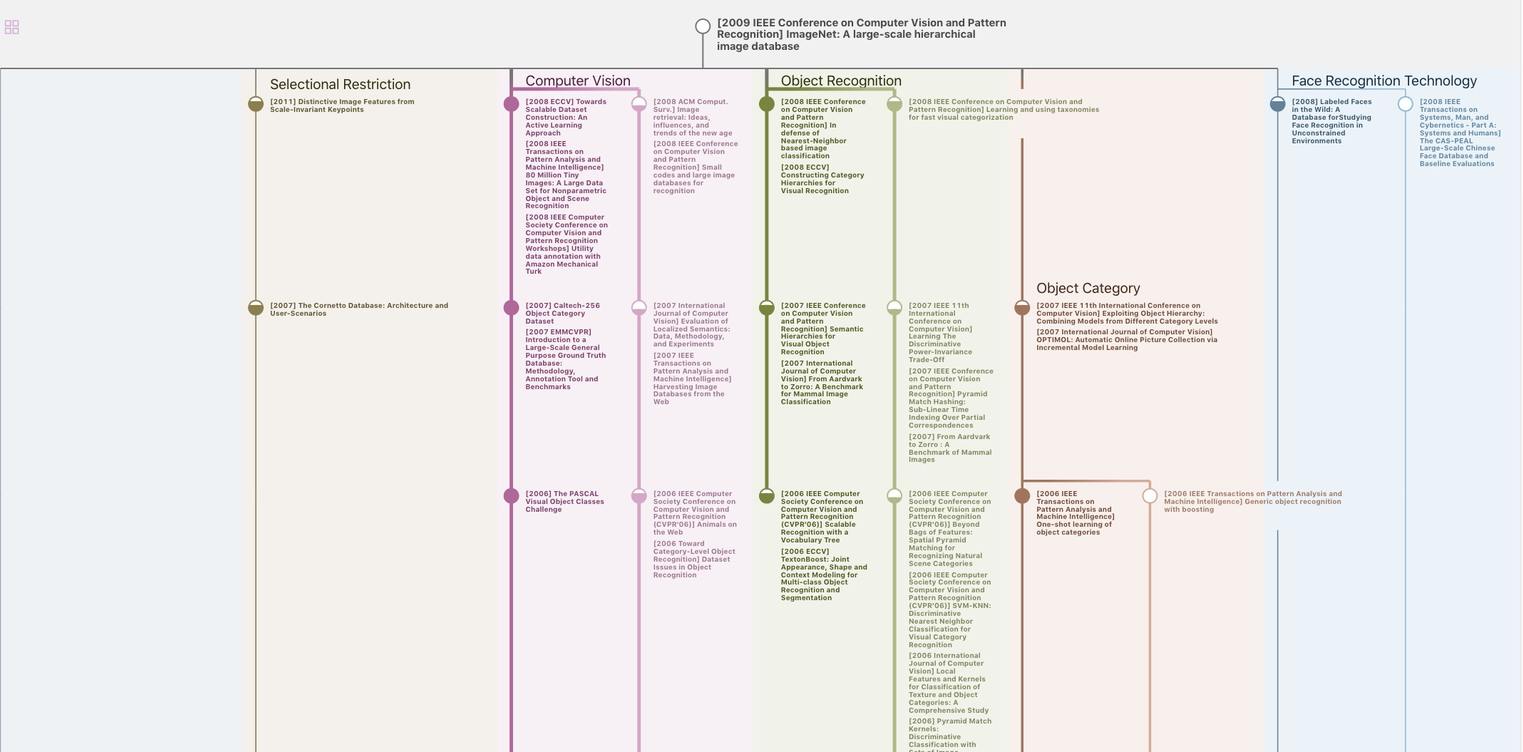
Generate MRT to find the research sequence of this paper
Chat Paper
Summary is being generated by the instructions you defined